Combining physical mechanisms and deep learning models for hourly surface ozone retrieval in China
JOURNAL OF ENVIRONMENTAL MANAGEMENT(2024)
摘要
As surface ozone (O3) gains increasing attention, there is an urgent need for high temporal resolution and accurate O3 monitoring. By taking advantage of the progress in artificial intelligence, deep learning models have been applied to satellite based O3 retrieval. However, the underlying physical mechanisms that influence surface O3 into model construction have rarely been considered. To overcome this issue, we considered the physical mechanisms influencing surface O3 and used them to select relevant variable features for developing a novel deep learning model. We used a wide and deep model architecture to account for linear and non-linear relationships between the variables and surface O3. Using the developed model, we performed hourly inversions of surface O3 retrieval over China from 2017 to 2019 (9:00-17:00, local time). The validation results based on sample-based (site-based) methods yielded an R2 of 0.94 (0.86) and an RMSE of 12.79 (19.13) mu g/m3, indicating the accuracy of the models. The average surface O3 concentrations in China in 2017, 2018, and 2019 were 82, 78, and 87 mu g/ m3, respectively. There was a diurnal pattern in surface O3 in China, with levels rising significantly from 55 mu g/ m3 at 9:00 a.m. to 96 mu g/m3 at 15:00. Between 15:00 and 16:00, the O3 concentration remained stable at 95 mu g/ m3 and decreased slightly thereafter (16:00-17:00). The results of this study contribute to a deeper understanding of the physical mechanisms of ozone and facilitate further studies on ozone monitoring, thereby enhancing our understanding of the spatiotemporal characteristics of ozone.
更多查看译文
AI 理解论文
溯源树
样例
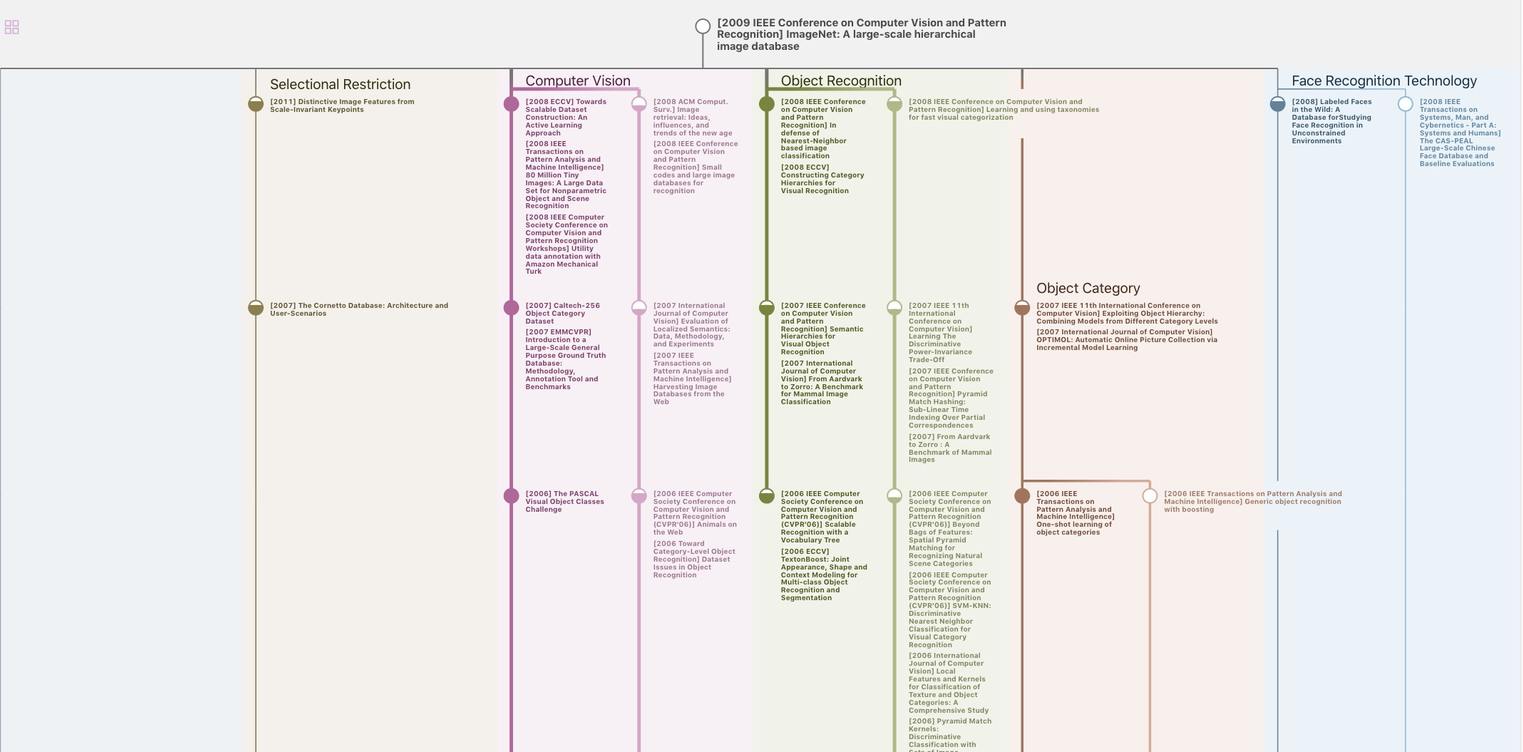
生成溯源树,研究论文发展脉络
Chat Paper
正在生成论文摘要