Combined Deterministic and Stochastic Streams for Visual Prediction using Predictive Coding
2023 IEEE INTERNATIONAL CONFERENCE ON DEVELOPMENT AND LEARNING, ICDL(2023)
Abstract
We present a hybrid predictive coding framework designed for the prediction of future video frames. This model draws its conceptual foundation inspired from the predictive coding theories within the realm of cognitive science. The framework is imbued with a novel amalgamation of bottom-up and top-down information flows, fostering heightened interconnectivity among diverse tiers between prediction and reality. Notably, conventional predictive coding models primarily entail hierarchical event anticipation rather than prospective prediction. To address this limitation, our proposed model adopts a multi-scale paradigm, characterized by a Coarse-to-Fine schema. In relation to the network architecture, we integrate the encoder-decoder network within the Long Short-Term Memory (LSTM) module. This integration facilitates the sharing of ultimate encoded high-level semantic insights across varying strata of the neural network. Consequently, a profound interplay is established between the prevailing input and the historical LSTM states. This stands in stark contrast to the conventional Encoder-LSTM-Decoder configuration. The outcome is an erudite grasp of temporal and spatial dependencies, thereby engendering more verisimilar predictions. Empirical evaluations of our approach on benchmark datasets KTH.
MoreTranslated text
Key words
Predictive Coding,Visual Prediction,Cognitive vision
AI Read Science
Must-Reading Tree
Example
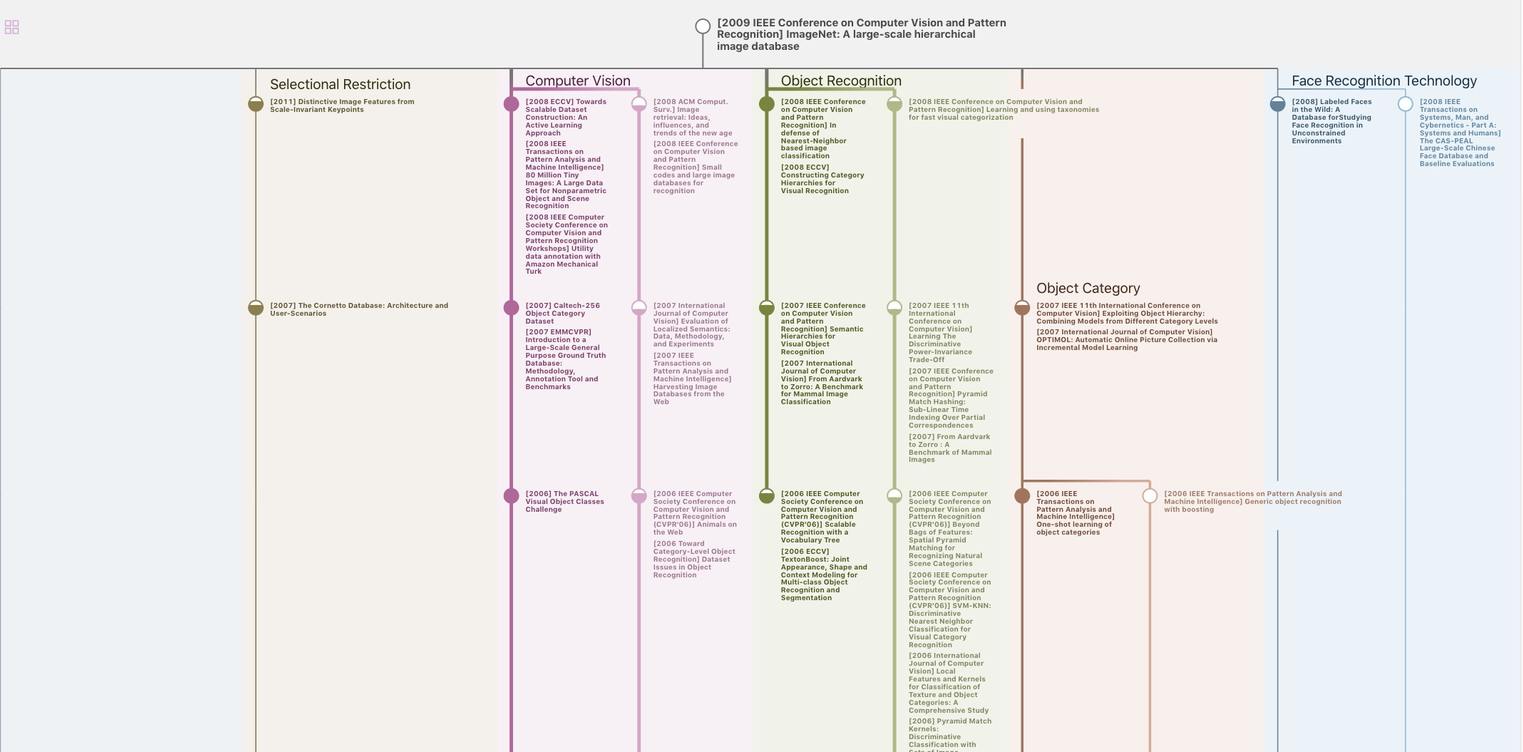
Generate MRT to find the research sequence of this paper
Chat Paper
Summary is being generated by the instructions you defined