DexDLO: Learning Goal-Conditioned Dexterous Policy for Dynamic Manipulation of Deformable Linear Objects
ICRA 2024(2024)
摘要
Deformable linear object (DLO) manipulation is needed in many fields. Previous research on deformable linear object (DLO) manipulation has primarily involved parallel jaw gripper manipulation with fixed grasping positions. However, the potential for dexterous manipulation of DLOs using an anthropomorphic hand is under-explored. We present DexDLO, a model-free framework that learns dexterous dynamic manipulation policies for deformable linear objects with a fixed-base dexterous hand in an end-to-end way. By abstracting several common DLO manipulation tasks into goal-conditioned tasks, DexDLO can perform tasks such as DLO grabbing, DLO pulling, DLO end-tip position controlling, etc. Using the Mujoco physics simulator, we demonstrate that our framework can efficiently and effectively learn five different DLO manipulation tasks with the same framework parameters. We further provide a thorough analysis of learned policies, reward functions, and reduced observations for a comprehensive understanding of the framework.
更多查看译文
关键词
Dexterous Manipulation,Reinforcement Learning
AI 理解论文
溯源树
样例
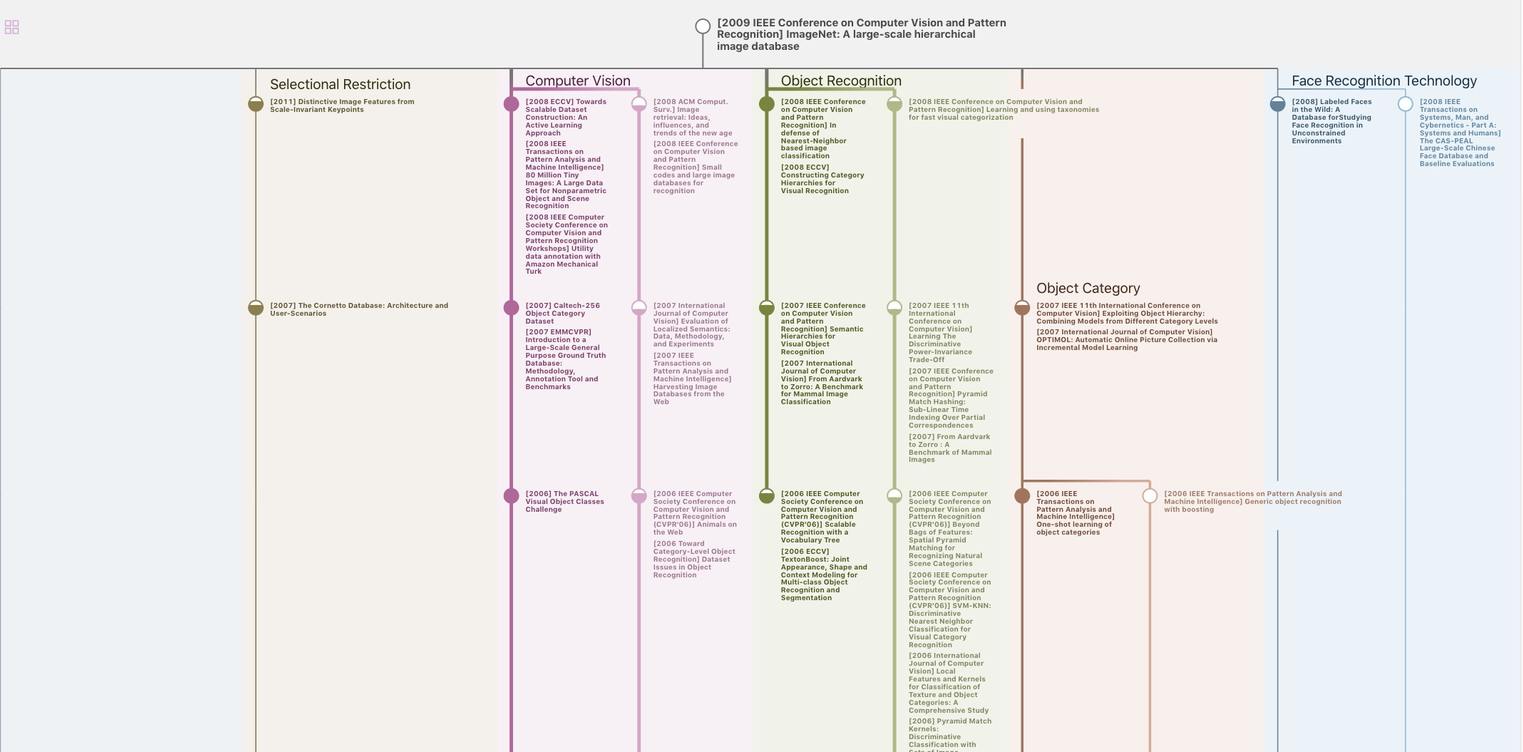
生成溯源树,研究论文发展脉络
Chat Paper
正在生成论文摘要