Industrial Internet of Things embedded devices fault detection and classification. A case study
INTERNET OF THINGS(2024)
摘要
Industries transition to the Industry 4.0 paradigm requires solutions based on devices attached to machines that allow monitoring and control of industrial equipment. Monitoring is essential to ensure devices' proper operation against different aggressions. We propose an approach to detect and classify faults that are typical in these devices, based on machine learning techniques that use energy, processing, and main application use as features. The proposal was validated using a dataset collected from a testbed executing a typical equipment monitoring application. The proposed machine learning pipeline uses a decision tree-based model for fault detection (with 99.4% accuracy, 99.7% precision, 99.6% recall, 75.2% specificity, and 99.7% F1) followed by a Semi-Supervised Graph-Based model (with 99.3% accuracy, 96.4% precision, 96.1% recall, 99.6% specificity, and 96.2% F1) for further fault classification. The obtained results demonstrate that machine learning techniques, based on easily obtainable metrics, help coping with common device faults.
更多查看译文
关键词
Embedded devices,Fault detection and diagnosis,Industrial Internet of Things,Machine learning
AI 理解论文
溯源树
样例
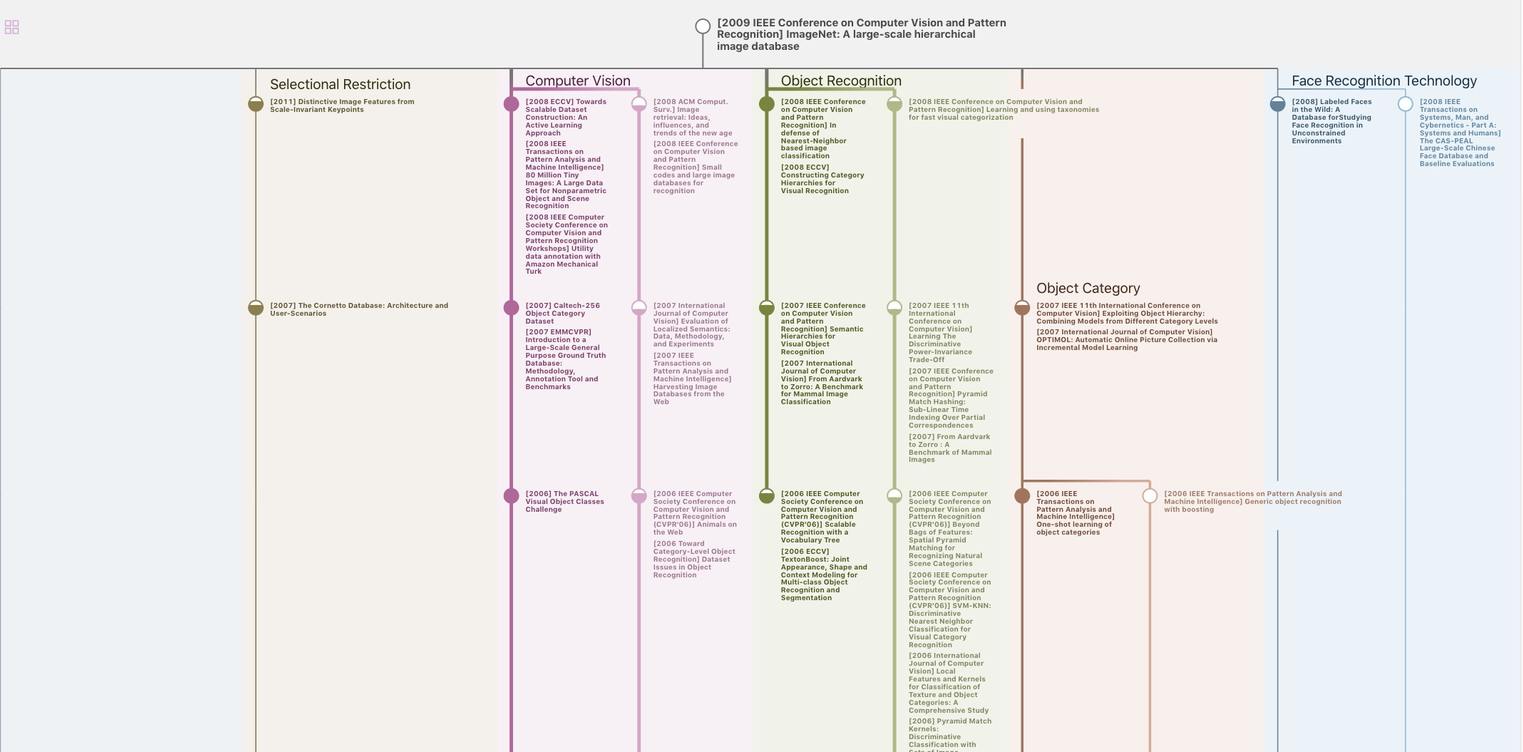
生成溯源树,研究论文发展脉络
Chat Paper
正在生成论文摘要