A Comparative Analysis of Machine Learning Algorithms for Credit Risk Scoring using Chi-Square Feature Selection
2023 International Conference on Converging Technology in Electrical and Information Engineering (ICCTEIE)(2023)
Abstract
Credit payment, which involves making predetermined installment amounts starting from the receipt of the purchased product, has become increasingly popular. Lending institutions often employ various risk assessment strategies, commonly known as credit risk scoring. Manual credit risk analysis often requires a longer processing time and may result in a decrease in accuracy. By leveraging the advancements in artificial intelligence, the current landscape offers promising opportunities for conducting credit risk analysis in a more efficient and accurate manner. The primary objective of this paper is to utilize the Chi-Square feature selection method in order to improve the speed and efficiency of these algorithms. Four machine learning algorithms are used to assess the outcomes. The 22 features have been carefully selected for further use and thoroughly evaluated. The final results indicate that the Naive Bayes Classifier technique, with a score of only 0.65, is less accurate when compared to other models. In the realm of predicting whether a client will default or not, the Random Forest Classifier model emerges as the most effective model. This model boasts the highest accuracy value while maintaining the evaluation value at 0.68, making it the top choice among all the models. The deployment of the model reveals that there has been a decrease of 10.22% in the percentage of LGD.
MoreTranslated text
AI Read Science
Must-Reading Tree
Example
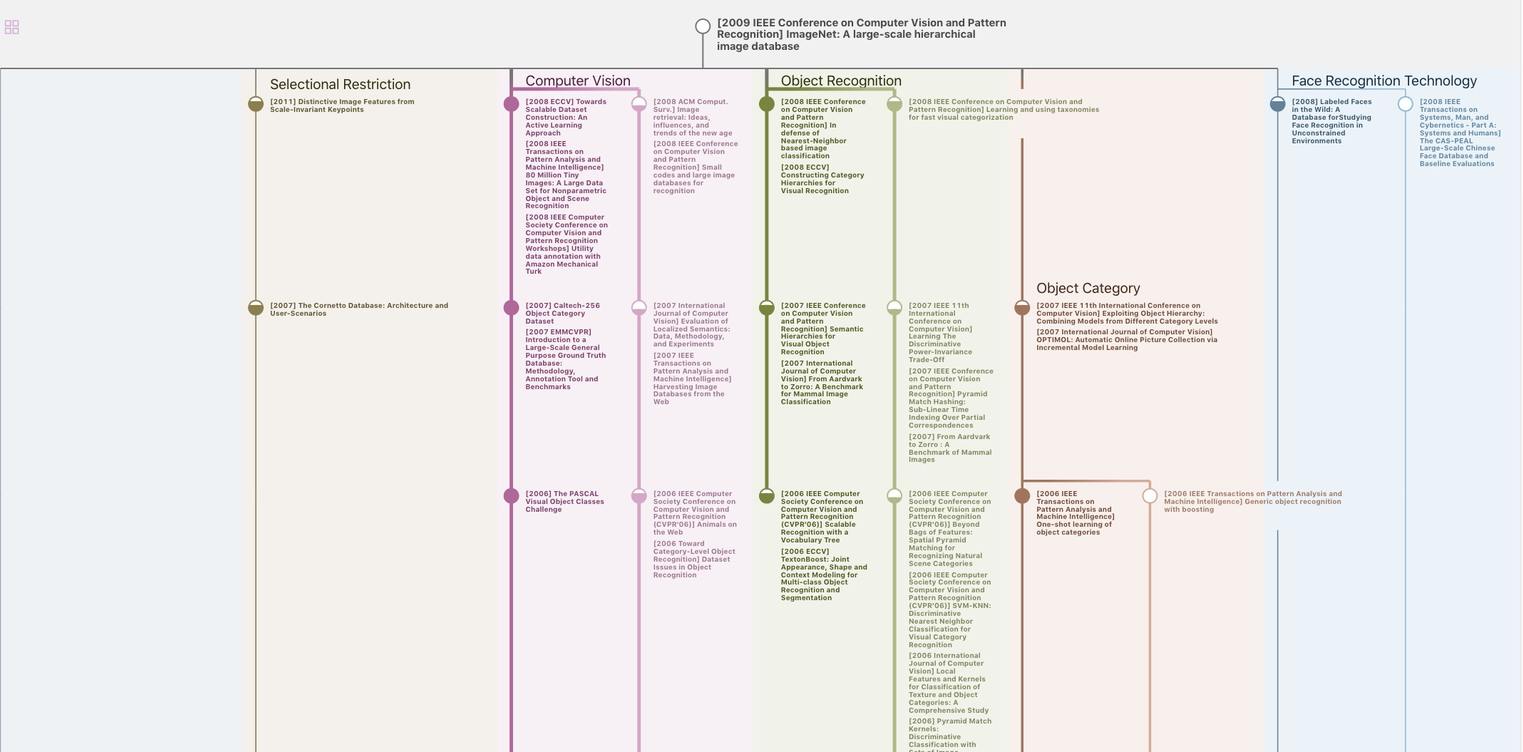
Generate MRT to find the research sequence of this paper
Chat Paper
Summary is being generated by the instructions you defined