Learning Deformable Hypothesis Sampling for Accurate PatchMatch Multi-View Stereo
AAAI 2024(2024)
Abstract
This paper introduces a learnable Deformable Hypothesis Sampler (DeformSampler) to address the challenging issue of noisy depth estimation in faithful PatchMatch multi-view stereo (MVS). We observe that the heuristic depth hypothesis sampling modes employed by PatchMatch MVS solvers are insensitive to (i) the piece-wise smooth distribution of depths across the object surface and (ii) the implicit multi-modal distribution of depth prediction probabilities along the ray direction on the surface points. Accordingly, we develop DeformSampler to learn distribution-sensitive sample spaces to (i) propagate depths consistent with the scene's geometry across the object surface and (ii) fit a Laplace Mixture model that approaches the point-wise probabilities distribution of the actual depths along the ray direction. We integrate DeformSampler into a learnable PatchMatch MVS system to enhance depth estimation in challenging areas, such as piece-wise discontinuous surface boundaries and weakly-textured regions. Experimental results on DTU and Tanks & Temples datasets demonstrate its superior performance and generalization capabilities compared to state-of-the-art competitors. Code is available at https://github.com/Geo-Tell/DS-PMNet.
MoreTranslated text
Key words
CV: 3D Computer Vision,CV: Computational Photography, Image & Video Synthesis
AI Read Science
Must-Reading Tree
Example
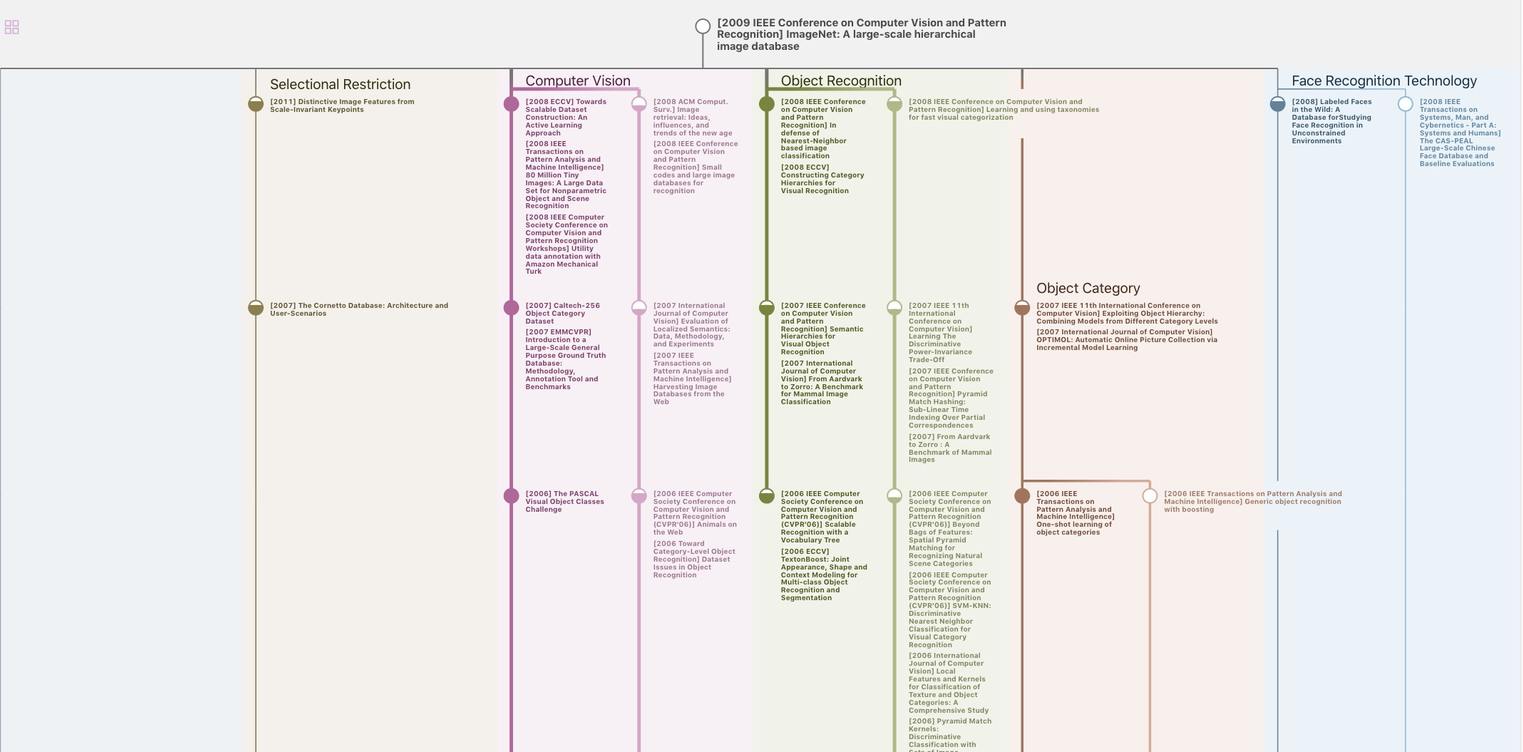
Generate MRT to find the research sequence of this paper
Chat Paper
Summary is being generated by the instructions you defined