A 3D-RRT-star algorithm for optimizing constrained mountain railway alignments
ENGINEERING APPLICATIONS OF ARTIFICIAL INTELLIGENCE(2024)
摘要
Railway alignment development in mountainous regions is a complex problem, especially when there are numerous obstacles in the study area. Obtaining a feasible solution that satisfies all the obstacle constraints requires considerable computing resources and time. To solve this problem, a graph-based shortest path method, i.e., three-dimensional rapidly exploring random tree star (3D-RRT-star), is customized. Three main innovations are included in this method: (1) A heuristic sampling process is proposed to avoid getting the RRT search trapped into local ranges and overlooking possible path alternatives by combining a specially designed RRT node sampling method and a railway spatially-reachable analysis. (2) A multi-level constraint discretization approach is proposed to describe the obstacles in the study area, while procedures are developed to tackle the obstacle constraints dynamically during the search process. (3) An evolutionary search method integrating a sampling strategy and constraint handling operator is devised for generating a set of dissimilar RRT paths, which are finally refined into railway alignments satisfying curve constraints. Ultimately, the proposed method is applied to a realistic railway case. The experimental results confirm that it can yield a better alignment than the best manually obtained solution. Furthermore, its search efficiency is compared to a previous optimization method for this problem and the results reveal significant improvement.
更多查看译文
关键词
Railway design,Alignment optimization,Rapidly exploring random tree,Heuristic sampling process,Evolutionary search,Constrained optimization
AI 理解论文
溯源树
样例
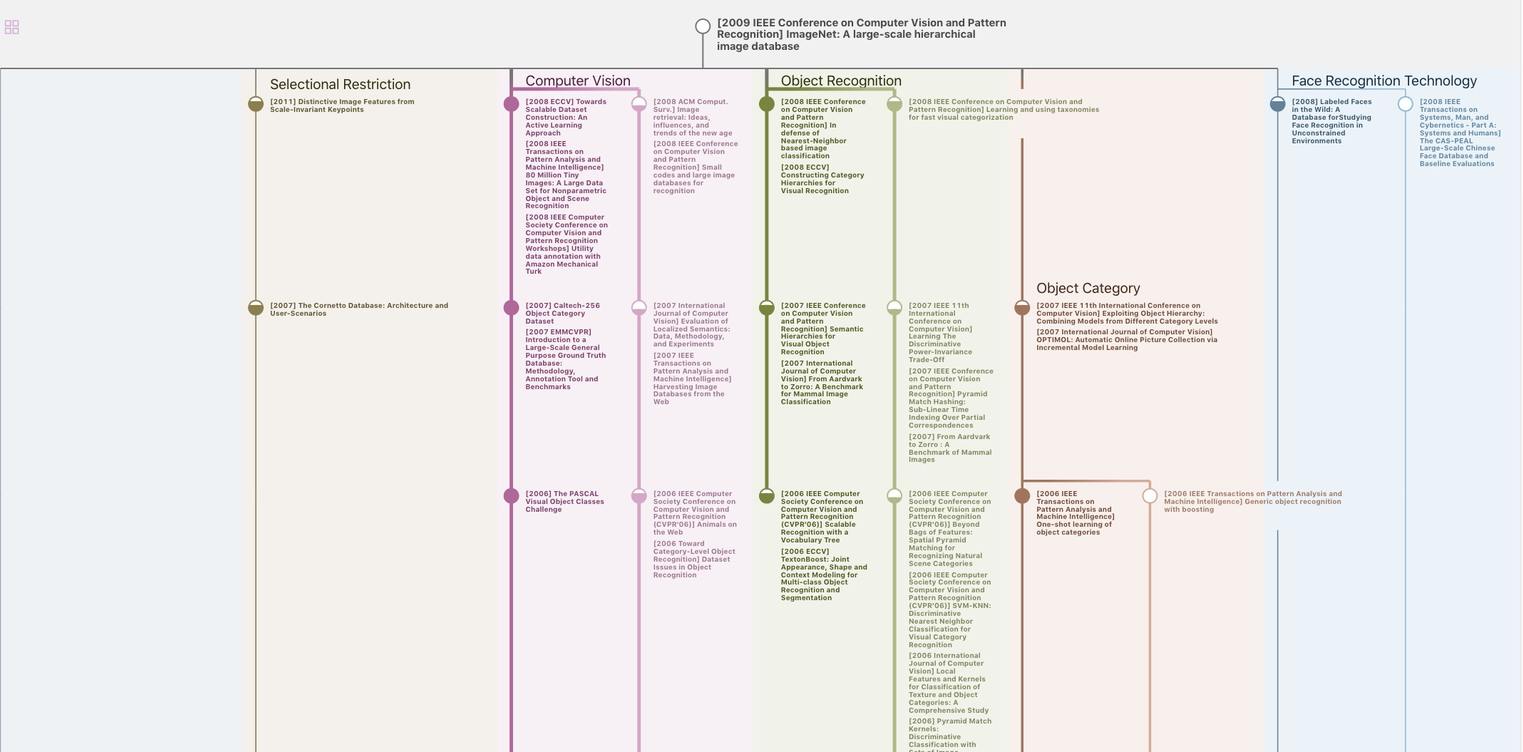
生成溯源树,研究论文发展脉络
Chat Paper
正在生成论文摘要