Short-lived High-volume Multi-A(rmed)/B(andits) Testing
CoRR(2023)
摘要
Modern platforms leverage randomized experiments to make informed decisions from a given set of items (“treatments”). As a particularly challenging scenario, these items may (i) arrive in high volume, with thousands of new items being released per hour, and (ii) have short lifetime, say, due to the item's transient nature or underlying non-stationarity that impels the platform to perceive the same item as distinct copies over time. Thus motivated, we study a Bayesian multiple-play bandit problem that encapsulates the key features of the multivariate testing (or “multi-A/B testing”) problem with a high volume of short-lived arms. In each round, a set of k arms arrive, each available for w rounds. Without knowing the mean reward for each arm, the learner selects a multiset of n arms and immediately observes their realized rewards. We aim to minimize the loss due to not knowing the mean rewards, averaged over instances generated from a given prior distribution. We show that when k = O(n^ρ) for some constant ρ>0, our proposed policy has Õ(n^-min{ρ, 1/2 (1+1/w)^-1}) loss on a sufficiently large class of prior distributions. We complement this result by showing that every policy suffers Ω (n^-min{ρ, 1/2}) loss on the same class of distributions. We further validate the effectiveness of our policy through a large-scale field experiment on Glance, a content-card-serving platform that faces exactly the above challenge. A simple variant of our policy outperforms the platform's current recommender by 4.32% in total duration and 7.48% in total number of click-throughs.
更多查看译文
AI 理解论文
溯源树
样例
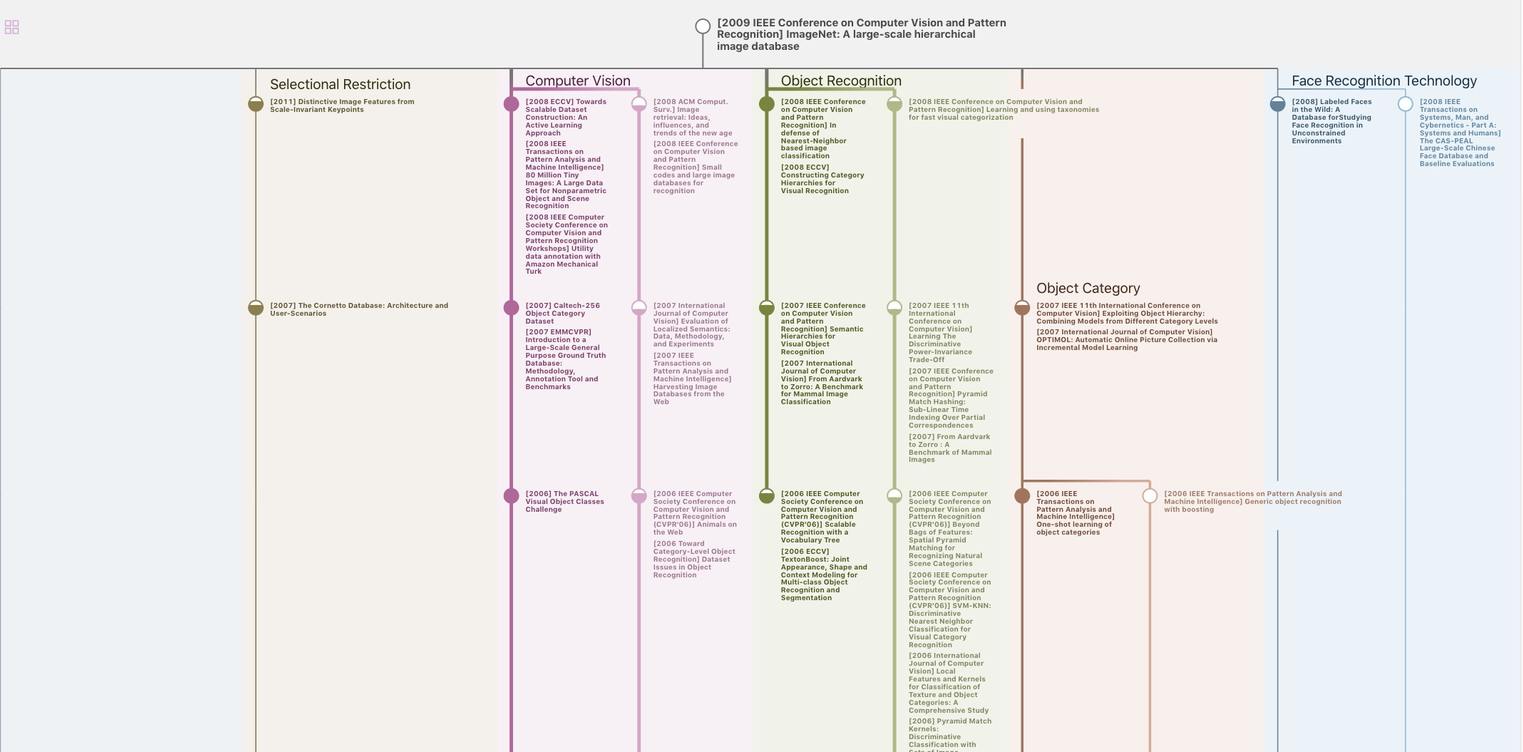
生成溯源树,研究论文发展脉络
Chat Paper
正在生成论文摘要