Comparison of Machine Learning Detection of Low Left Ventricular Ejection Fraction Using Individual ECG Leads.
2023 Computing in Cardiology (CinC)(2023)
摘要
The 12-lead electrocardiogram (ECG) is the most common front-line diagnosis tool for assessing cardiovascular health, yet traditional ECG analysis cannot detect many diseases. Machine learning (ML) techniques have emerged as a powerful set of techniques for producing automated and robust ECG analysis tools that can often predict diseases and conditions not detectable by traditional ECG analysis. Many contemporary ECG-ML studies have focused on utilizing the full 12-lead ECG; however, with the increased availability of single-lead ECG data from wearable devices, there is a clear motivation to explore the development of single-lead ECG-ML techniques. In this study we developed and applied a deep learning architecture for the detection of low left ventricular ejection fraction (LVEF), and compared the performance of this ar-chitecture when it was trained with individual leads of the 12-lead ECG to the performance when trained using the entire 12-lead ECG. We observed that single-lead-trained networks performed similarly to the full 12 -lead-trained network. We also noted patterns of agreement and disagreement between network low LVEF predictions across the different lead-trained networks.
更多查看译文
AI 理解论文
溯源树
样例
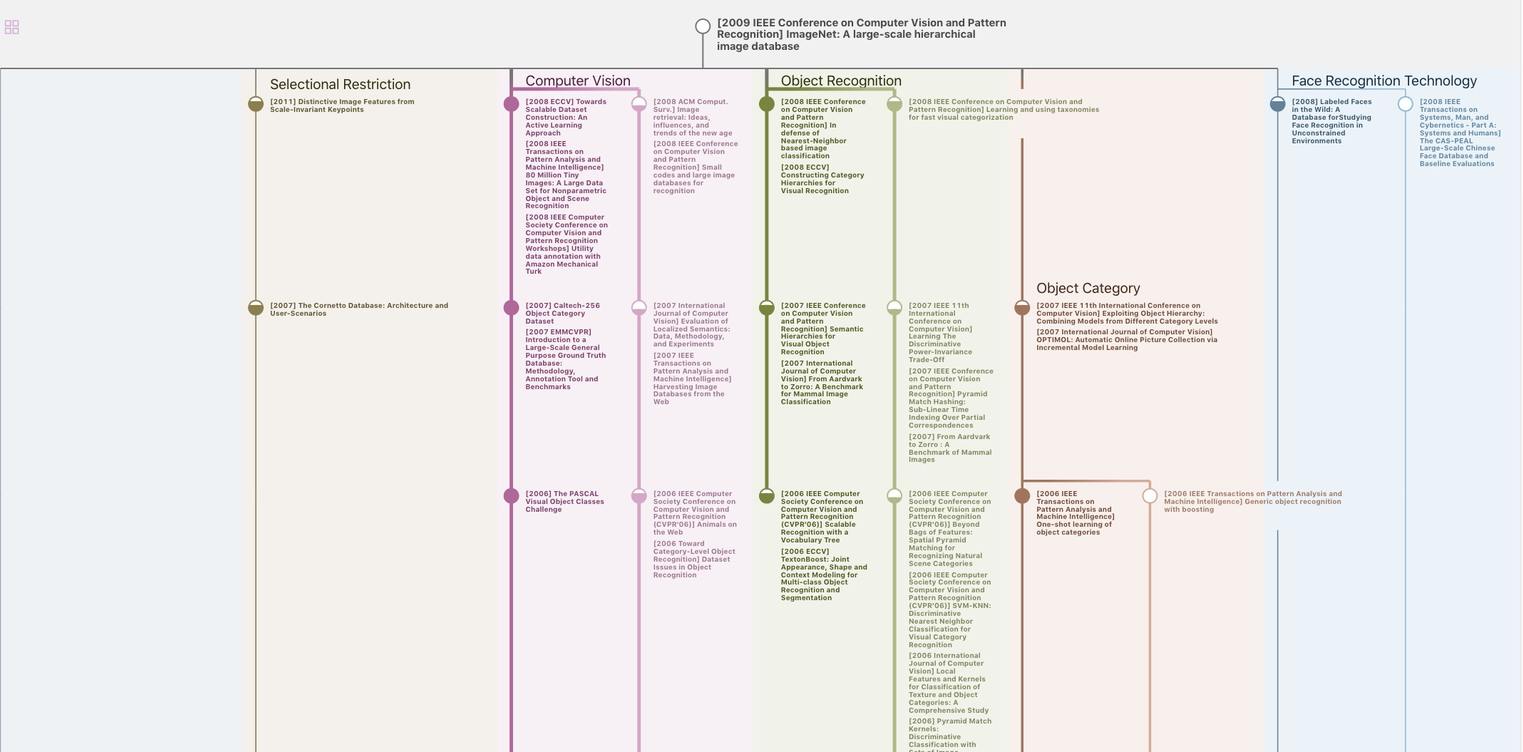
生成溯源树,研究论文发展脉络
Chat Paper
正在生成论文摘要