Embracing the Imaginary: Deep Complex-valued Networks for Heart Murmur Detection.
2023 Computing in Cardiology (CinC)(2023)
摘要
Machine learning for automated heart auscultation offers a scalable solution with the potential to broaden the accessibility of vital healthcare services. While conventional short-time Fourier transform-based audio representations contain both amplitude and phase information, the vast majority of proposed machine audition methods only consider the magnitude, discarding the phase information. In this work, we explore, for the first time, the potential of complex-valued neural networks (CVNNs) for heart sound classification, leveraging all available input information to derive complex representations from sound segments. We showcase the effectiveness of complex-valued neural networks for sound analysis by directly comparing them with real-valued counterparts of our employed neural architectures. On the patient-independent test set of the PhysioNet 2022 Challenge dataset, a complex-valued treatment of two neural network architectures - including HMS-Net, the winning model of PhysioNet 2022 - leads to a consistent 1% absolute improvement in murmur detection accuracy compared to the real-valued baseline. Ensemble model using the non-complex and complex varieties of the two network architectures exhibits a further 6% improvement in sensitivity of murmur and 15% increase in sensitivity of unknown. This highlights the benefits of leveraging the complex domain in deep learning for heart sound analysis.
更多查看译文
AI 理解论文
溯源树
样例
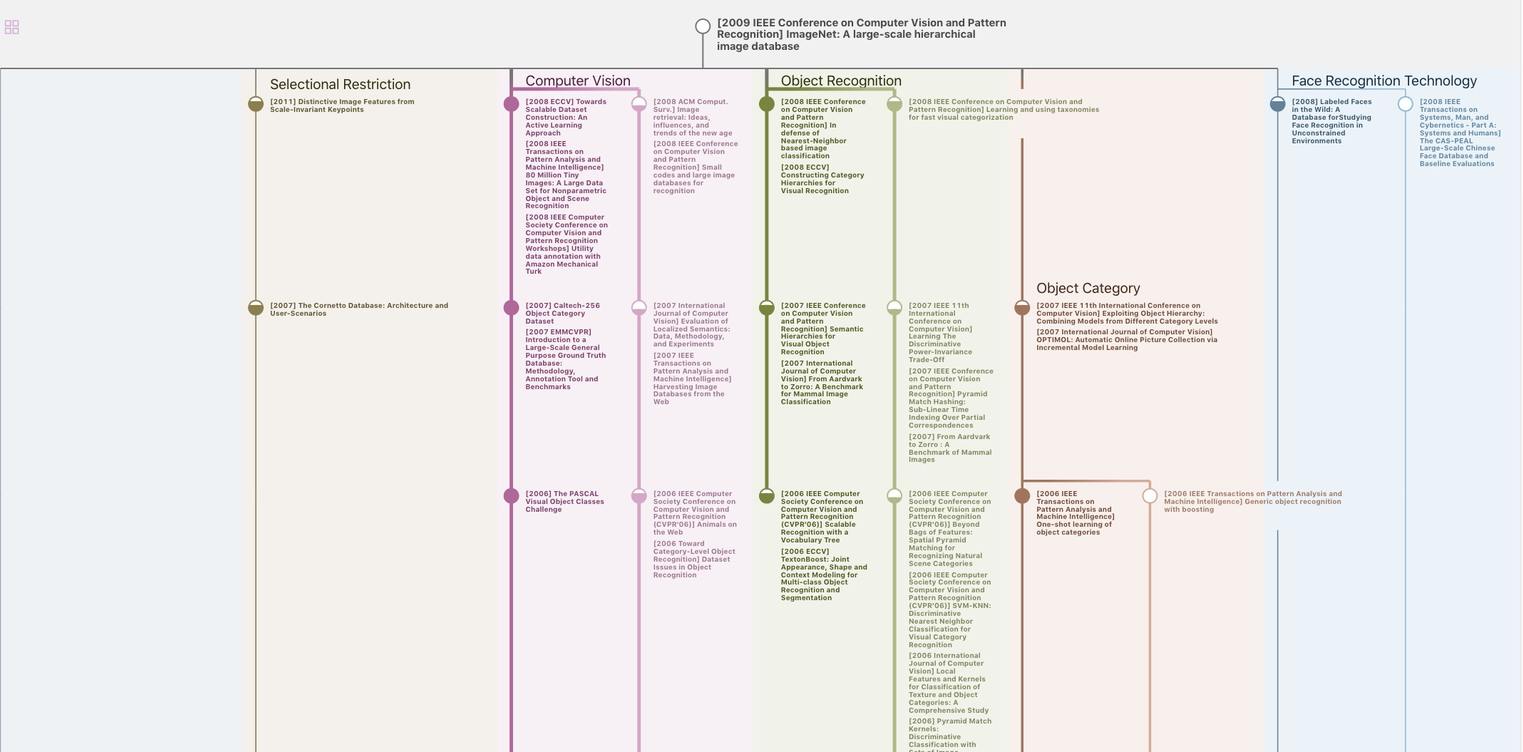
生成溯源树,研究论文发展脉络
Chat Paper
正在生成论文摘要