Cross-grained Contrastive Representation for Unsupervised Lesion Segmentation in Medical Images
2023 IEEE/CVF INTERNATIONAL CONFERENCE ON COMPUTER VISION WORKSHOPS, ICCVW(2023)
摘要
Automatic segmentation of lesions in medical images plays a crucial role in the quantitative assessment of disease progression. While supervised deep learning-based methods have been successful in numerous segmentation tasks, they rely on a large number of labeled images for training, which can be expensive and time-consuming to acquire. Although unsupervised learning shows potential in addressing this challenge, the performance of current unsupervised algorithms is mostly unsatisfactory. To overcome this issue, we propose a new unsupervised framework for medical lesion segmentation using a novel cross-granularity contrastive (CGC) module. Our module contains coarse-grained and fine-grained discrimination paths that enable the network to capture the distinctions between lesions and normal tissues at different levels of context. We evaluate our method on two large public datasets of CT/MRI scans and demonstrate that our approach improves a Gaussian mixture model-based segmentation by up to 9%, which surpasses all other unsupervised segmentation methods by a large margin. Additionally, our module can also be integrated with other existing unsupervised segmentation methods to further enhance their performance. Therefore, our framework shows great potential for use in medical image applications with limited labeled data availability. The code of this work will be released via https://github.com/yu02019.
更多查看译文
AI 理解论文
溯源树
样例
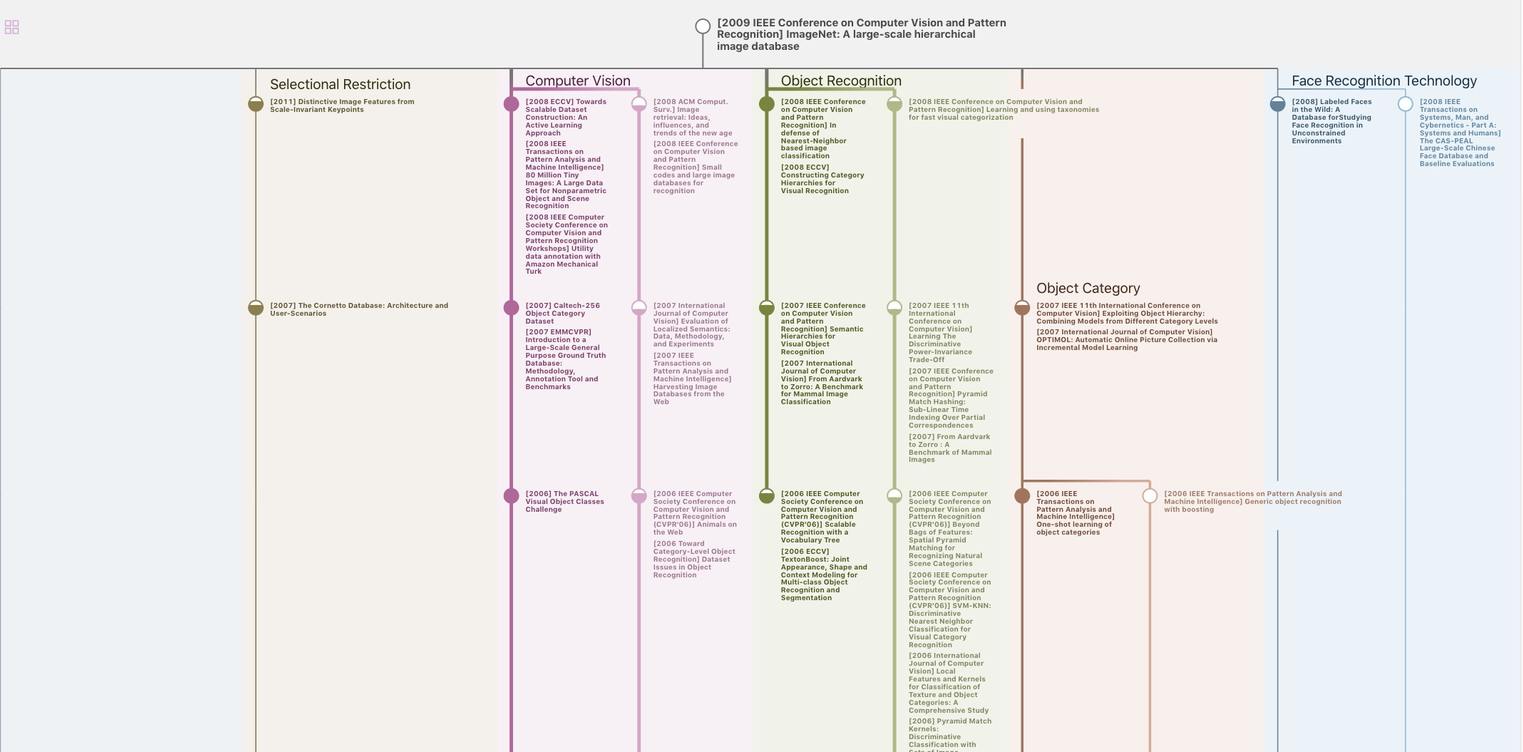
生成溯源树,研究论文发展脉络
Chat Paper
正在生成论文摘要