River Ice Regime Recognition Based on Deep Learning: Ice Concentration, Area, and Velocity
WATER(2024)
摘要
The real-time derivation of the concentration, area, and velocity of river surface ice based on camera imagery is essential for predicting the potential risks related to ice blockages in water routes. The key lies in the continuous tracking and velocity measuring of river ice, and reliable ice motion detection is a prerequisite for the dynamic perception of tracking targets. Previous studies did not utilize motion tracking for measuring ice velocity, and particle image velocimetry and feature point matching were used. This study aimed to use deep learning methods to address the challenging problems of deriving the ice concentration, area, and velocity based on camera imagery, and the focus was on measuring the ice velocity and drawing trajectories using the particle video tracking algorithm. We built a dataset named IPC_RI_IDS and collected information during the ice cover break-up process in the Nenjiang River (China). Our suggested approach was divided into four steps: (1) image preprocessing, where the camera image was calibrated to real-world coordinates; (2) determining the ice and water pixels in the camera image using the lightweight semantic segmentation network and then calculating the ice concentration and area; (3) enhancing and optimizing motion detection using the semantic segmentation results; and (4) adapting the particle video tracking algorithm to measure ice velocity using the proposed tracking points generation strategy. Finally, we analyzed the surface ice data in the study area and attempted to predict the stage of the ice break-up process to provide support for the real-time short-term forecasts of ice floods.
更多查看译文
关键词
river ice,ice regime,ice velocity,ice tracking,particle video,deep learning
AI 理解论文
溯源树
样例
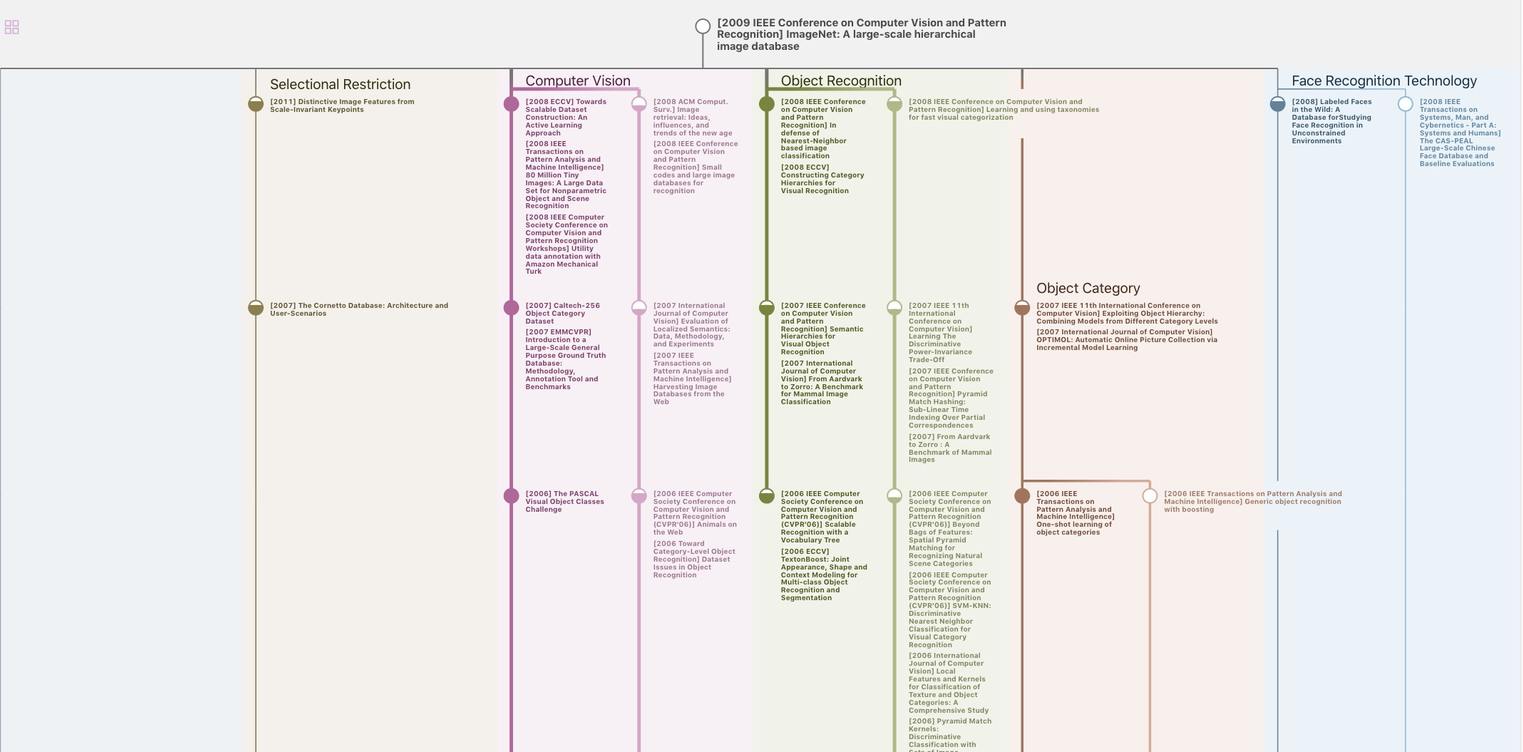
生成溯源树,研究论文发展脉络
Chat Paper
正在生成论文摘要