Solving Sparse Linear Systems via Flexible GMRES with In-Memory Analog Preconditioning
2023 IEEE HIGH PERFORMANCE EXTREME COMPUTING CONFERENCE, HPEC(2023)
摘要
Analog arrays of non-volatile crossbars leverage physics to compute approximate matrix-vector multiplications in a rapid, in-memory fashion. In this paper we consider exploiting this technology to precondition the Generalized Minimum Residual iterative solver (GMRES). Since the preconditioner must be applied through matrix-vector multiplication, approximate inverse preconditioners are a natural fit. At the same time, the errors introduced by the analog hardware render an iteration matrix that changes from one iteration to another. To remedy this, we propose to combine analog approximate inverse preconditioning with a flexible GMRES algorithm that naturally incorporates variations of the preconditioner into its model. The benefit of our approach is that the analog circuit is much simpler than correcting the errors at the hardware level. Our experiments with a simulator for analog hardware show that such an analogflexible scheme can lead to fast convergence.
更多查看译文
关键词
Analog hardware,preconditioning,flexible GM-RES,sparse linear systems
AI 理解论文
溯源树
样例
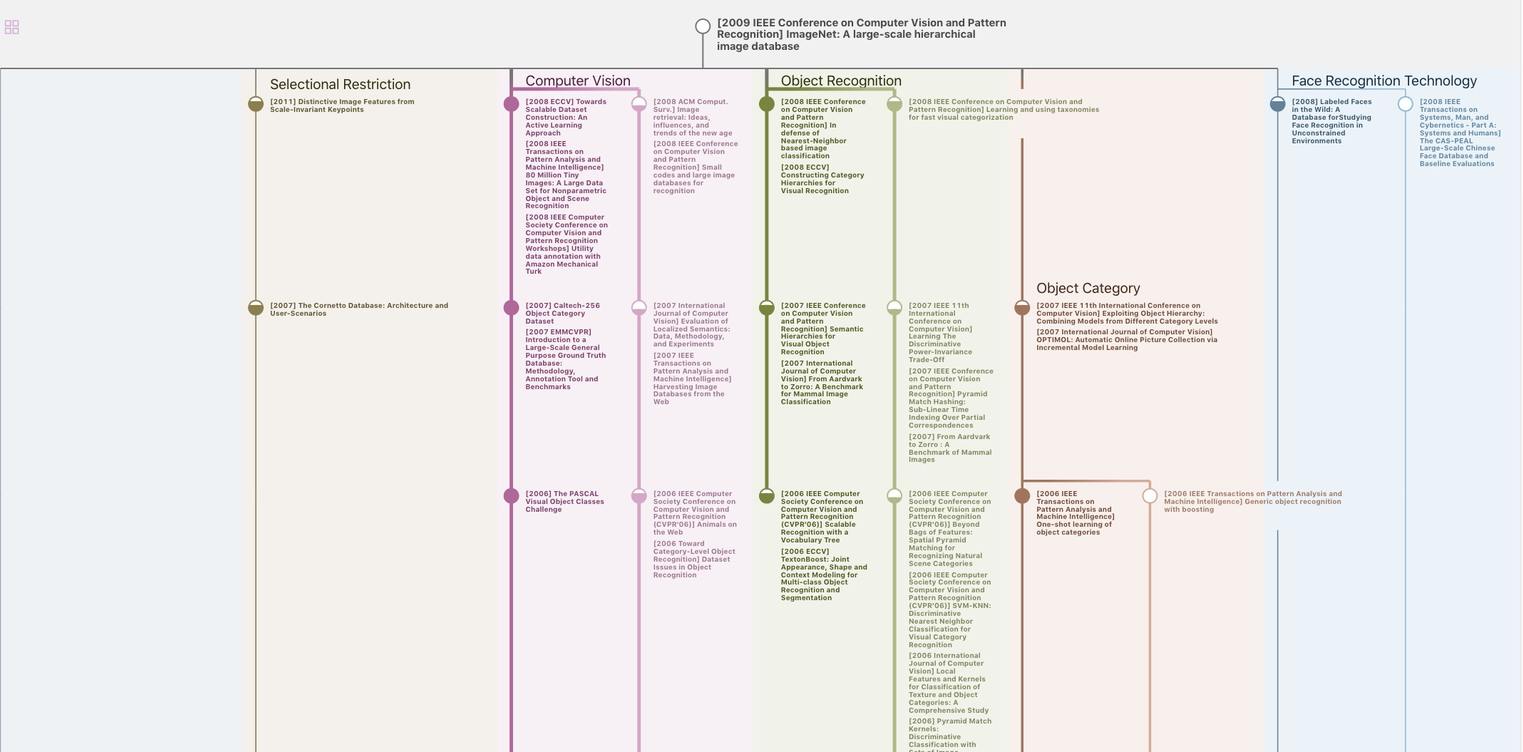
生成溯源树,研究论文发展脉络
Chat Paper
正在生成论文摘要