QuAsyncFL: Asynchronous Federated Learning With Quantization for Cloud-Edge-Terminal Collaboration Enabled AIoT.
IEEE Internet of Things Journal(2024)
摘要
Federated Learning is a promising technique that facilitates cloud–edge–terminal collaboration in Artificial Intelligence of Things (AIoT). It will enable model training without centralizing data, addressing privacy, and security concerns. However, when applied to AIoT, this technique faces several challenges, such as low communication efficiency among terminal devices, edges, and cloud platforms. In this article, we propose a novel approach called asynchronous federated learning with quantization (QuAsyncFL), which combines asynchronous federated learning with an unbiased nonuniform quantizer to address the issue of low communication efficiency. Moreover, we provide a detailed theoretical analysis of convergence with quantized gradients proving that the model could converge to a certain bound. Our experiments demonstrate that QuAsyncFL outperforms the original approach, achieving significant improvements in terms of communication efficiency. The research results represent a further step toward developing cloud–edge–terminal collaboration enabled AIoT.
更多查看译文
关键词
Artificial Intelligence of Things (AIoT),asynchronous federated learning,cloud–edge–terminal collaboration,communication efficiency,quantization
AI 理解论文
溯源树
样例
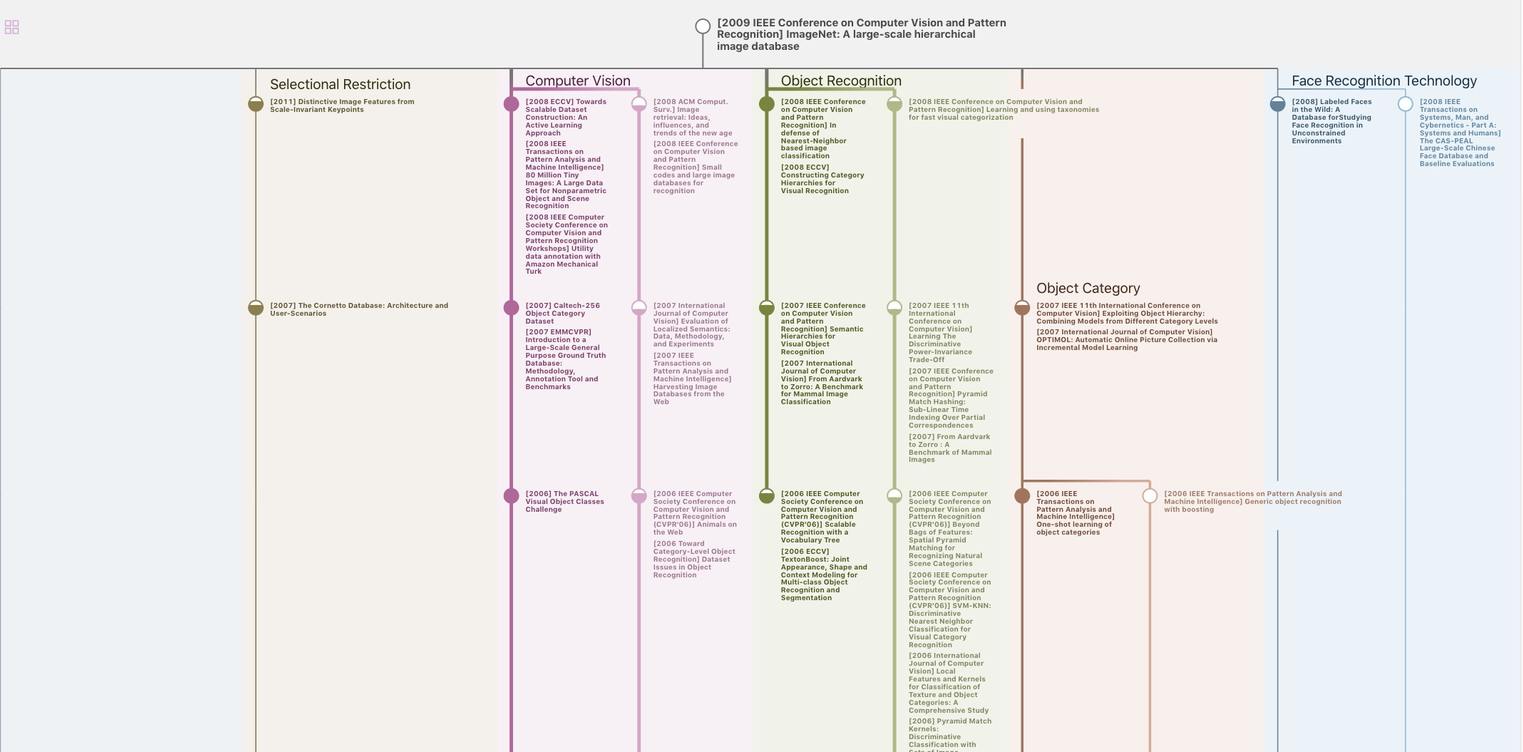
生成溯源树,研究论文发展脉络
Chat Paper
正在生成论文摘要