Margin Contrastive Learning with Learnable-Vector for Continual Learning
2023 IEEE/CVF INTERNATIONAL CONFERENCE ON COMPUTER VISION WORKSHOPS, ICCVW(2023)
摘要
In continual learning, there is a serious problem "catastrophic forgetting", in which previously acquired knowledge is forgotten when a new task is learned. Various methods have been proposed to solve this problem. Among them, Replay methods, which store a portion of the past training data and regenerate it for later tasks, have shown excellent performance. In this paper, we propose a new online continuous learning method that adds a representative vector for each class and a margin for similarity computation to the conventional method, Supervised Contrastive Replay (SCR). Our method aims to mitigate the catastrophic forgetting caused by class imbalance by using learnable vectors of each class and adding a margin to the calculation of similarity. Experiments on multiple image classification datasets confirm that our method outperformed conventional methods.
更多查看译文
AI 理解论文
溯源树
样例
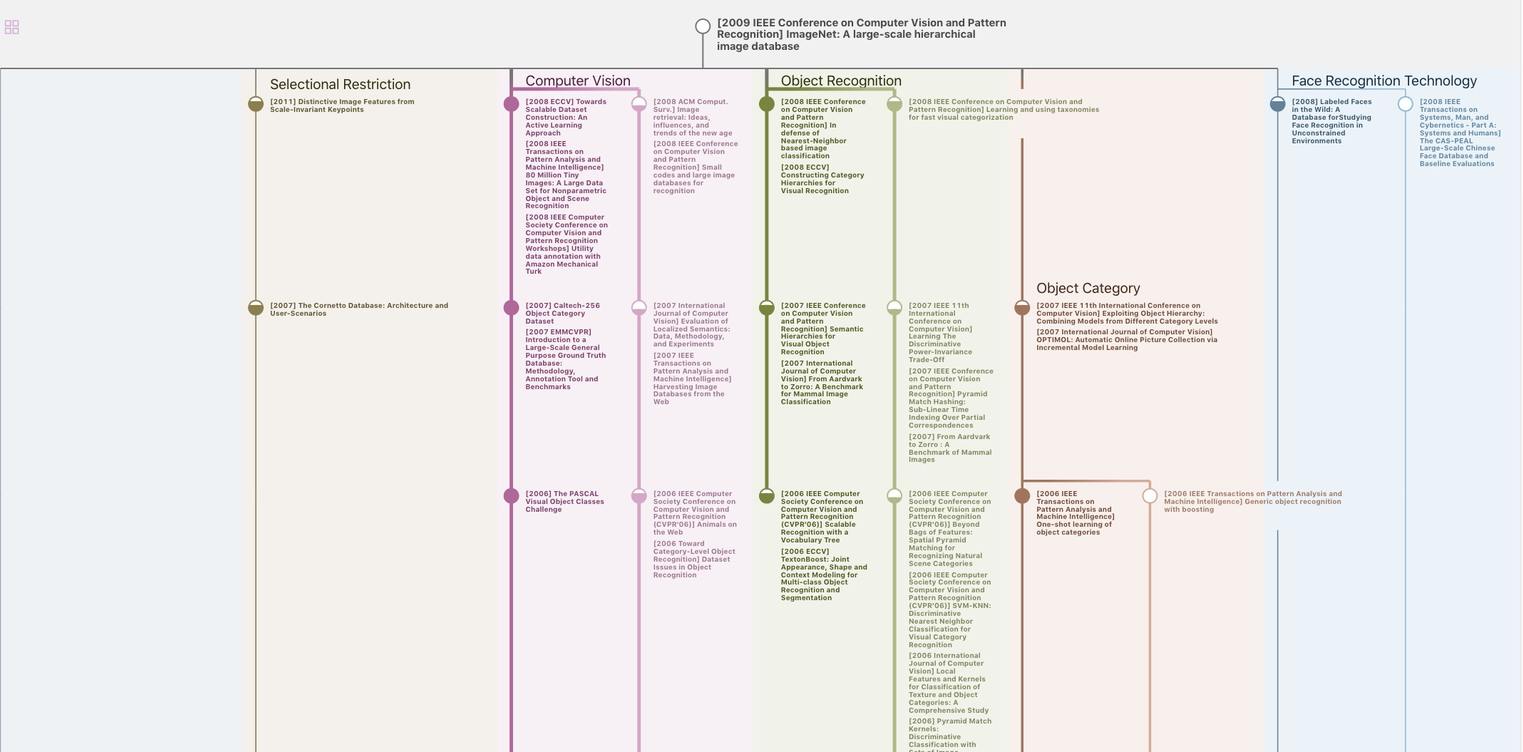
生成溯源树,研究论文发展脉络
Chat Paper
正在生成论文摘要