Sentinel SAR-optical fusion for improving in-season wheat crop mapping at a large scale using machine learning and the Google Earth engine platform
Applied Geomatics(2023)
摘要
In-season wheat growing area identification is of great importance for monitoring crop growth conditions and predicting related yield. In this study, we developed an approach to map wheat crops at a regional scale, using both the Synthetic Aperture Radar (SAR, Sentinel-1, S1) and Copernicus Optical (Sentinel-2, S2) satellite data, to estimate the extent of the wheat growing area. The approach relies on machine learning random forest classification algorithm performed in the Google Earth Engine (GEE) cloud platform. The methodology is based on three experiments, each consisting of the processing of a specific Sentinel time series imageries: a first experiment considering the S1 data solely, a second experiment with the S2 data solely and a third experiment with S1 + S2 data merged. The results showed that the third experiment combining SAR and optical data turned out with the best overall accuracy of 82.36% and a kappa coefficient of 0.77. These results indicate that the integration of Sentinel-1 and Sentinel-2 improved classification accuracy by 1.5 to 6% over the use of Sentinel-2 only. A comprehensive assessment based on survey samples revealed Producer and User accuracies of 84% and 81% respectively; and an F1-score of 0.82. The approach followed in the study provides a basis for mapping seasonal wheat areas that will support planning and policy decisions.
更多查看译文
关键词
Winter wheat,Mapping,Sentinel,Crop classification,Random forest,GEE
AI 理解论文
溯源树
样例
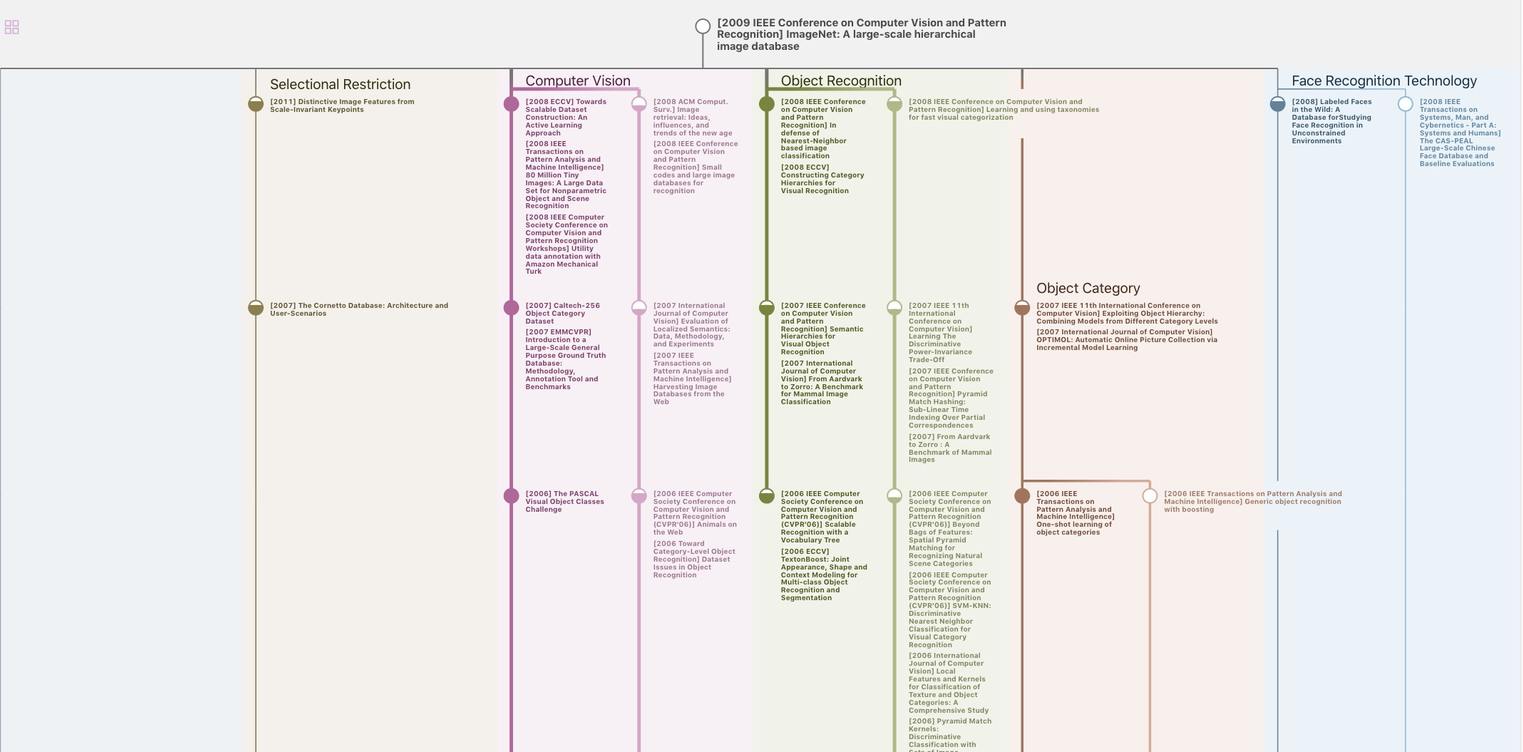
生成溯源树,研究论文发展脉络
Chat Paper
正在生成论文摘要