Developing a Machine Learning Pipeline for Predicting Neurological Outcomes in Comatose Cardiac Arrest Survivors Using Continuous EEG Data.
2023 Computing in Cardiology (CinC)(2023)
Abstract
As part of the ‘Predicting Neurological Recovery from Como After Cardiac Arrest: The George B. Moody PhysioNet Challenge 2023’, we propose a two-step approach, which comprises i) a custom convolutional neural network designed to classify Cerebral Performance Categories (CPC) based on 30-second EEG signals from 19 channels, and ii) random forest models that combine the logits obtained in the previous step with the patient metadata to predict the CPC values and the patient outcome. The final predictions for patient CPC and outcome are determined through a time-weighted average of EEG window prediction. Our submission achieved challenge metric scores of 0.351, 0.386, 0.431, and 0.475 for the prediction outcome at 12h, 24h, 48h, and 72h, respectively. These scores placed the AIMED team in the 20th position in the rank of the official phase. Our study highlights the potential of a machine learning pipeline to predict neurological outcomes in comatose cardiac arrest survivors. This is achieved through a straightforward methodology that combines features extracted from EEG signals with patient metadata.
MoreTranslated text
AI Read Science
Must-Reading Tree
Example
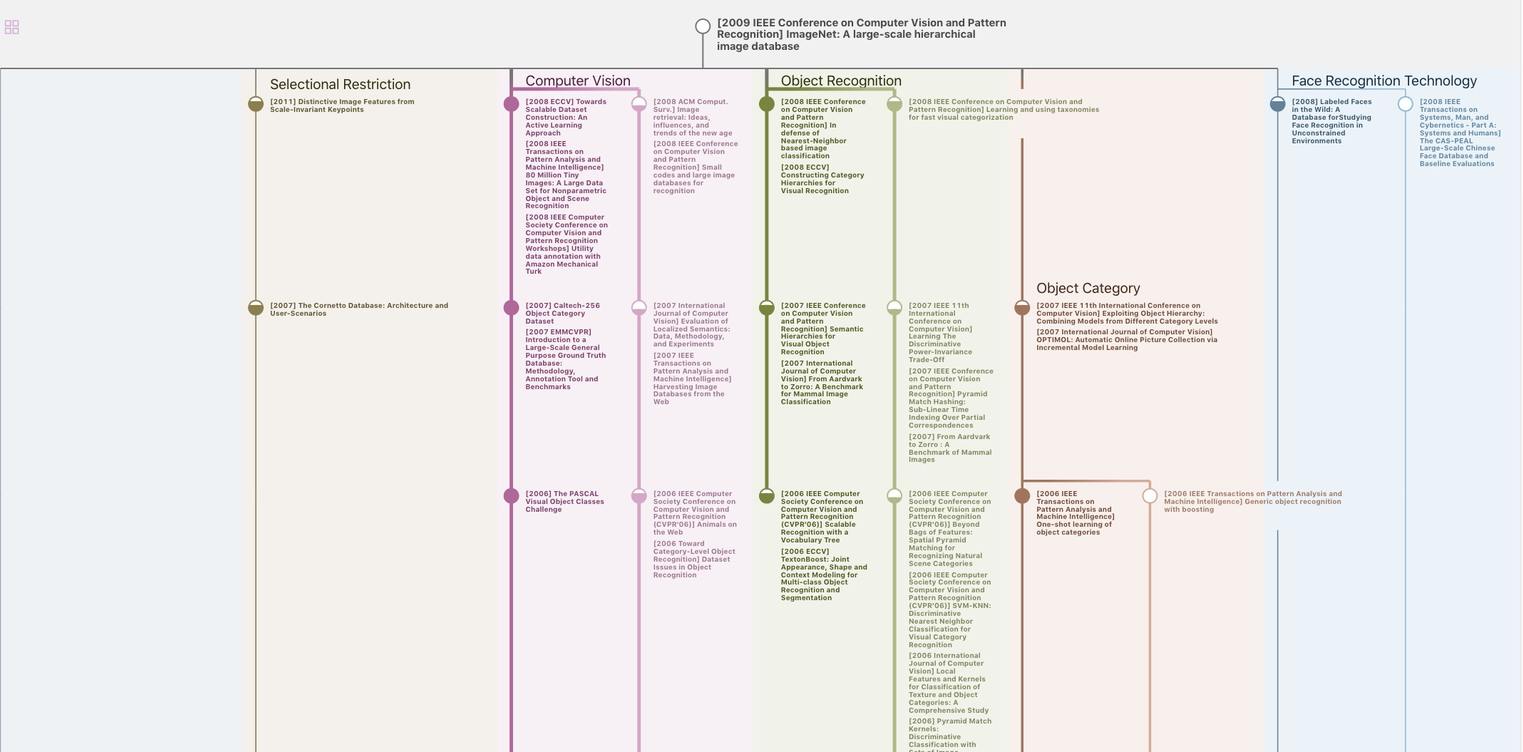
Generate MRT to find the research sequence of this paper
Chat Paper
Summary is being generated by the instructions you defined