Scalable Deep Learning for Pilot Performance Analysis Using Multimodal Physiological Time Series
2023 IEEE HIGH PERFORMANCE EXTREME COMPUTING CONFERENCE, HPEC(2023)
摘要
Sensors used to collect human physiological data often necessitate the processing and classification of time series data, which can quickly become intractable with very lengthy inputs or many time series features. In this study we compared the performance of two methods of time series feature extraction and dimensionality reduction, Minimally Random Convolutional Kernel Transform (MiniRocket) and statistical feature engineering using TSFresh, to determine the optimal hardware configurations and associated performance-accuracy trade-offs between model speed and complexity. Our results showed that MiniRocket scales extremely well with only linear complexity while the scaling of TSFresh is dependent on the set of features selected for computation. Further, MiniRocket outperformed the TSFresh model accuracy for all configurations except the most comprehensive (but slowest) feature extraction set thereby highlighting MiniRocket as a great all-purpose dimensionality reduction tool for human physiological time series data.
更多查看译文
关键词
Artifical Intelligence,dimentionality reduction,Multimodality,MiniRocket,Physiology,Scalability,TSFresh
AI 理解论文
溯源树
样例
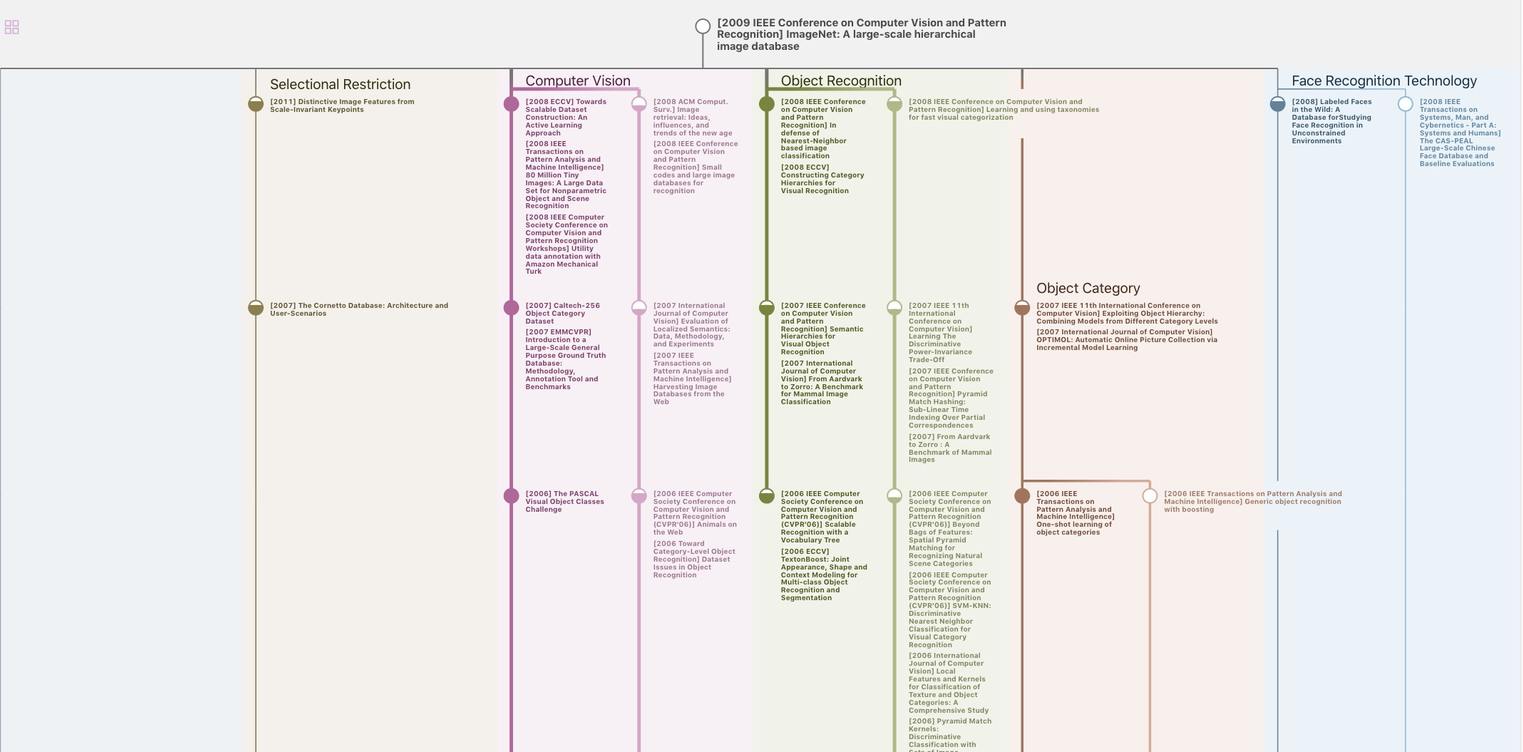
生成溯源树,研究论文发展脉络
Chat Paper
正在生成论文摘要