Self-Supervised Depth Completion Guided by 3D Perception and Geometry Consistency
CoRR(2023)
摘要
Depth completion, aiming to predict dense depth maps from sparse depth measurements, plays a crucial role in many computer vision related applications. Deep learning approaches have demonstrated overwhelming success in this task. However, high-precision depth completion without relying on the ground-truth data, which are usually costly, still remains challenging. The reason lies on the ignorance of 3D structural information in most previous unsupervised solutions, causing inaccurate spatial propagation and mixed-depth problems. To alleviate the above challenges, this paper explores the utilization of 3D perceptual features and multi-view geometry consistency to devise a high-precision self-supervised depth completion method. Firstly, a 3D perceptual spatial propagation algorithm is constructed with a point cloud representation and an attention weighting mechanism to capture more reasonable and favorable neighboring features during the iterative depth propagation process. Secondly, the multi-view geometric constraints between adjacent views are explicitly incorporated to guide the optimization of the whole depth completion model in a self-supervised manner. Extensive experiments on benchmark datasets of NYU-Depthv2 and VOID demonstrate that the proposed model achieves the state-of-the-art depth completion performance compared with other unsupervised methods, and competitive performance compared with previous supervised methods.
更多查看译文
AI 理解论文
溯源树
样例
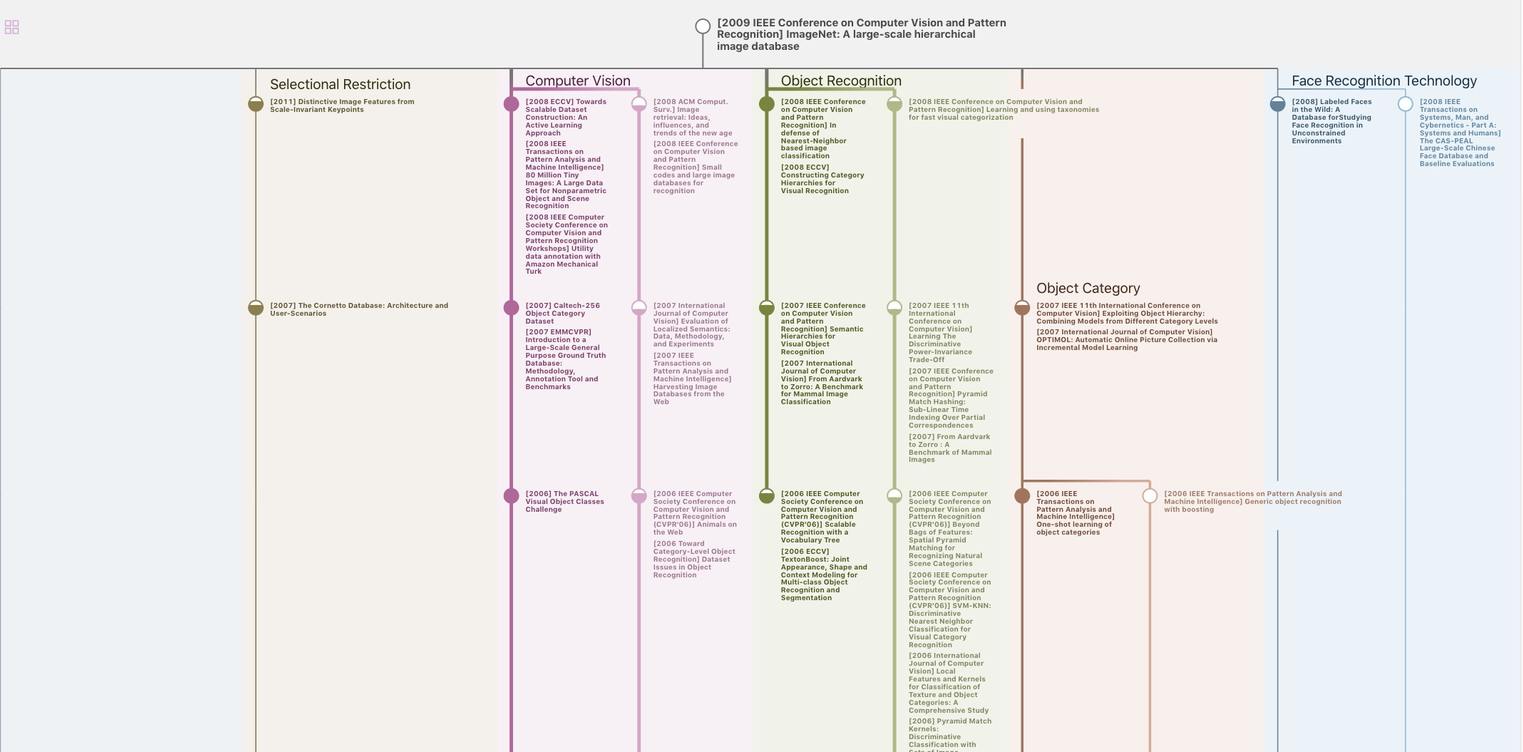
生成溯源树,研究论文发展脉络
Chat Paper
正在生成论文摘要