A federated distillation domain generalization framework for machinery fault diagnosis with data privacy
ENGINEERING APPLICATIONS OF ARTIFICIAL INTELLIGENCE(2024)
摘要
Federated learning is an emerging technology that enables multiple clients to cooperatively train an intelligent diagnostic model while preserving data privacy. However, federated diagnostic models still suffer from a performance drop when applied to entirely unseen clients outside the federation in practical deployments. To address this issue, a Federated Distillation Domain Generalization (FDDG) framework is proposed for machinery fault diagnosis. The core idea is to enable individual clients to access multi-client data distributions in a privacypreserving manner and further explore domain invariance to enhance model generalization. A novel diagnostic knowledge-sharing mechanism is designed based on knowledge distillation, which equips multiple generators to augment fake data during the training of local models. Based on generated data and real data, a low-rank decomposition method is utilized to mine domain invariance, enhancing the model's ability to resist domain shift. Extensive experiments on two rotating machines demonstrate that the proposed FDDG achieves a 3% improvement in accuracy compared to state-of-the-art methods.
更多查看译文
关键词
Fault diagnosis,Rotating machine,Federated learning,Domain generalization,Data privacy
AI 理解论文
溯源树
样例
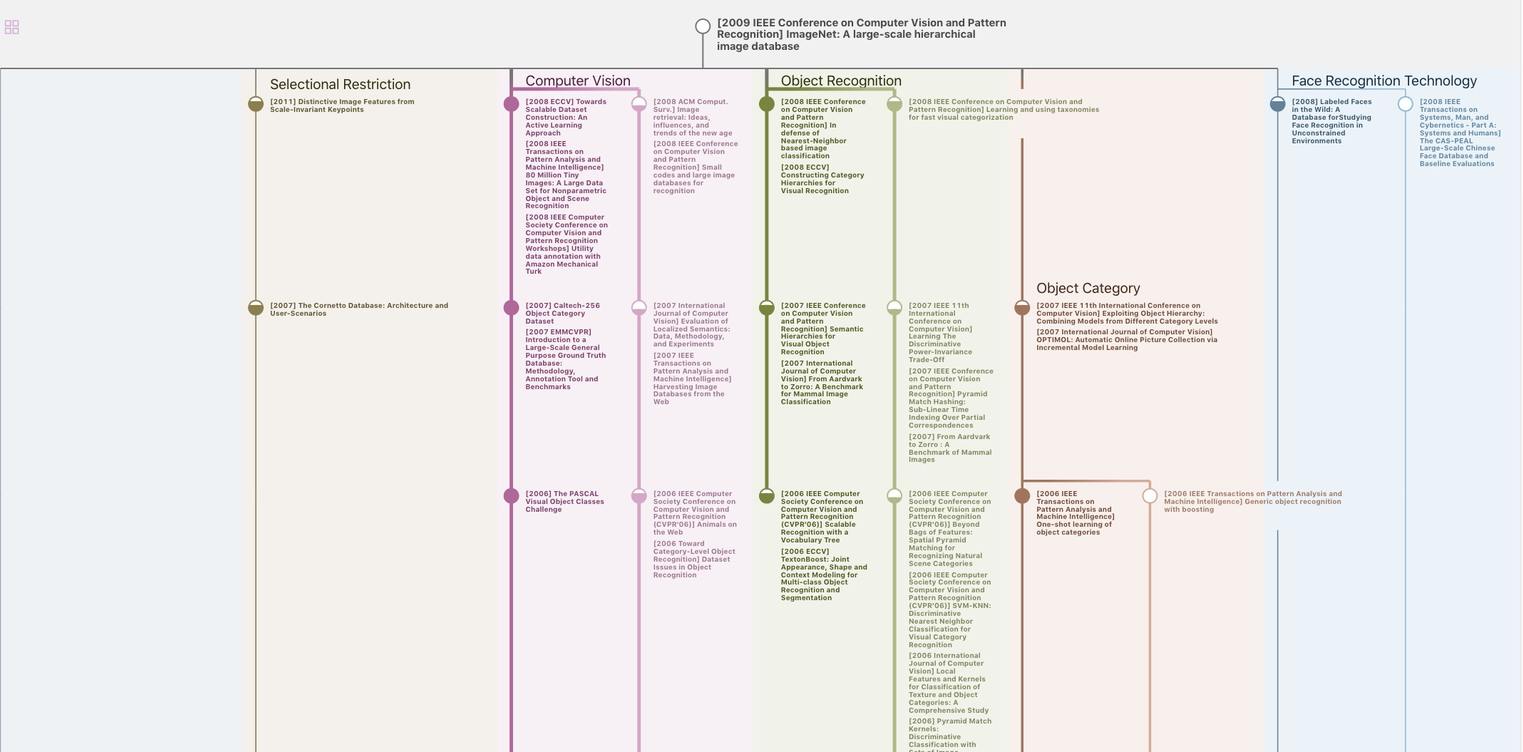
生成溯源树,研究论文发展脉络
Chat Paper
正在生成论文摘要