Do Programs Dream of Electromagnetic Signals? Towards GAN-based Code-to-Signal Synthesis
MILCOM 2023 - 2023 IEEE MILITARY COMMUNICATIONS CONFERENCE(2023)
摘要
The analysis of EM signals has become a popular approach as a means for achieving non-intrusive and external anomaly detection, particularly in the realm of embedded devices. Previous efforts have shown huge potential in terms of discriminative precision between those signals that correspond to normal versus anomalous operations. However, virtually all research in the area neglects the challenge of gathering high-quality signals for the purpose of fingerprinting all possible execution paths of a program. Today, such efforts are mainly human-driven and because there may be hundreds of alternative paths even in simple programs, EM-based anomaly detection approaches exhibit poor scalability. In this work, we introduce a set of methods for the generation of EM signals directly from code. To the best of our knowledge, this is the first effort that aims to address code-to-signal synthesis for purposes of side-channel analysis. The proposed framework is based on Generative Adversarial Networks (GAN) that receive the corresponding assembly (ASM) code of a program as input and in turn produce artificial signals which bear near-identical morphological characteristics as the corresponding real EM signals. Although the proposed method pertains across a wide range of applications we have evaluated our approach specifically for the task of training anomaly detection models. We experimentally showed that synthetic signals can train models that can then detect even stealthy code injection attacks i.e., very challenging minimal code alterations with great accuracy. More specifically, synthetically trained anomaly detection models achieve a near-perfect AUC score of 0.993 for the detection of four malicious instructions and 0.981 for the detection of two malicious instructions. Interestingly, applying rudimentary feature engineering i.e., considering the maximum amplitude of the signal per cycle as opposed
更多查看译文
关键词
Side channel analysis,electromagnetic signals,generative adversarial networks,anomaly detection
AI 理解论文
溯源树
样例
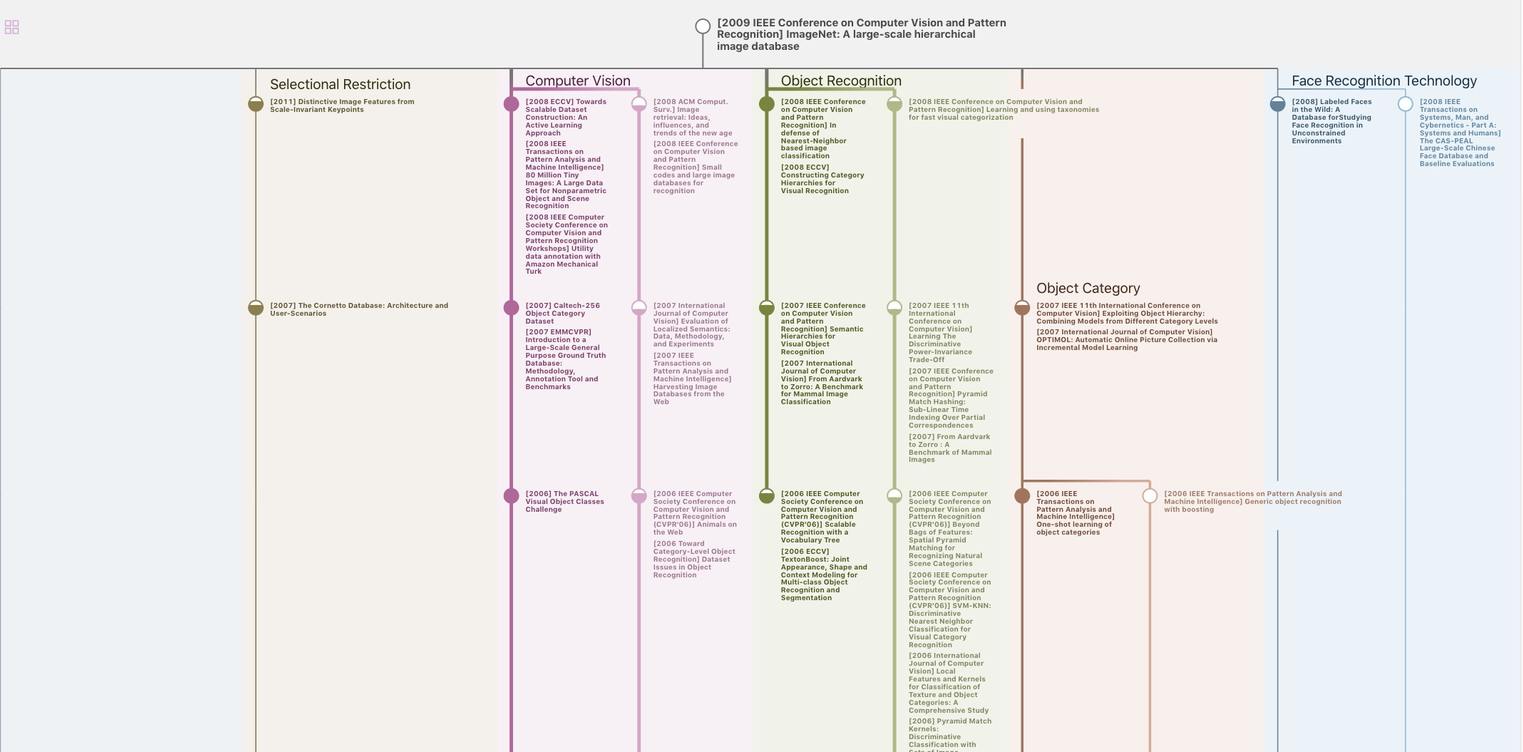
生成溯源树,研究论文发展脉络
Chat Paper
正在生成论文摘要