Predicting Recovery from Coma After Cardiac Arrest Using Low-level Features from EEG Recordings and a Small-sized LSTM Network.
2023 Computing in Cardiology (CinC)(2023)
摘要
This paper presents a computational approach proposed as part of the George B. Moody PhysioNet Challenge 2023. This method uses electroencephalograms (EEGs) to predict the neurological recovery of patients following cardiac arrest and was proposed by the team Oldenburg. It relies on computing a set of low-level time-varying features from a small subset of the available EEG channels. The resulting sequence of features are input to a recurrent neural network based on long short-term memory (LSTM) cells that estimates the probability of the target patient to be in a given cerebral performance category (CPC) status. The resulting model received a Challenge score of 0.025 (ranked 36th out of 36 submissions) on the hidden test set, failing to produce satisfying detection with a false positive rate inferior to 0.05, as required by the Challenge score. However, results on the validation set suggest that the proposed method could yield useful results, though more thorough tuning and evaluation would be needed.
更多查看译文
AI 理解论文
溯源树
样例
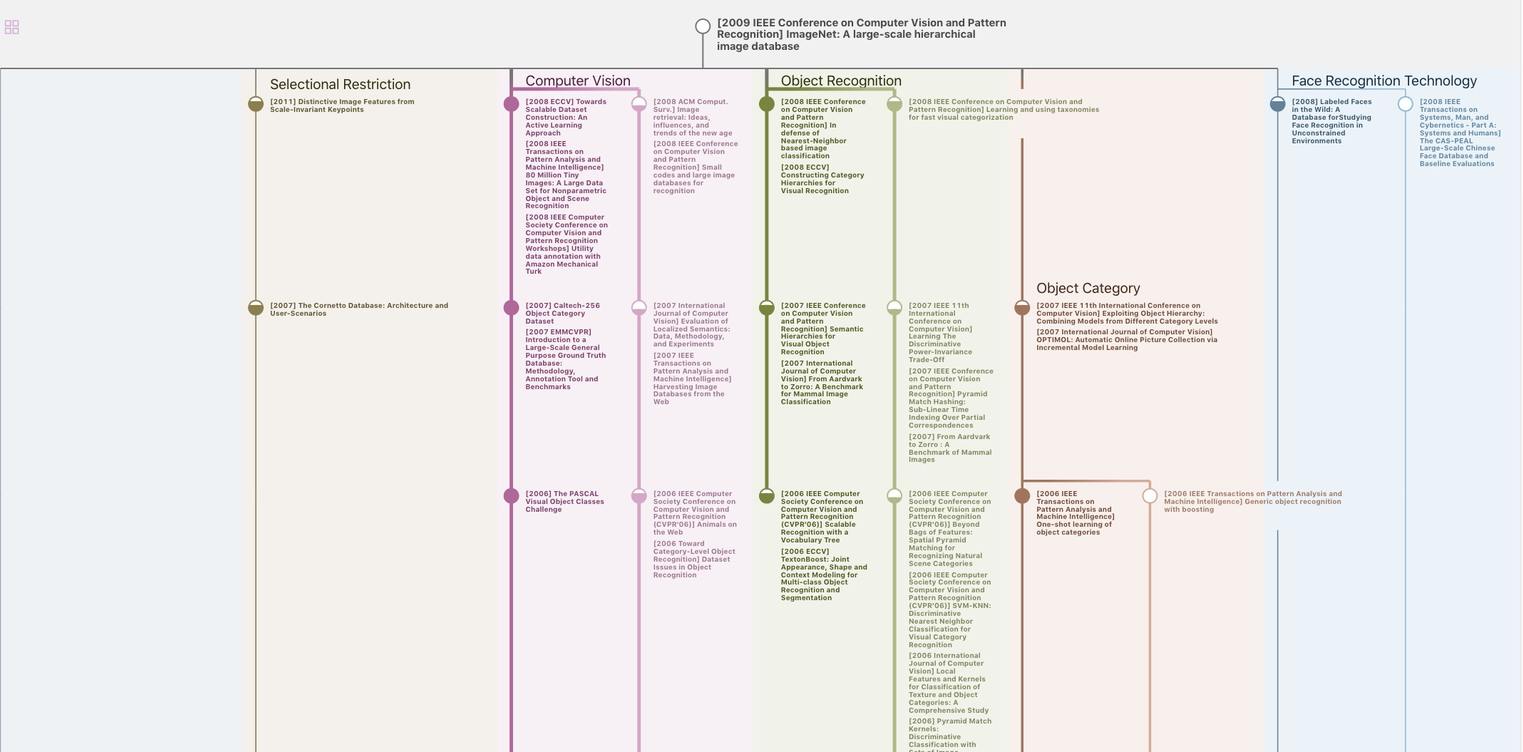
生成溯源树,研究论文发展脉络
Chat Paper
正在生成论文摘要