Unsupervised structural damage assessment method based on response correlations
ENGINEERING STRUCTURES(2024)
摘要
Structural damage assessment for civil engineering structures has become an important research topic and has gained much attention for decades in the field of structural health monitoring (SHM). Current unsupervised structural damage assessment methods can achieve damage detection for practical civil engineering structures. However, these methods cannot fully mine the response correlations among sensors, thus are difficult to localize damage in practical engineering applications. Therefore, this study proposes an unsupervised structural damage assessment method with bidirectional long short-term memory (BiLSTM) networks to establish the response correlations among sensors. Numerical studies of a beam-like bridge model, an experimental study of Yellow Frame, and an in-field study of Yonghe Bridge were conducted to validate the accuracy and reliability of the proposed approach. The results show that unsupervised structural damage identification and localization can be achieved even in the case of sparse sensor deployment due to the fact that the response correlations among sensors can be well extracted. The proposed approach can be applied to damage identification of practical engineering structures under operational and ambient excitations and can automatically provide damage location warnings to ensure structural health and prevent catastrophic events.
更多查看译文
关键词
Structural health monitoring,Unsupervised learning,Structural damage identification,Bidirectional long short-term memory networks,Statistical pattern recognition
AI 理解论文
溯源树
样例
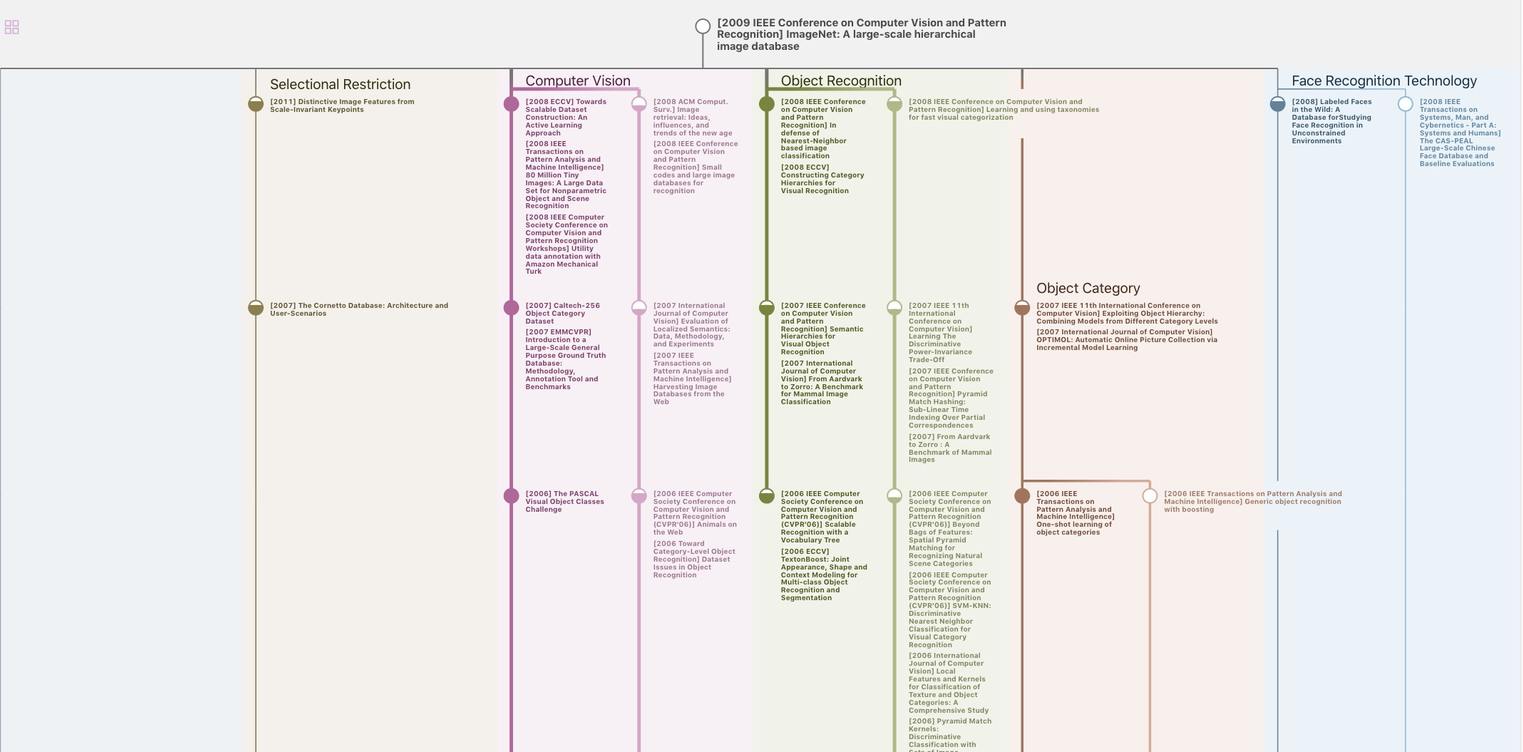
生成溯源树,研究论文发展脉络
Chat Paper
正在生成论文摘要