Predicting meltpool depth and primary dendritic arm spacing in laser powder bed fusion additive manufacturing using physics-based machine learning
MATERIALS & DESIGN(2024)
摘要
The long-term goal of this work is to predict and control the microstructure evolution in metal additive manufacturing processes. In pursuit of this goal, we developed and applied an approach which combines physicsbased thermal modeling with machine learning to predict two important microstructure-related characteristics, namely, the meltpool depth and primary dendritic arm spacing in Nickel Alloy 718 parts made using the laser powder bed fusion (LPBF) process. Microstructure characteristics are critical determinants of functional physical properties, e.g., yield strength and fatigue life. Currently, the microstructure of LPBF parts is optimized through a cumbersome build-and-characterize empirical approach. Rapid and accurate models for predicting microstructure evolution are therefore valuable to reduce process development time and achieve consistent properties. However, owing to their computational complexity, existing physics-based models for predicting the microstructure evolution are limited to a few layers, and are challenging to scale to practical parts. This paper addresses the aforementioned research gap via a novel physics and data integrated modeling approach. The approach consists of two steps. First, a rapid, part-level computational thermal model was used to predict the temperature distribution and cooling rate in the entire part before it was printed. Second, the foregoing physicsbased thermal history quantifiers were used as inputs to a simple machine learning model (support vector machine) trained to predict the meltpool depth and primary dendritic arm spacing based on empirical materials characterization data. As an example of its efficacy, when tested on a separate set of samples from a different build, the approach predicted the primary dendritic arm spacing with root mean squared error approximate to 110 nm. This work thus presents an avenue for future physics-based optimization and control of microstructure evolution in LPBF.
更多查看译文
关键词
Laser powder bed fusion,Microstructural evolution,Primary dendritic arm spacing (PDAS),Meltpool depth,Thermal modeling,Physics-based machine learning
AI 理解论文
溯源树
样例
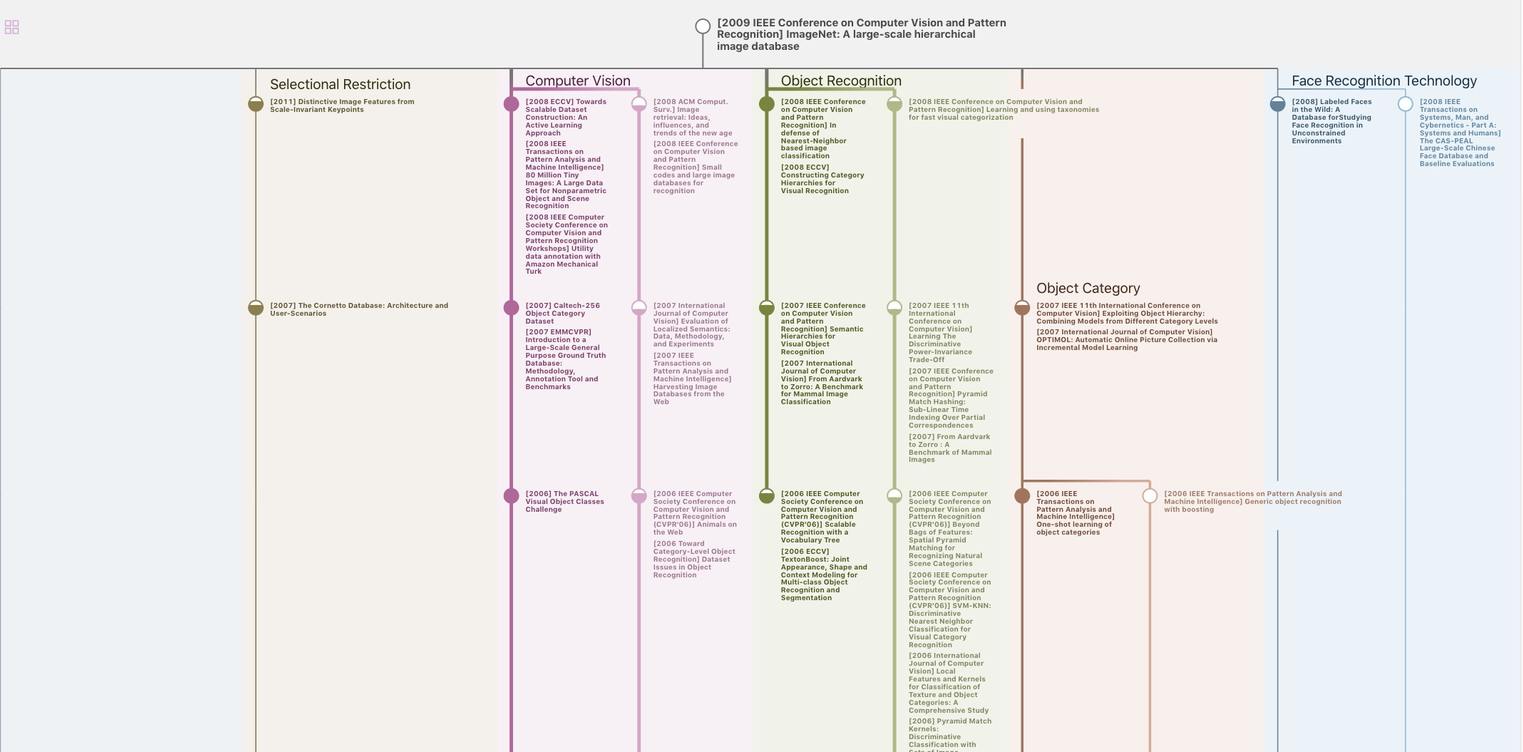
生成溯源树,研究论文发展脉络
Chat Paper
正在生成论文摘要