The Statistical Building Blocks of Animal Movement Simulations
biorxiv(2024)
摘要
Animal movement plays a key role in many ecological processes and has a direct influence on the individual's fitness. Movement tracks can be studied at various spatio-temporal scales, but the movement usually emerges from sequences of fundamental movement elements (FuMEs: e.g., a step or wing flap). The importance of movement in all aspects of the life history of mobile animals highlights the need to develop tools for generating realistic tracks. These individual movement elements are typically not extractable from relocation tracking data: a time series of sequential locations (e.g., GPS fixes) from which we can extract step-size or length (SL, aka velocity when sampling frequency is fixed) and turning-angle (TA) time series. The statistics of these extracted quantities (means, standard deviations, correlations), computed for fixed short segments of track (e.g., 10-30 point sequences), can then be used to categorize such segments into statistical movement elements (StaMEs; e.g. directed fast movement versus random slow movement elements). StaMEs from the same category can be used as a basis for constructing step-selection kernels of a particular movement type, referred to as a canonical activity mode (CAM; e.g. foraging, or commuting). Such CAM kernels can be used to simulate tracks from pure or characteristic mixtures of two or more CAMs, where such mixtures are recognized as a particular type of activity: e.g., foraging or directed walking with interruptions for vigilance or navigation bouts. These activity types in turn (e.g., interspersed sequences of resting, foraging, and directed movements) can then be organized into temporally fixed (24-hour) diel activity routines (DARs; e.g., a day of migration vs a day of local movement). We suggest that a simulator accounting specifically for the hierarchical nature of movement able to generate segments of tracks that may fit CAM length sections of real movement data. Hence, here we present a two-mode, step-selection kernel simulator (Numerus ANIMOVER_1), built using Numerus RAMP technology. We discuss methods for selecting kernel parameters that generate StaMEs comparable to those observed in empirical data, thereby providing a general tool for simulating animal movement in diverse contexts such as evaluation on animal response to landscape changes. We illustrate our methods for extracting StaMEs from both simulated and real data\---|in our case the latter is movement data of two barn owls (( Tyto alba )) in the Harod Valley, Israel. The methods described here may provide a way to fit fine scale simulations to empirical data to in ways that may allow us to identify stressed or diseased individuals.
### Competing Interest Statement
The authors have declared no competing interest.
更多查看译文
AI 理解论文
溯源树
样例
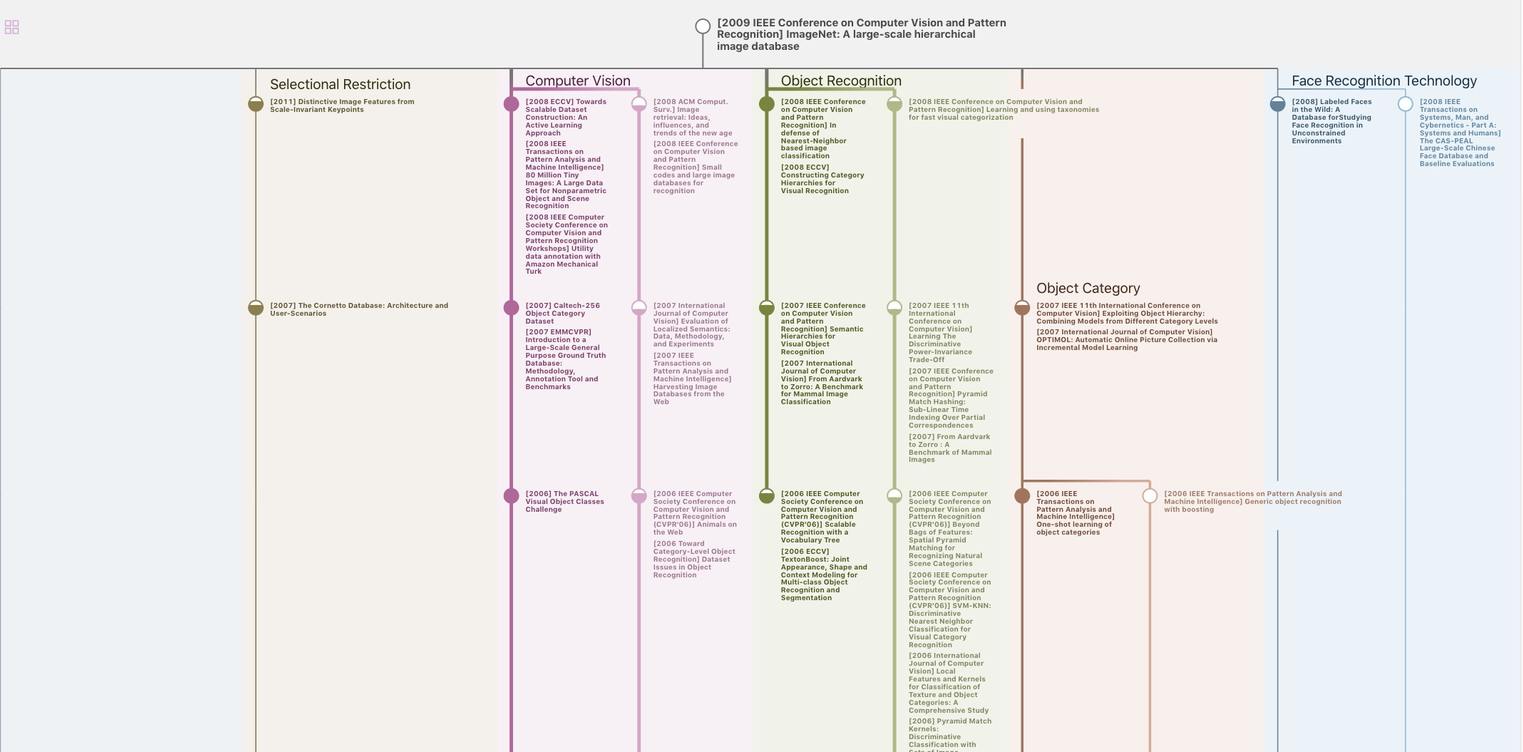
生成溯源树,研究论文发展脉络
Chat Paper
正在生成论文摘要