Single-Cell Transcriptomic, hdWGCNA, and Machine Learning reveal the Role of DYNLL1 in Lung Adenocarcinoma within Epithelial Cells
crossref(2023)
Abstract
Abstract Background: Lung cancer has the highest morbidity and mortality worldwide. Lung adenocarcinoma (LUAD) is the most common histological subtype and tends to be diagnosed at advanced stages with poor prognosis. Tumor heterogeneity and lack of prognostic biomarkers pose significant challenges for personalized therapy. Single-cell sequencing provides new opportunities to characterize intratumor heterogeneity and identify prognostic markers for early diagnosis or treatment target. Methods: In this study, we analyzed single cell RNA sequencing data of 11 normal lung tissue samples and 11 LUAD samples from the GEO dataset GSE131907. High dimensional Weighted gene co-expression network analysis (hdWGCNA) was applied to the single-cell expression profile of epithelial cells to construct gene co-expression modules. Subsequently, a 13-gene prognostic signature was established by integrating 207 models with 15 machine learning algorithms in a cross validation framework. The correlation of the hub gene DYNLL1 with prognosis, clinical characteristics and biological pathways were comprehensively analyzed. Furthermore, loss-of-function assays by siRNA knockdown of DYNLL1 were performed to examine its functional role in LUAD cell lines. Results: Significant differences in immune cell type distributions were observed between normal and tumor tissues, indicating important immune dysregulations during LUAD development. hdWGCNA identified 27 modules in LUAD epithelial cells potentially associated with tumorigenesis and disease outcomes. The 13-gene prognostic model effectively stratified patients into high- and low-risk groups with distinct survival. DYNLL1 was identified as an independent prognostic biomarker and potential therapeutic target. High expression of DYNLL1 positively correlated with advanced tumor stage and poorer survival. Knockdown of DYNLL1 expression suppressed cancer cell proliferation and invasion capacities. Conclusions: Our study provided in-depth characterization of LUAD at single-cell resolution, identified key gene modules and prognostic biomarkers, and suggested DYNLL1 as a candidate prognostic predictor and therapeutic target, pending further verification in clinical settings. Keywords: Lung adenocarcinoma、scRNA_seq、hdWGCNA、machine learning 、Prognostic biomarker
MoreTranslated text
AI Read Science
Must-Reading Tree
Example
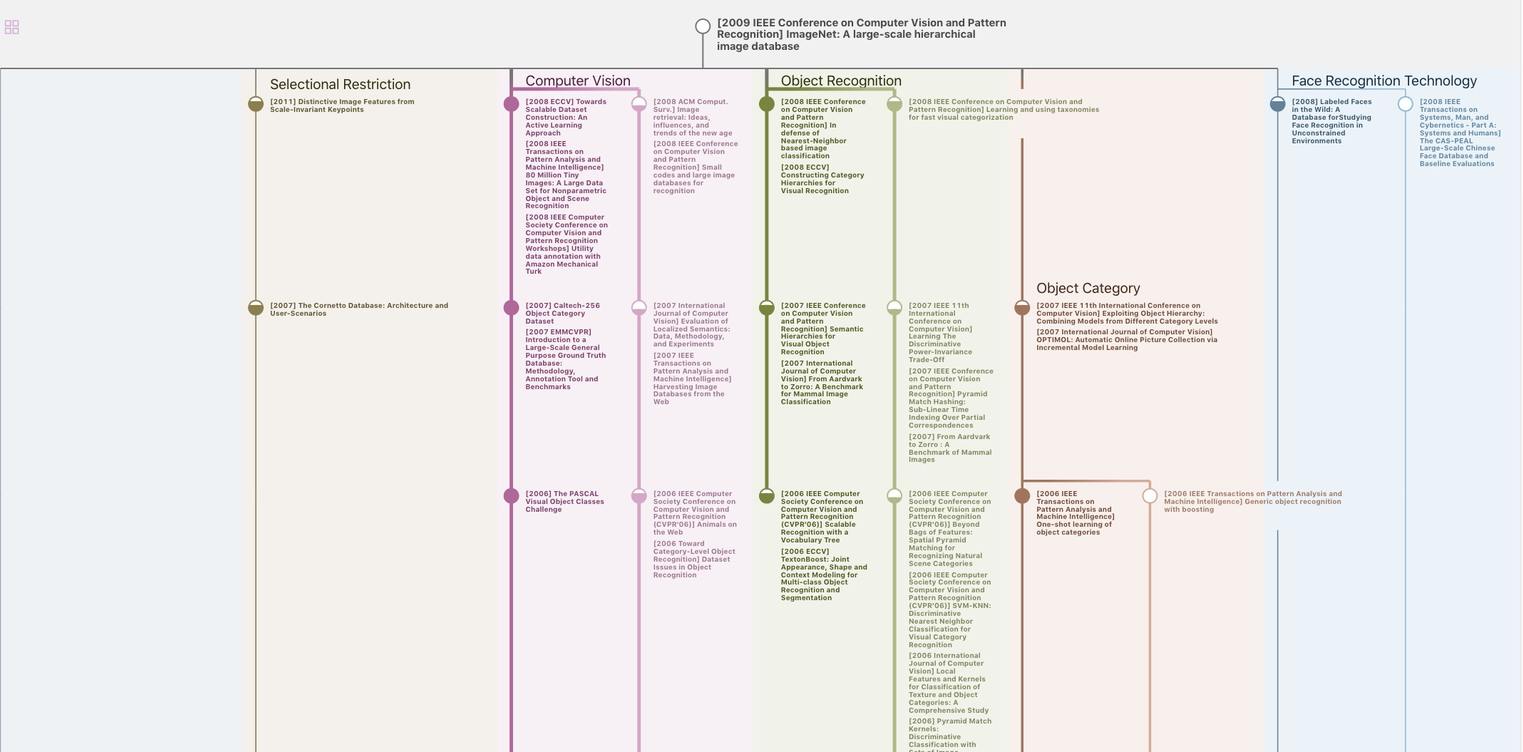
Generate MRT to find the research sequence of this paper
Chat Paper
Summary is being generated by the instructions you defined