A Tensor Decomposition-Based Feature Extraction Method to Predict Neurological Recovery from Coma After Cardiac Arrest Using EEG Signals.
2023 Computing in Cardiology (CinC)(2023)
摘要
Electroencephalogram (EEG) patterns can reveal important details regarding the level of neurological recovery in comatose individuals who have undergone cardiac arrest hospitalization. Automated algorithms based on EEG signal processing and machine learning can be used to estimate a patient's chances of regaining consciousness. This study uses tunable Q-factor wavelet transform (TQWT)-based signal refinement and Tensor decomposition-based feature extraction from EEG records to construct probability-based favorable or bad outcome labels for prognosis. TQWT-based signal decomposition into sub-bands of EEG signals employing optimal settings for feature extraction to enhance critical care patterns. A 3-way tensor per record is created using scattering transform, which captures time-frequency information. The core tensor from Tucker decomposition of the produced tensor is utilized to get 1-D feature vectors along with other time and frequency domain features for bagging ensemble classifier learning. We participated in the George B. Moody PhysioNet Challenge 2023 as team ‘Medics’ and obtained 7
th
ranking with challenge score of 0.69 on challenge hidden test data for prognostication 72 hours after return of spontaneous circulation (ROSC).
更多查看译文
AI 理解论文
溯源树
样例
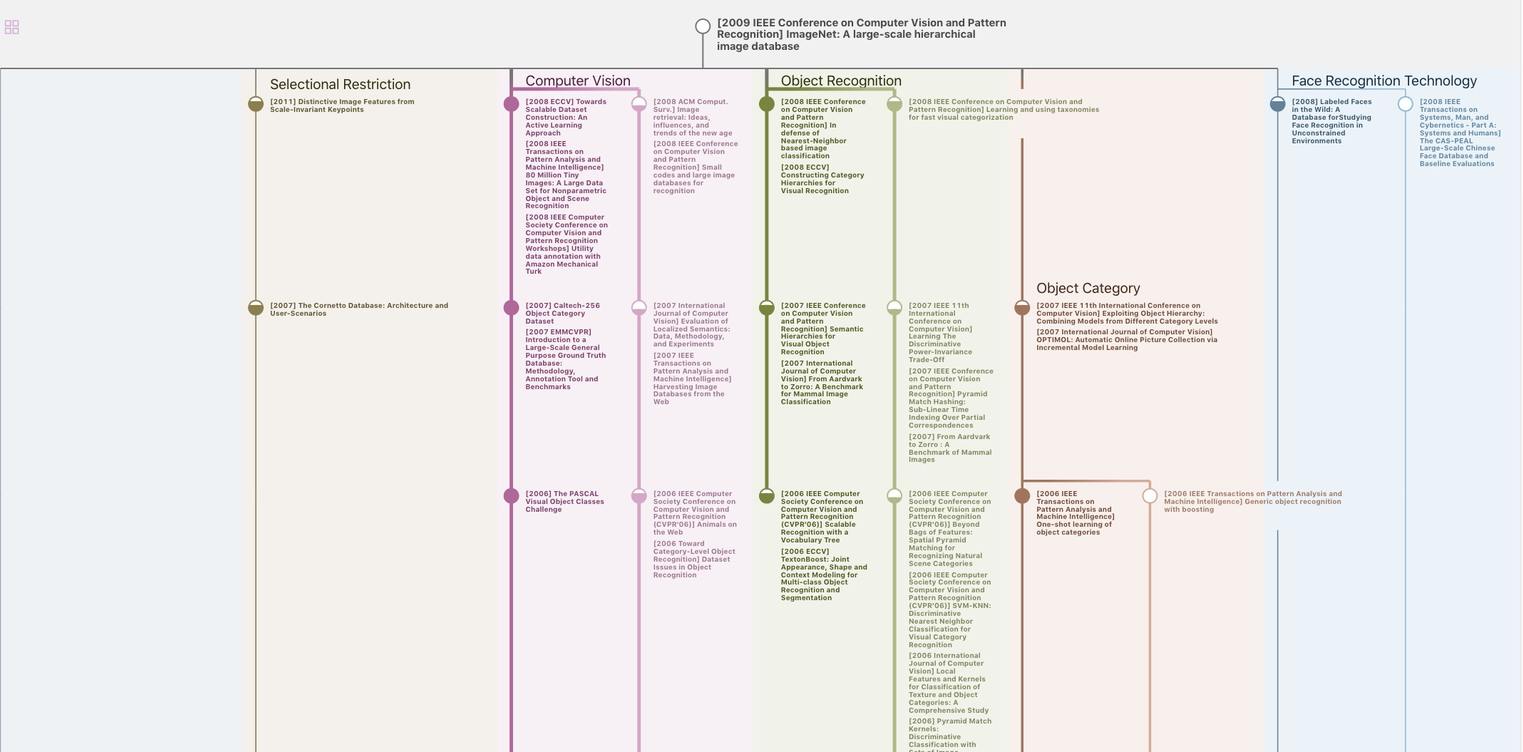
生成溯源树,研究论文发展脉络
Chat Paper
正在生成论文摘要