Zero-Shot Dynamic Neural Network Adaptation in Tactical Wireless Systems
MILCOM 2023 - 2023 IEEE MILITARY COMMUNICATIONS CONFERENCE(2023)
摘要
Models based on deep neural networks have attracted interest in a myriad of applications in the wireless landscape, including spectrum intelligence, modulation recognition, and radio fingerprinting, among others. One key challenge that is currently inhibiting the application of such models in real-world tactical scenarios is that their performance radically changes with continuously changing dynamic wireless channel conditions. Existing work tackle this by performing efficient fine-tuning or adaptation using as few samples as possible. However, some prior knowledge about the new conditions is involved in the process. In this paper, we propose for the first time zero-shot dynamic neural network adaptation (zDNA) (i.e., without any additional training samples) of wireless classification models after deployment in unseen conditions. Specifically, we show that by changing only the affine transformation parameters and normalizing the learned features in different layers of the neural network in an online manner without any labeled samples, we can achieve superior performance in dynamic conditions. Our proposed approach is evaluated on the publicly available RadioML 2018.01A dataset, to test its adaptability to dynamically changing signal-to-noise ratio (SNR) conditions. Performance improvement consistency in all unseen test scenarios (up to 24% in low SNR regime) demonstrates the applicability of our framework in real-world contexts.
更多查看译文
AI 理解论文
溯源树
样例
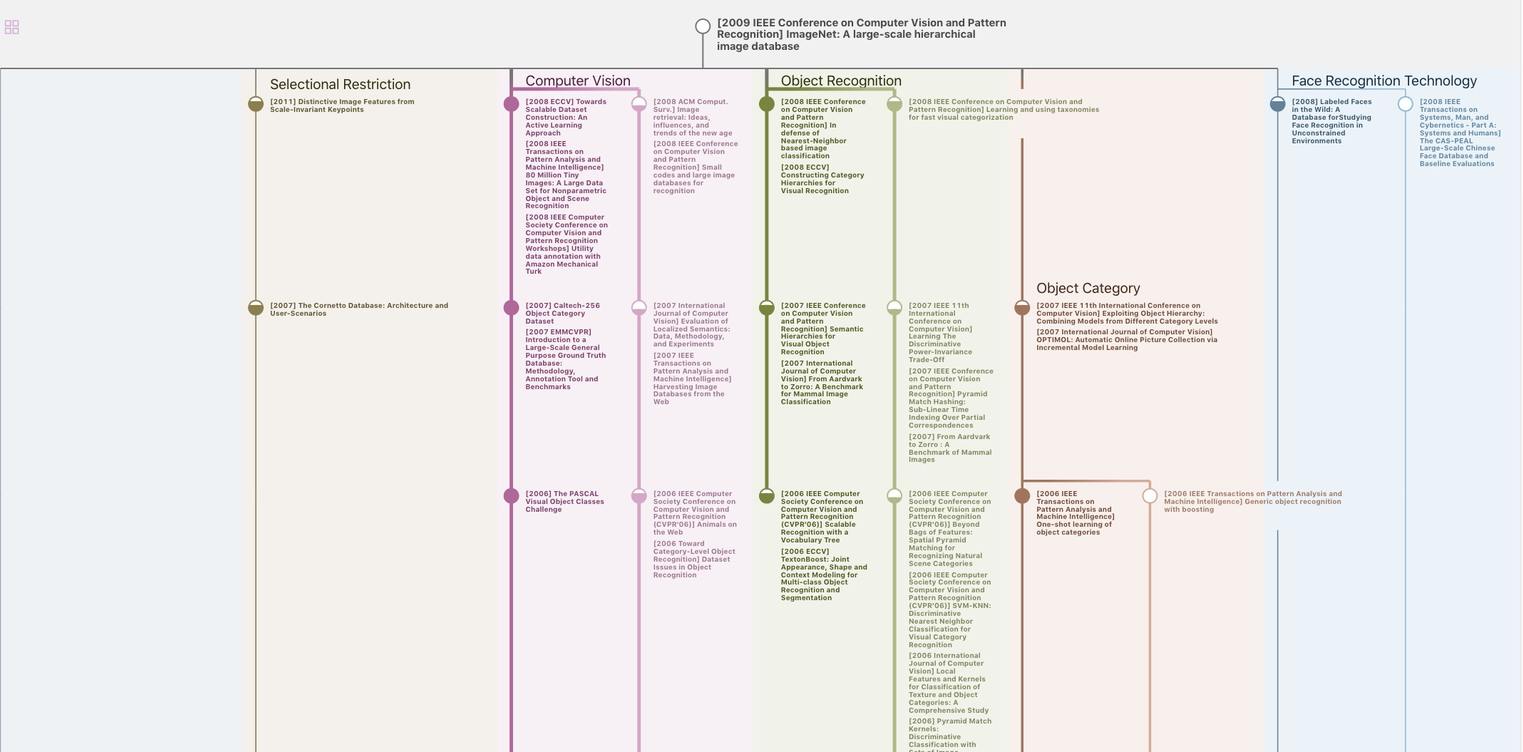
生成溯源树,研究论文发展脉络
Chat Paper
正在生成论文摘要