Characterizing Distributed Inferencing at the Edge in Resource-Constrained Environments
MILCOM 2023 - 2023 IEEE MILITARY COMMUNICATIONS CONFERENCE(2023)
摘要
There are a growing number of low compute devices gathering data in network constrained environments at the edge. There are several existing solutions which have attempted to solve the limits of computation at the edge, including local computation, cloud offloading, data distributed and model distributed inferencing. While each of these inferencing types have their own benefits and drawbacks, this work seeks to evaluate which performs best in a resource constrained environment. Model distributed inferencing (MDI) is a technique recently developed to help bridge this gap by allowing models to be divided into individual layers and run across multiple worker nodes. This allows for a limited amount of data to be sent between nodes in the form of activation vectors, without the need to offload the full dataset to either the cloud or other distributed devices. To conduct these experiments a testbed has been created allowing for various devices to be defined each with various level of compute and memory capability. In addition, the network within this testbed is controllable allowing for the bandwidth between devices to be defined for evaluating performance in a bandwidth constrained environment with heterogeneous devices. Results highlight the performance benefits of both model distributed inferencing in relation to computation limitations, but also in relation to network bandwidth limitations.
更多查看译文
关键词
Machine learning,Edge Computing
AI 理解论文
溯源树
样例
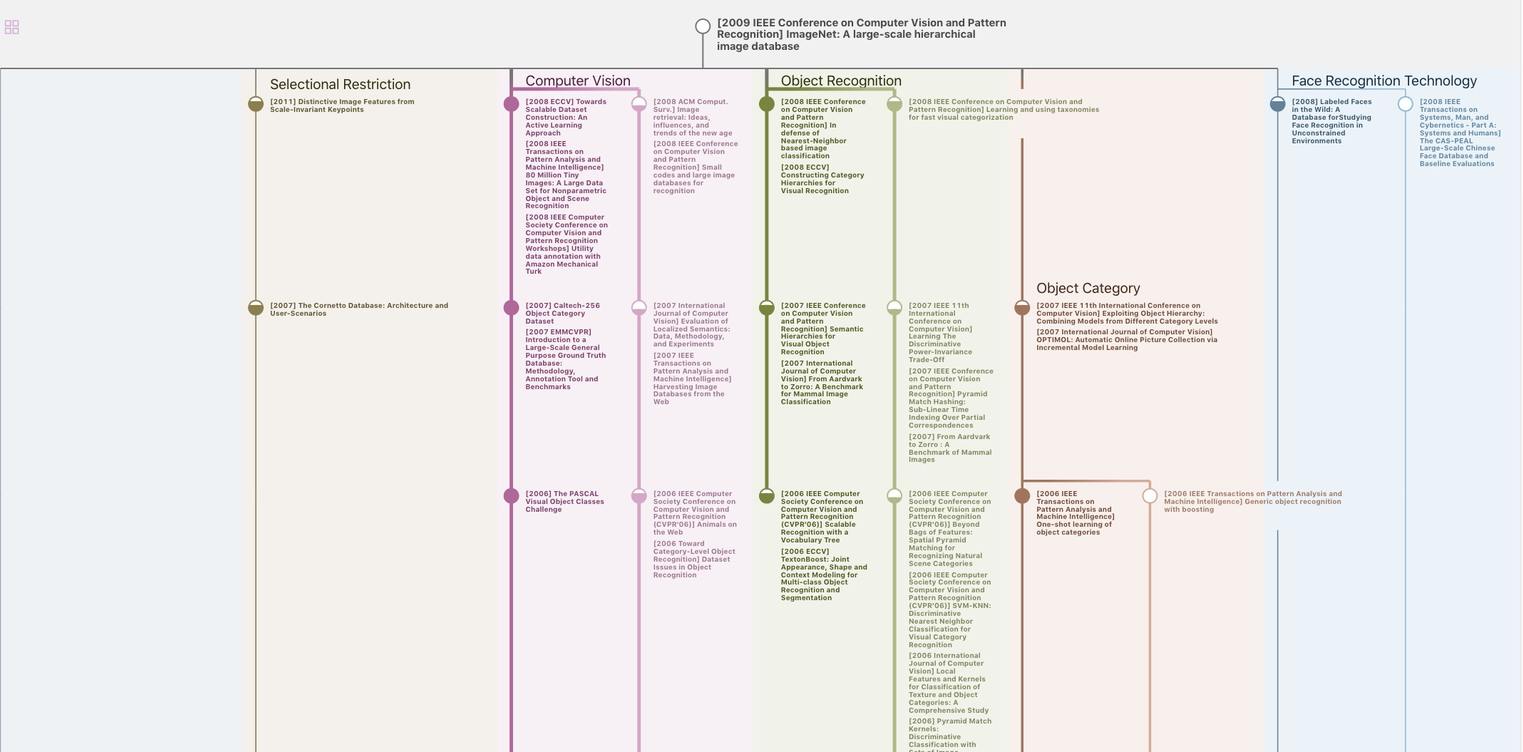
生成溯源树,研究论文发展脉络
Chat Paper
正在生成论文摘要