Channel-Adaptive Dynamic Neural Networks for Low-Complexity Distributed Signal Awareness
MILCOM 2023 - 2023 IEEE MILITARY COMMUNICATIONS CONFERENCE(2023)
摘要
Deep neural networks (DNNs) are being increasingly used to achieve signal awareness. Usually, DNNs have been entirely executed at the mobile device. However, as DNNs become more complex, edge computing techniques will become necessary for DNN deployment. Notably, it has been shown that the original DNN architectures can be modified by introducing a "bottleneck" producing a compressed representation of the input. The DNN split computation is then orchestrated between the mobile device and the edge, which respectively execute the layers before and after the bottleneck. As yet, the concept of split computing has not been applied for signal awareness. Moreover, in existing work, the dynamic nature of the wireless channel is not considered in the bottleneck design. In this paper, we propose Split Computing Enhancing Signal Awareness (SpliCES), a new approach where the bottleneck is adapted at inference time according to ongoing channel conditions. Moreover, we present a novel training strategy which optimizes the bottleneck-injected ensemble parameters to generalize to all channel conditions in its first stage, and then specializes each bottleneck separately to a certain level of channel impairment in the second stage. We evaluate SpliCES on the well-known RadioML dataset for modulation recognition, and show that on average, our proposed method transmits 12x less data while experiencing only about 3% accuracy drop in high signal-to-noise ratio (SNR) regimes. With low SNR, SpliCES achieves 5% better accuracy thanks to its dynamic structure.
更多查看译文
AI 理解论文
溯源树
样例
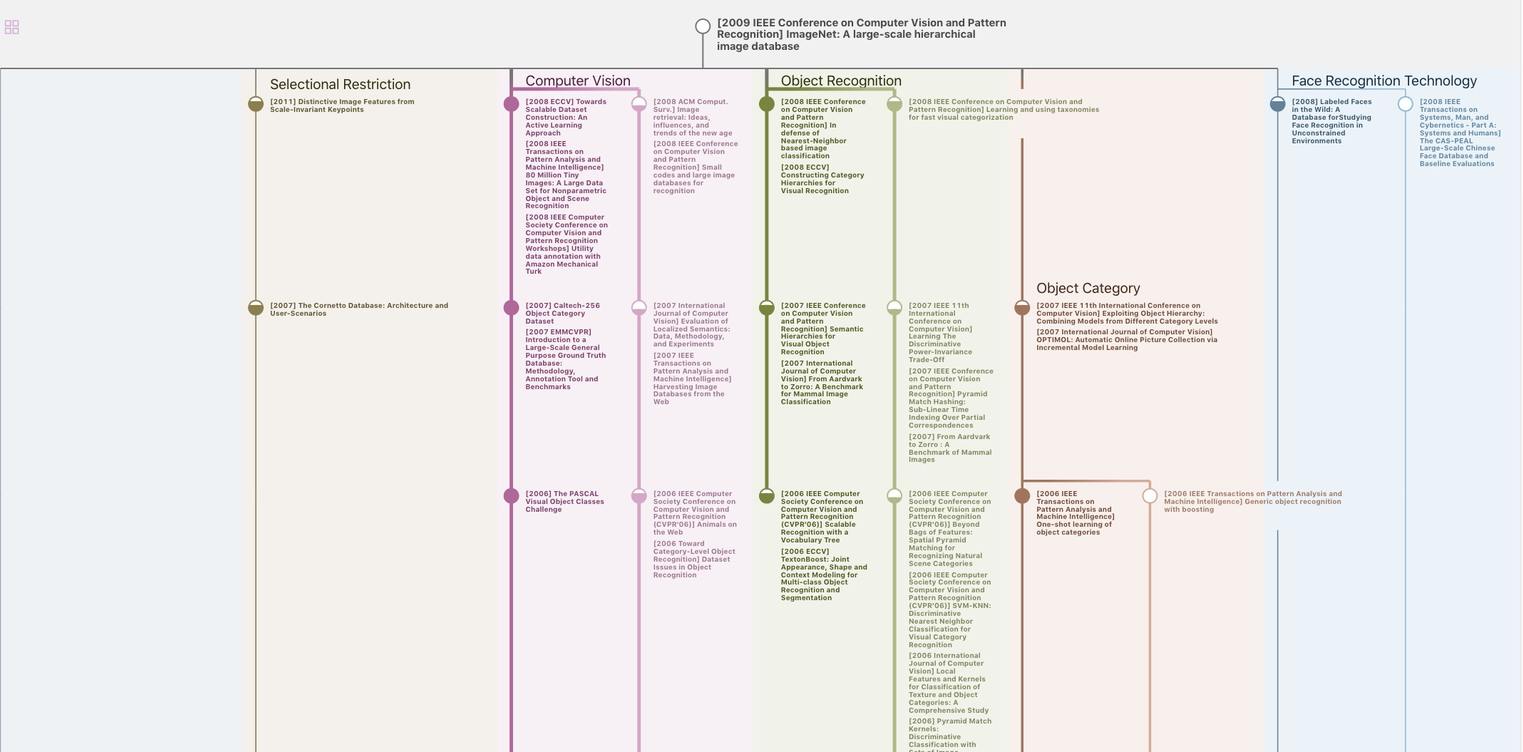
生成溯源树,研究论文发展脉络
Chat Paper
正在生成论文摘要