Transformer with Time Vector Representation for Remaining Useful Life Prediction of Lithium-Ion Batteries
2023 International Conference on Radar, Antenna, Microwave, Electronics, and Telecommunications (ICRAMET)(2023)
Abstract
Lithium-ion batteries are arguably one of the most widely used storage devices for many applications. Unfortunately, these batteries experience a decline in performance over time due to usage, thereby reducing capacity and power. Their safety may be compromised when used with a capacity lower than a certain threshold (70 to 80%). Therefore, monitoring their capacity is necessary to be carried out. In recent years, the adoption of deep learning technologies has become more dominant for predicting battery capacity. In this study, in addition to using deep learning for prediction models, time vector representation (Time2Vec) is employed to find vector representation of time series data. Then, the Transformer is used as a prediction model. The experiments were conducted using the lithium-ion batteries dataset of NASA. The proposed method improves the performance compared to LSTM, a well-known deep learning method for time series prediction. The results found that using Time2Vec significantly improves the performance of the system.
MoreTranslated text
Key words
Remaining useful life (RUL),battery,Transformer,time representation,LSTM,neural network
AI Read Science
Must-Reading Tree
Example
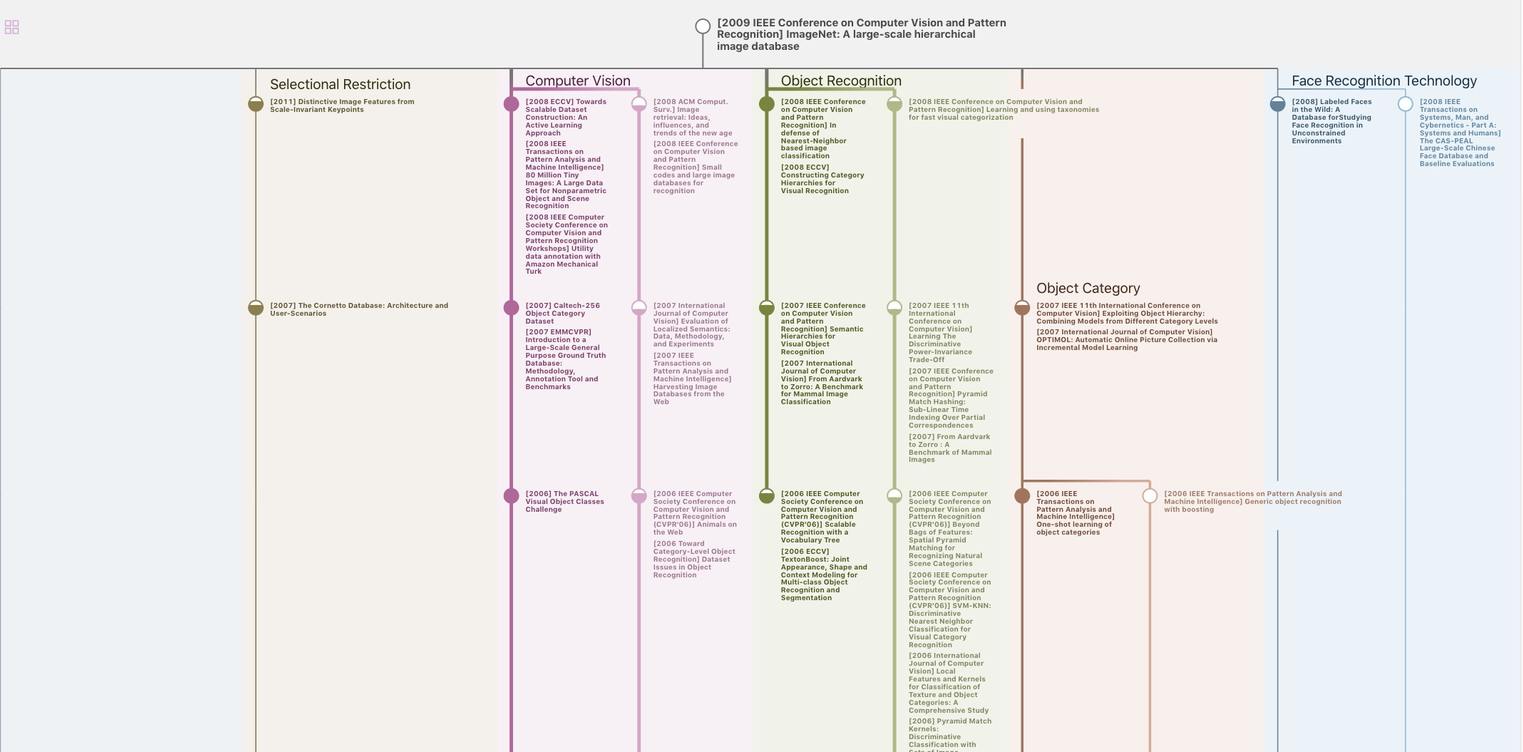
Generate MRT to find the research sequence of this paper
Chat Paper
Summary is being generated by the instructions you defined