MMCTNet: Multi-Modal Cony-Transformer Network for Predicting Good and Poor Outcomes in Cardiac Arrest Patients.
2023 Computing in Cardiology (CinC)(2023)
摘要
Electroencephalography (EEG) has been demonstrated to be a valuable tool for predicting neurological outcomes after cardiac arrest. However its complexity limits timely interpretation. As part of George B. Moody Physionet Challenge 2023, our team (CQUPT_FP_mana) proposes a Multi-Modal Conv-Transformer network to accurately and timely assess probability of coma recovery with complex EEG. We select the five-minute EEGs nearest to the six moments of 12h, 24h, 36h, 48h, 60h, and 72h, respectively, resample them to 100Hz, filter them using a 5-order Butterworth bandpass filter, and slice the EEGs into 10-second slices. The one-dimensional representation of the EEG and the time-frequency spectrograms after a short-time Fourier transform are then used as inputs to the network structure. Our network consists of two convolutional branches, a transformer encoder, and a classification header. In the end, our network scored 0.48 points in the tournament, placing us 19th out of all challenged teams.
更多查看译文
AI 理解论文
溯源树
样例
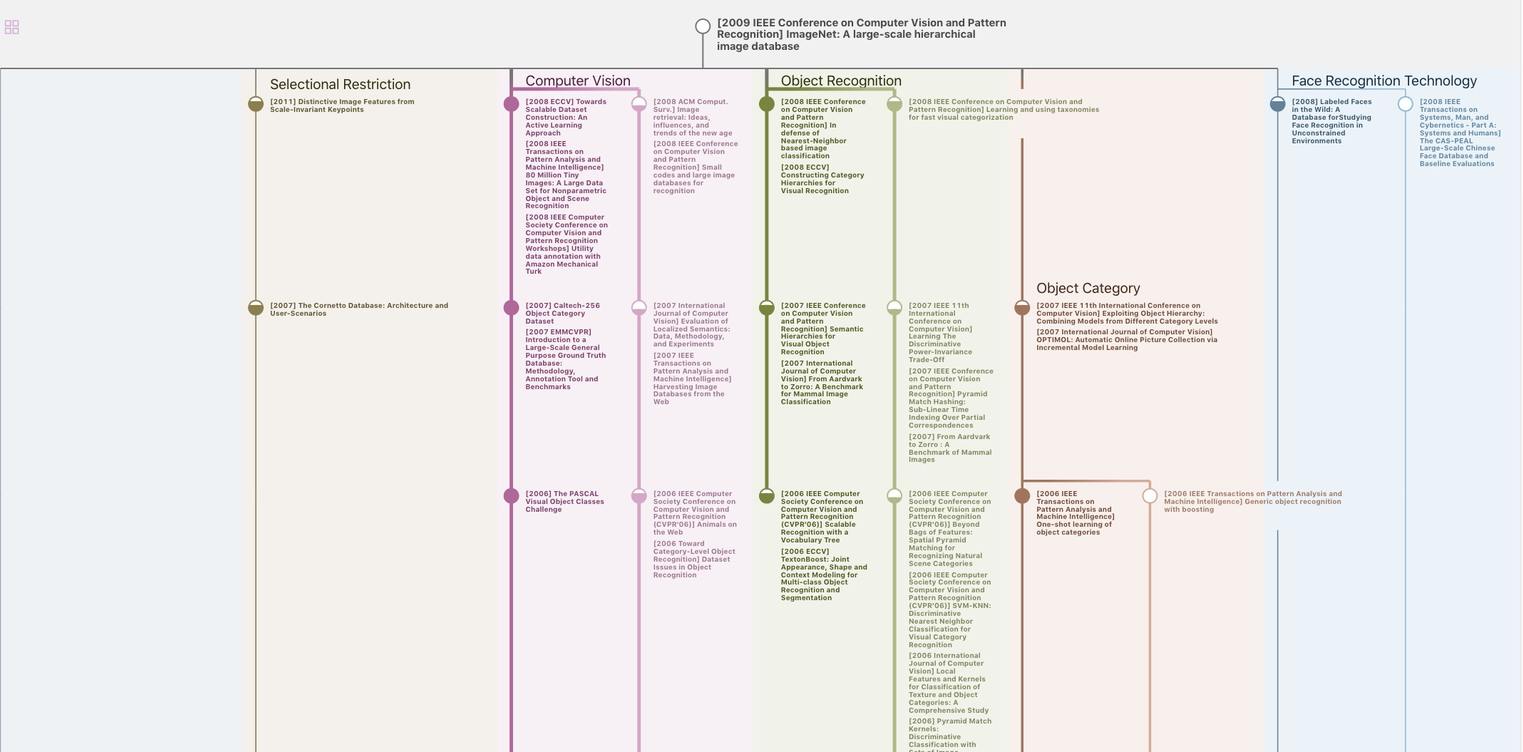
生成溯源树,研究论文发展脉络
Chat Paper
正在生成论文摘要