PDRLRR: A novel low-rank representation with projection distance regularization via manifold optimization for clustering
PATTERN RECOGNITION(2024)
摘要
The low-rank representation (LRR) method has attracted widespread attention due to its excellent performance in pattern recognition and machine learning. LRR-based variants have been proposed to solve the three existing problems in LRR: (1) the projection matrix is permanently fixed when dimensionality reduction techniques are adopted; (2) LRR fails to capture the local geometric structure; and (3) the solution deviates from the real low-rank solution. To address these problems, this paper proposes a low-rank representation with projection distance regularization (PDRLRR) via manifold optimization for clustering. In detail, we first introduce a low-dimensional projection matrix and a projection distance regularization term to fit the projected data automatically and capture the local structure of the data, respectively. Consequently, the projection matrix and representation matrix are obtained jointly. Then, we obtain a more accurate low-rank solution by minimizing the Schatten-p norm instead of the nuclear norm. Next, the projection matrix is optimized through a generalized Stiefel manifold. Extensive experiments demonstrate that our proposed method outperforms the state-of-the-art methods.
更多查看译文
关键词
Low-rank representation,Schatten-p norm,Projection distance regularization,Manifold optimization,Data clustering
AI 理解论文
溯源树
样例
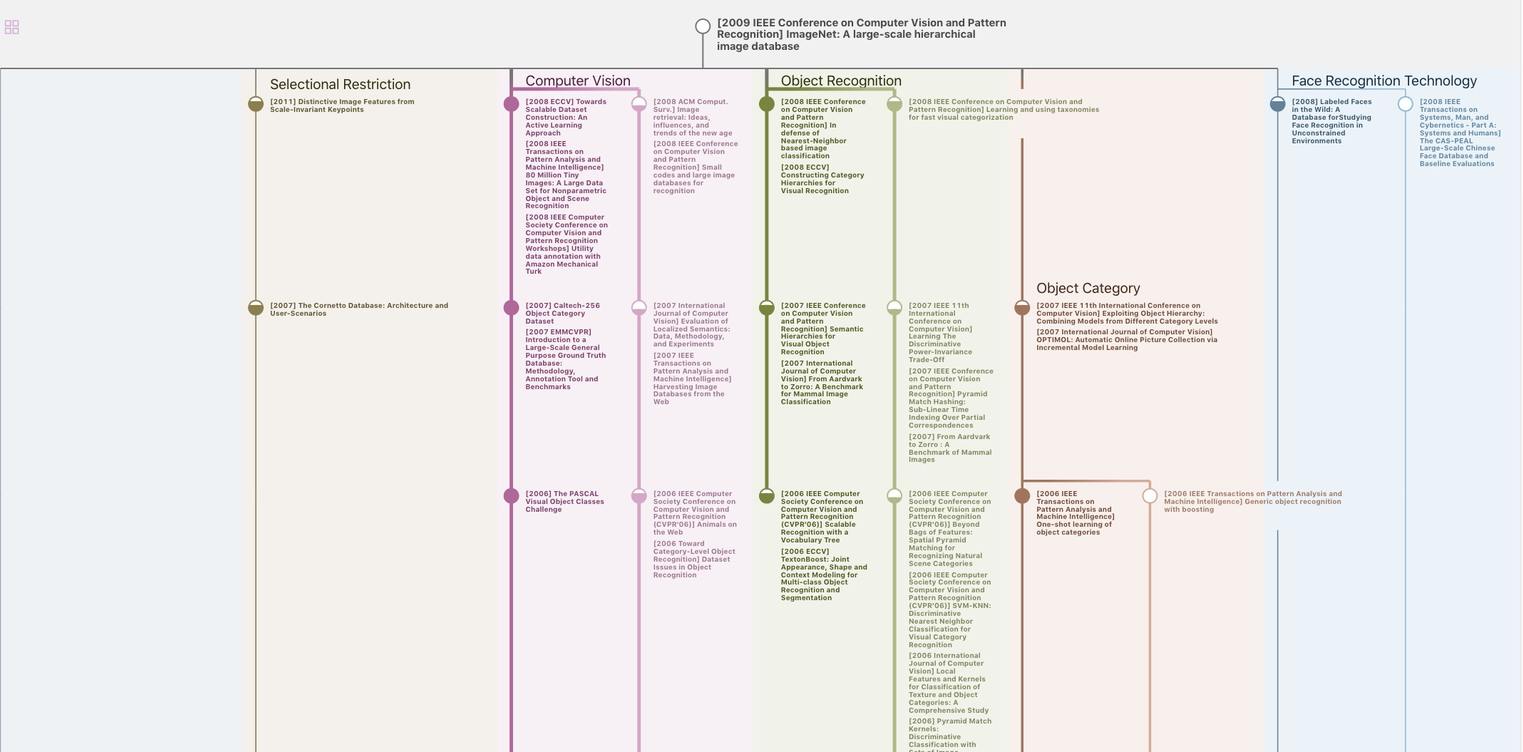
生成溯源树,研究论文发展脉络
Chat Paper
正在生成论文摘要