Knowledge graph-guided object detection with semantic distance network
ELECTRONICS LETTERS(2023)
摘要
In this research study, the inadequacies of current object detection techniques are analyzed. These techniques solely recognize individual objects without considering their interrelationships. To address this issue, a novel solution called the knowledge graph-guided semantic distance network (KGSDN) approach is proposed. By utilizing a knowledge graph, KGSDN provides semantic contextual cues, leading to enhanced object detection accuracy. The KGSDN framework seamlessly integrates the knowledge graph and object detection network and employs an attention-based network to evaluate the semantic distance between objects. As a result, the conditional object probability of every bounding box is updated, and the joint probability of all objects in the image is determined. The empirical findings indicate that this approach significantly improves the performance of deep learning-based object detection methods. This paper introduces the knowledge graph-guided semantic distance network (KGSDN), a novel approach to object detection that leverages a knowledge graph to capture semantic relationships between objects. By integrating this knowledge graph into the object detection network and using an attention-based mechanism to measure semantic distances, KGSDN significantly improves object detection accuracy compared to existing methods. Experimental results demonstrate its effectiveness in enhancing deep learning-based object detection performance.image
更多查看译文
关键词
computer vision,knowledge graph,object detection
AI 理解论文
溯源树
样例
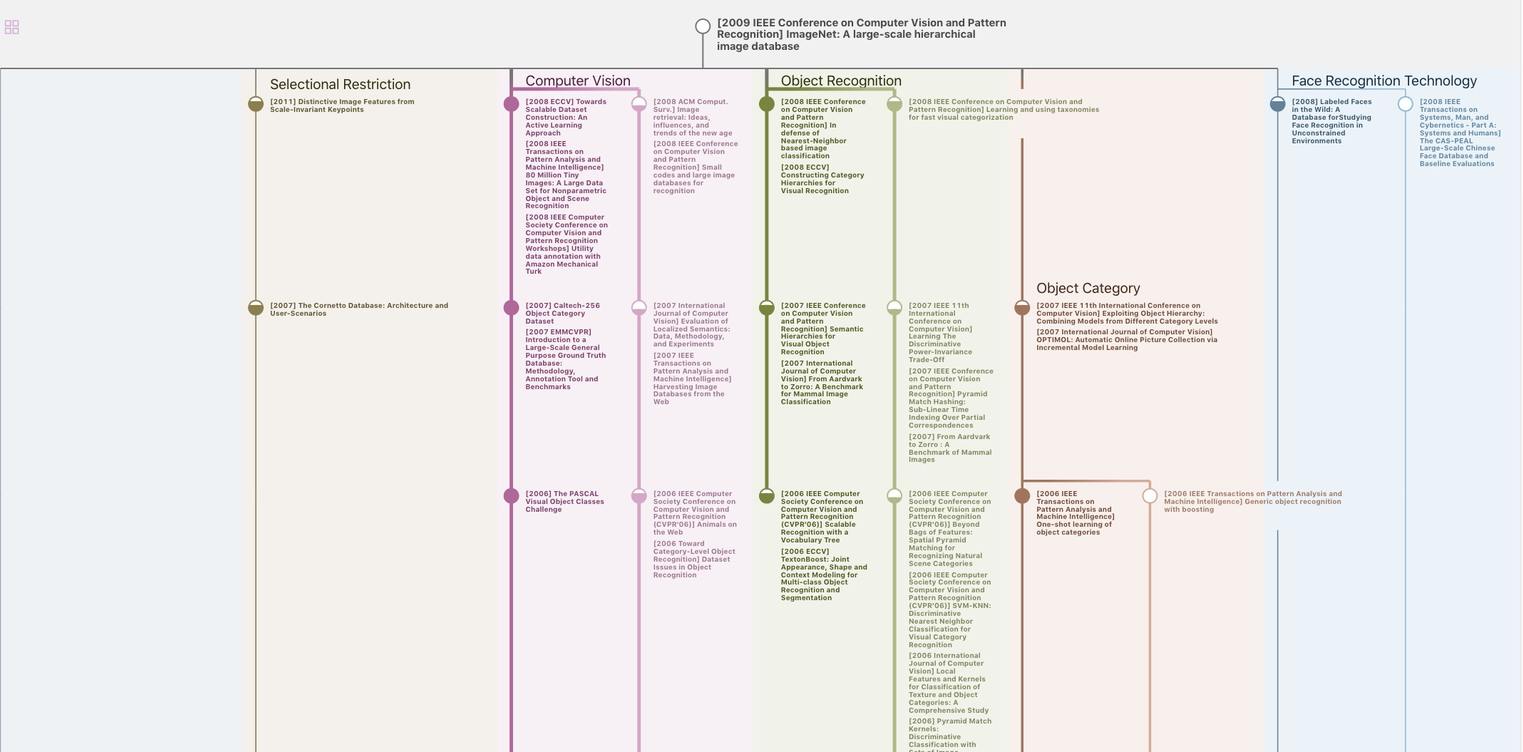
生成溯源树,研究论文发展脉络
Chat Paper
正在生成论文摘要