HyperMix: Out-of-Distribution Detection and Classification in Few-Shot Settings
CoRR(2023)
Abstract
Out-of-distribution (OOD) detection is an important topic for real-world machine learning systems, but settings with limited in-distribution samples have been underexplored. Such few-shot OOD settings are challenging, as models have scarce opportunities to learn the data distribution before being tasked with identifying OOD samples. Indeed, we demonstrate that recent state-of-the-art OOD methods fail to outperform simple baselines in the few-shot setting. We thus propose a hypernetwork framework called HyperMix, using Mixup on the generated classifier parameters, as well as a natural out-of-episode outlier exposure technique that does not require an additional outlier dataset. We conduct experiments on CIFAR-FS and MiniImageNet, significantly outperforming other OOD methods in the few-shot regime.
MoreTranslated text
Key words
Algorithms,Machine learning architectures,formulations,and algorithms
AI Read Science
Must-Reading Tree
Example
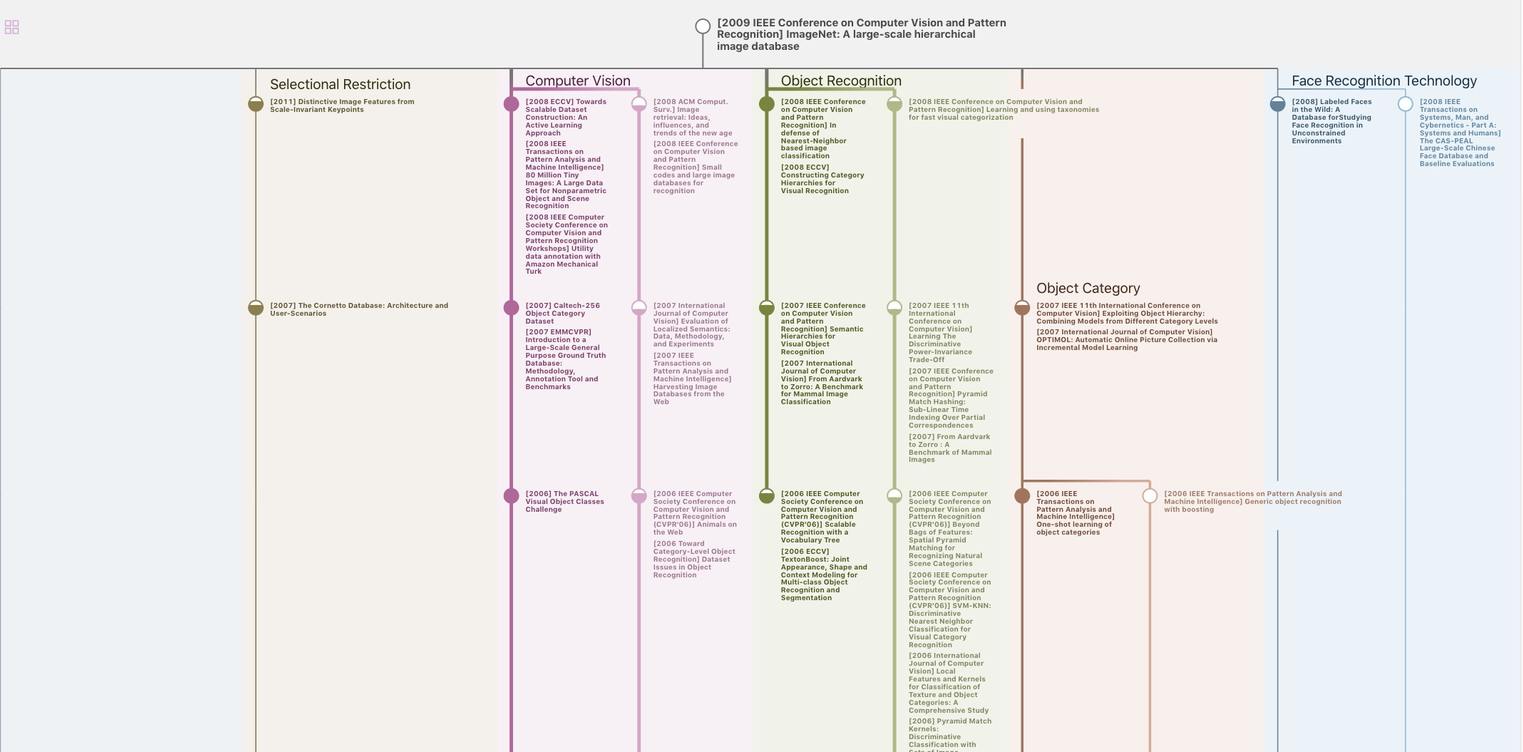
Generate MRT to find the research sequence of this paper
Chat Paper
Summary is being generated by the instructions you defined