Two-Stream Architecture Using RGB-based ConvNet and Pose-based LSTM for Video Action Recognition.
2023 15th International Conference on Innovations in Information Technology (IIT)(2023)
摘要
Traditional methods for video recognition require hand-crafted features, which often involves offline pre-processing for real-world videos. In this study, we propose a conceptually simple framework that directly takes raw videos as an input source for activity recognition. Our framework consists of two streams, namely a spatial stream and a temporal stream. The spatial stream is trained on RepVGG-B0 ConvNet using cropped RGB features, while the temporal stream uses an attention-based Bi-directional Long Short-Term Memory (Bi-LSTM) network to learn posture vectors from human pose data obtained through Faster R-CNN pre-trained model. Our proposed method is evaluated on a standard video action recognition benchmark, MSR Daily Activity3D, and proves to be competitive with state-of-the-art action recognition methods. We achieve state-of-the-art performance on MSR Daily Activity3D with a precision and recall rate of 99.01% and 98.91%, respectively. Our results demonstrate the effectiveness of our approach in recognizing video actions.
更多查看译文
关键词
video action recognition,Faster R-CNN,deep learning,attention-based Bi-directional LSTM,RepVGG,RGB activity images
AI 理解论文
溯源树
样例
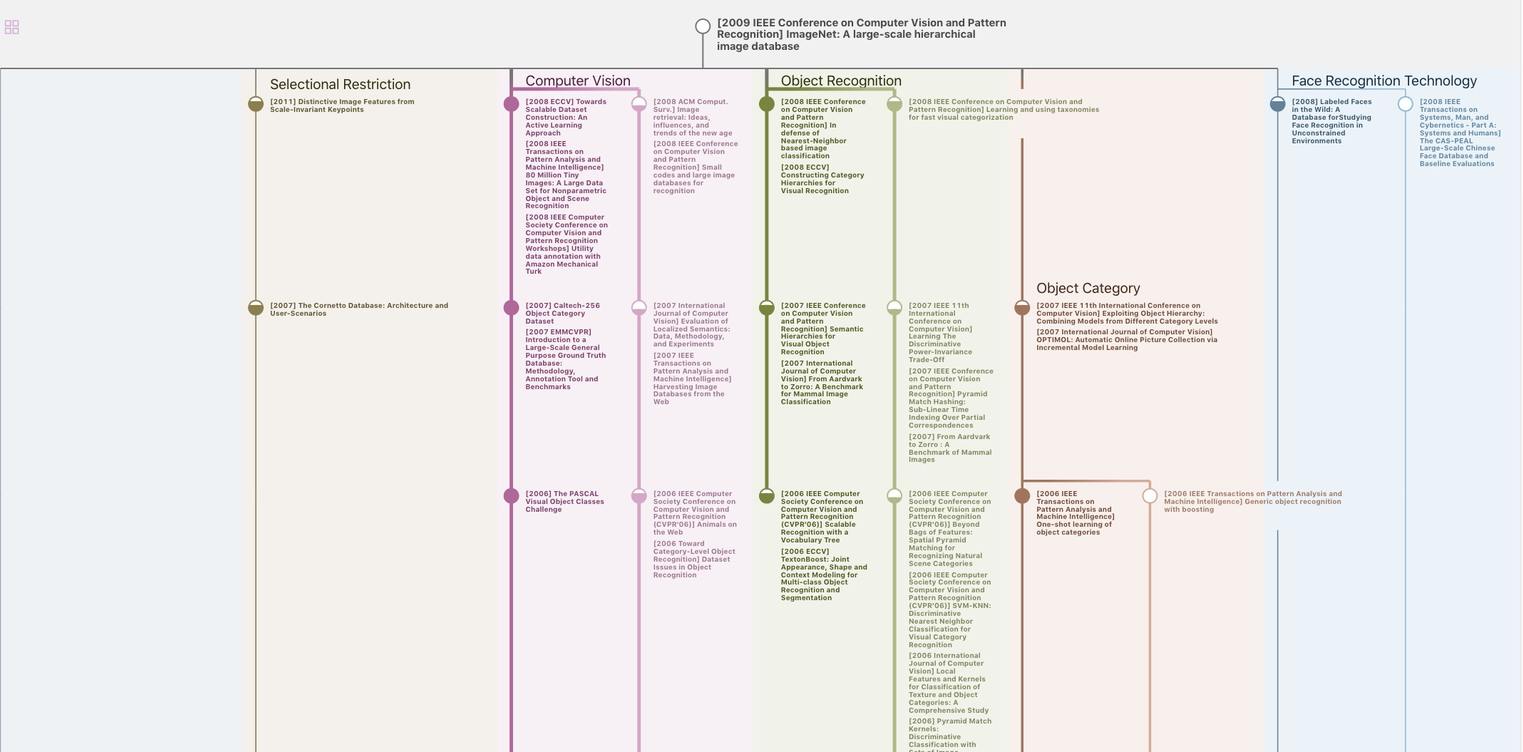
生成溯源树,研究论文发展脉络
Chat Paper
正在生成论文摘要