Exploring Inlier and Outlier Specification for Improved Medical OOD Detection
2023 IEEE/CVF INTERNATIONAL CONFERENCE ON COMPUTER VISION WORKSHOPS, ICCVW(2023)
Abstract
We address the crucial task of developing well-calibrated out-of-distribution (OOD) detectors, in order to enable safe deployment of medical image classifiers. Calibration enables deep networks to protect against trivial decision rules and controls over-generalization, thereby supporting model reliability. Given the challenges involved in curating appropriate calibration datasets, synthetic augmentations have gained significant popularity for inlier/outlier specification. Despite the rapid progress in data augmentation techniques, our study reveals a remarkable finding: the synthesis space and augmentation type play a pivotal role in effectively calibrating OOD detectors. Using the popular energy-based OOD detection framework, we find that the optimal protocol is to synthesize latent-space inliers along with diverse pixel-space outliers. Through extensive empirical studies conducted on multiple medical imaging benchmarks, we consistently demonstrate the superiority of our approach, achieving substantial improvements of 15% - 35% in AU-ROC compared to the state-of-the-art across various openset recognition settings.
MoreTranslated text
AI Read Science
Must-Reading Tree
Example
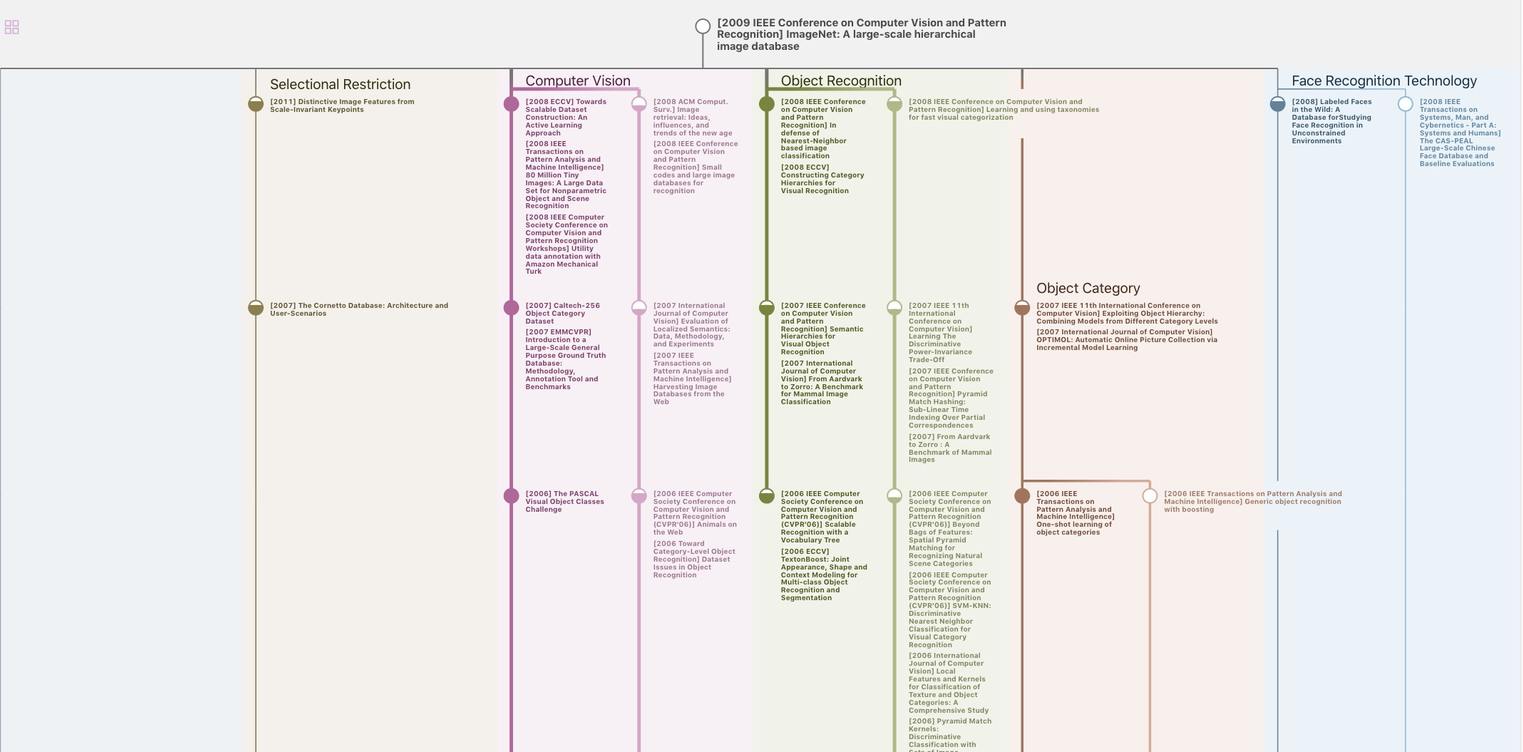
Generate MRT to find the research sequence of this paper
Chat Paper
Summary is being generated by the instructions you defined