Time-Embedded EEG Sequence Learning for Comatose Patients' Prognosis.
2023 Computing in Cardiology (CinC)(2023)
摘要
In an intensive care unit (ICU), an accurate prognosis of comatose patients' recovery is critical for ongoing medical interventions. Patient prognosis guides decisions around continuation of care. Patients may recover from a coma despite poor initial prognosis; thus, more reliable predictors for recovery are needed. Electroencephalography (EEG)-based neurological markers may complement the current prognosis. The PhysioNet Challenge 2023 includes a dataset of EEG signals and clinical attributes from a total of 1020 adult patients in ICUs that remained in a coma after cardiac arrest, 607 of whom were dedicated to algorithmic training. We conceptualized a novel time-embedded feature space for continuous EEG followed by a bidirectional long short-term memory for learning any temporal patterns associated with comatose patients' recovery. We extracted EEG-related attributes: dynamic range, skewness, kurtosis, and subband
$\delta,\ \theta,\ \alpha$
and
$\beta$
) power after selecting 1 minute/hour EEG using a preprocessing algorithm. With a false positive rate < 0.05, the true positive rate (TPR) was the scoring metric at the 72
nd
hour post cardiac arrest. Our team, USYD_BrainBuzz ranked 27
th
and achieved scores of 0.26, 0.51 and 0.40 on the training, validation and testing sets, respectively. Results implicated that our approach has shown promise for continuous monitoring of comatose patients.
更多查看译文
AI 理解论文
溯源树
样例
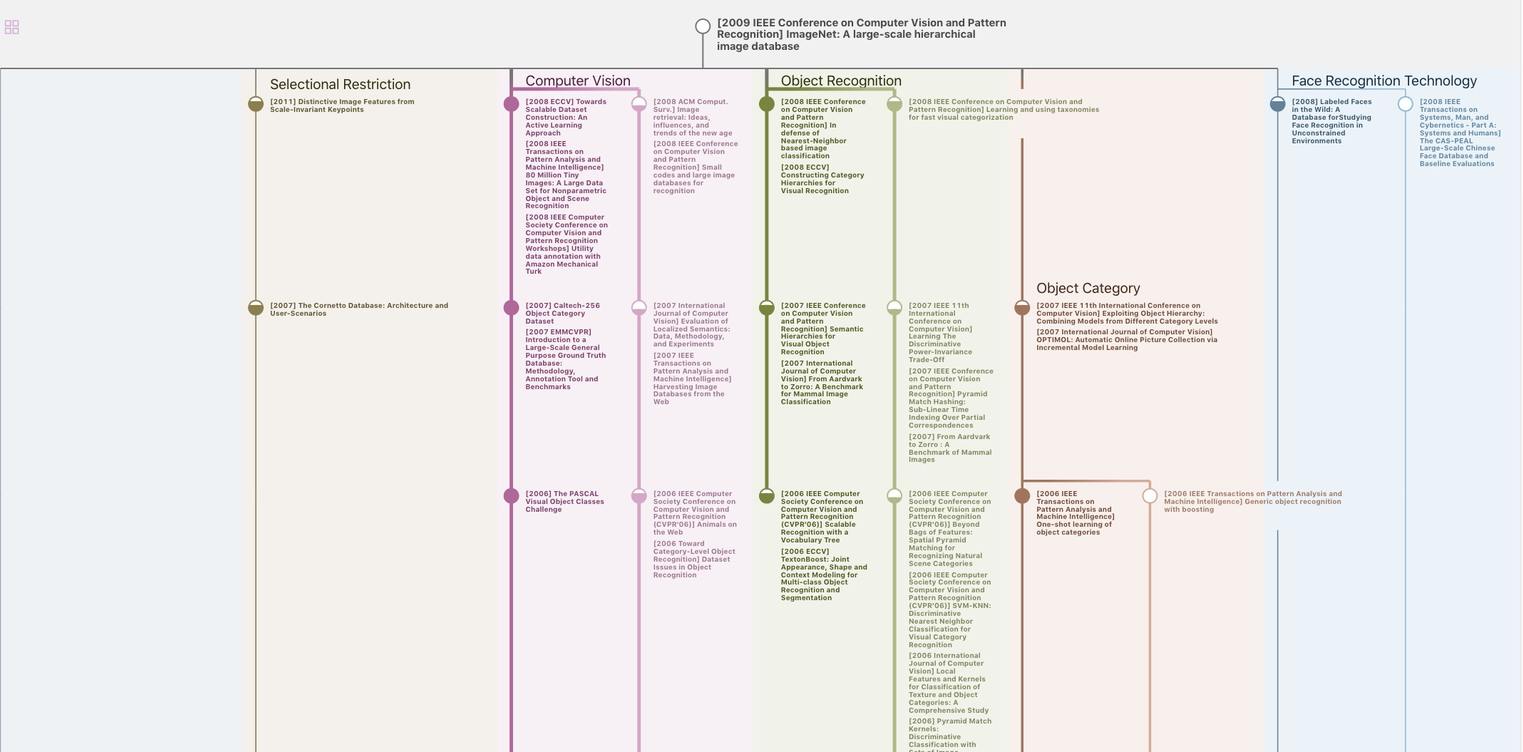
生成溯源树,研究论文发展脉络
Chat Paper
正在生成论文摘要