Data-Driven Constraint Mining for Realizable Adversarial Samples
MILCOM 2023 - 2023 IEEE MILITARY COMMUNICATIONS CONFERENCE(2023)
摘要
Machine learning based malware detectors can identify new patterns in malware not considered by classic signature matching antivirus software, by processing features extracted from cyber artifacts. However, they must be evaluated for robustness against adversarial attacks that cause mis-classifications by making small modifications to the feature values to exploit weaknesses in the machine learning model's decision surface. The process of feature extraction maps the problem space, or the actual cyber artifact (e.g. file, network flow data), to the feature space, often resulting in dependencies between features. To learn adversarial examples for malware detectors that can be realized in the real world, we need to be able to map feature-value perturbations back to the original problem space. This requires that 1) all feature value changes are consistent with dependencies between features that are inherent in the mapping from the problem space 2) the feature values are realizable in the problem space. While most current methods require expert knowledge to identify these constraints on the feature values, data-driven constraint mining is an automatic and generalized alternative. This work identifies Tabular Constraint Learning (TaCLe) as a method to automatically mine the dependencies between features via analysis of the feature space data. TaCLe is incorporated into a constraint aware adversarial attack strategy to identify the watermark selection space for a backdoor poisoning attack. We find that it enables the attack to find watermarks that are 70% more evasive than the state-of-the-art baseline.
更多查看译文
关键词
adversarial learning,cyber-security,constraint learning,malware detection
AI 理解论文
溯源树
样例
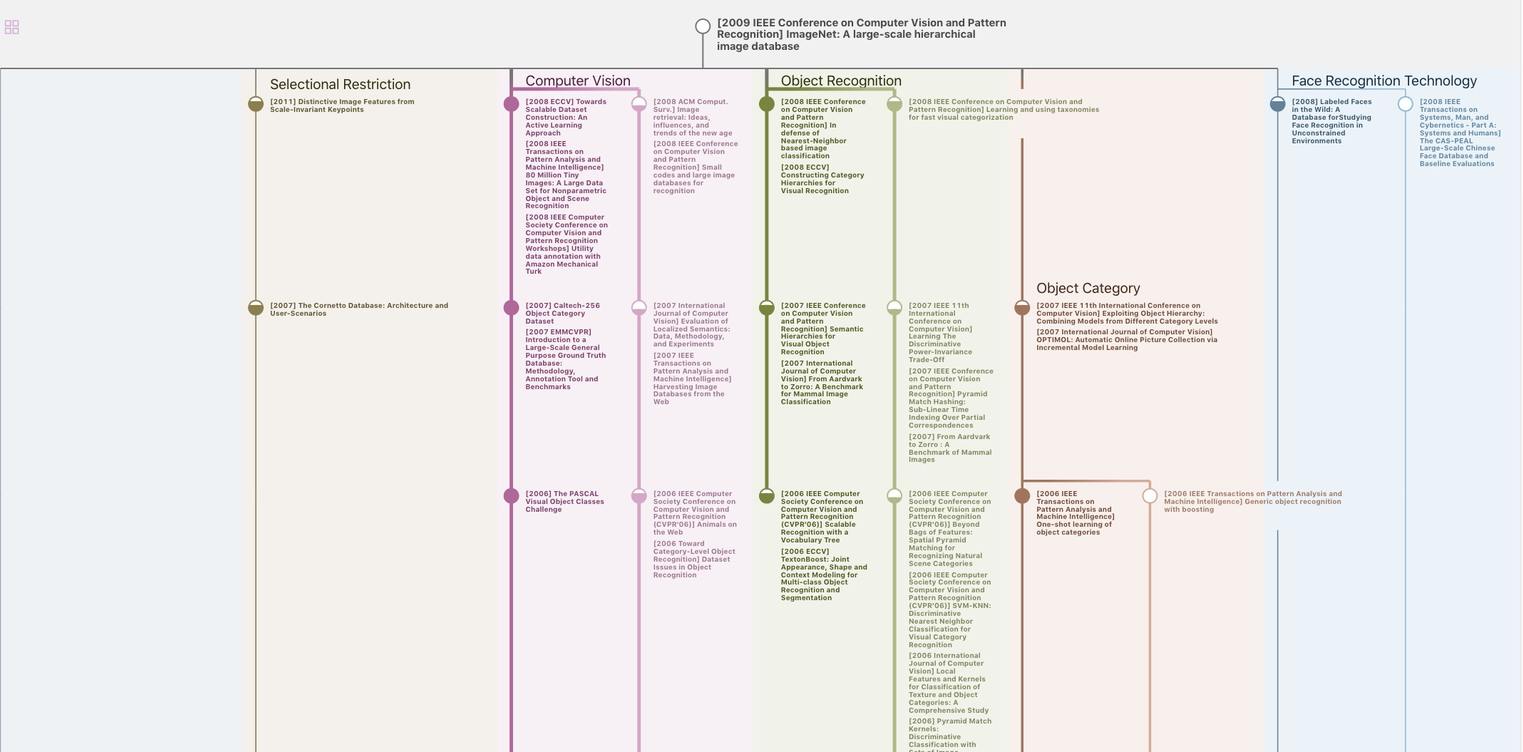
生成溯源树,研究论文发展脉络
Chat Paper
正在生成论文摘要