Embed Trajectory Imitation in Reinforcement Learning: A Hybrid Method for Autonomous Vehicle Planning.
DTPI(2023)
摘要
Learning-based autonomous vehicle trajectory planning methods have shown excellent performance in a variety of complex traffic scenarios. However, the existing imitation learning (IL) and reinforcement learning (RL) algorithms still have their limitations, such as poor safety and generalizability for IL, and low data efficiency for RL. To leverage their respective advantages and mitigate the limitations, this paper proposes a novel hybrid RL algorithm for autonomous vehicle planning, where IL is embedded in it to guide its exploration with expert knowledge. Different from existing approaches, we use multi-step trajectory prediction instead of behavior cloning as the IL method integrated with online RL. Through such design, we make a further step in the research about how expert demonstration can be helpful to RL. Moreover, we conduct parallel training and testing of the algorithm based on real-world driving data. Experimental results demonstrate that our proposed approach outperforms standalone IL and RL methods, and performs better than RL methods enhanced by behavior cloning.
更多查看译文
关键词
Imitation Learning,Trajectory Planning,Deep Reinforcement Learning,Autonomous Driving
AI 理解论文
溯源树
样例
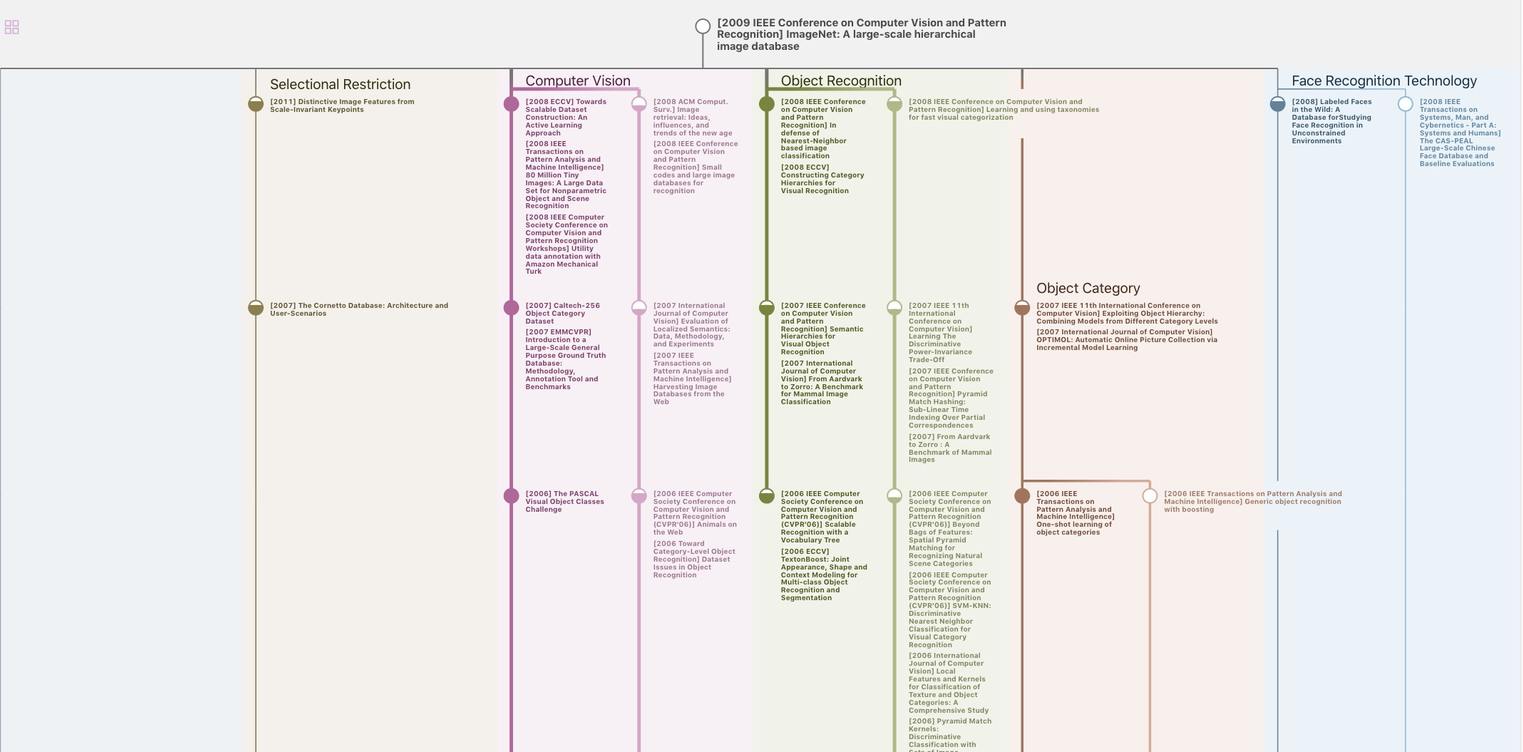
生成溯源树,研究论文发展脉络
Chat Paper
正在生成论文摘要