Synthetic Seismocardiography Signal Generation by a Generative Adversarial Network.
2023 Computing in Cardiology (CinC)(2023)
摘要
Aims: Seismocardiography (SCG) signals provide valuable information about the heart's performance. The technique subsists in a noisy environment where deep learning is often needed to extract important information, requiring large amounts of training data which can be expensive to obtain. In this work, we aim to create synthetic SCG heartbeats that are realistic and diverse to affordably augment current SCG datasets. Methods: We trained a Generative Adversarial Network (GAN) on real SCG heartbeats to produce synthetic SCG data. The architecture consisted of a deep convolutional GAN that was conditioned on an embedded identifier label for each subject to enable the generation of subject-specific heartbeats. Results: Our results demonstrated that the GAN could generate SCG heartbeats that closely resembled real SCG morphology. Generated heartbeats had an average root-mean-squared-error of 0.1831 when compared to the ensemble average of their real counterparts. Conclusion: The study presented a novel approach of using GANs to generate artificial SCG heartbeats. The use of GAN-generated SCG heartbeats has the potential to overcome the limitations of real SCG data availability, allowing for enhanced research and clinical applications of this valuable cardiac diagnostic technique.
更多查看译文
AI 理解论文
溯源树
样例
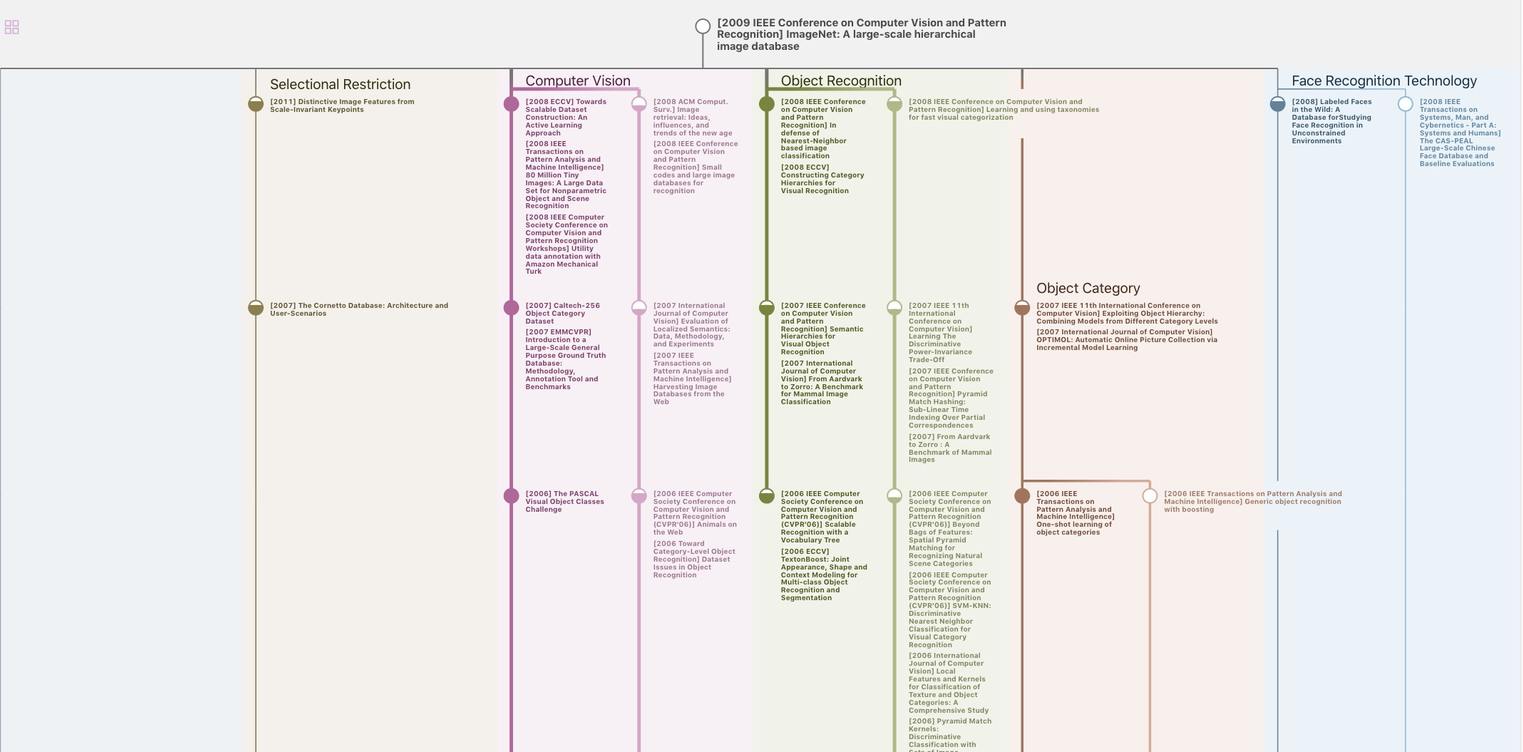
生成溯源树,研究论文发展脉络
Chat Paper
正在生成论文摘要