MSMB-GCN: Multi-scale Multi-branch Fusion Graph Convolutional Networks for 3D Human Pose Estimation.
2023 IEEE International Conference on Robotics and Biomimetics (ROBIO)(2023)
摘要
In human-robot interaction (HRI), human pose estimation is a necessary technology for the robot to perceive the dynamic environment and make interactive actions. Recently, graph convolutional networks (GCNs) have been increasingly used for 2D to 3D pose estimation tasks since the skeleton topologies can be viewed as graph structures. In this paper, we propose a novel graph convolutional network architecture, Multi-scale Multi-branch Fusion Graph Convolutional Networks (MSMB-GCN), for 3D Human Pose Estimation(3D HPE) task. The proposed model consists of multiple GCN blocks with a multi-branch architecture. This multi-branch architecture enables the model to get multi-scale features for human skeletal representations. The group of GCN blocks, which has strong multi-level feature extraction capabilities, allows the model to learn global and local features, lower-level and higher-level features. Experiment results on the HumanPose benchmark demonstrate that our model outperforms the state-of-the-art and ablation studies validate the effectiveness of our approach.
更多查看译文
AI 理解论文
溯源树
样例
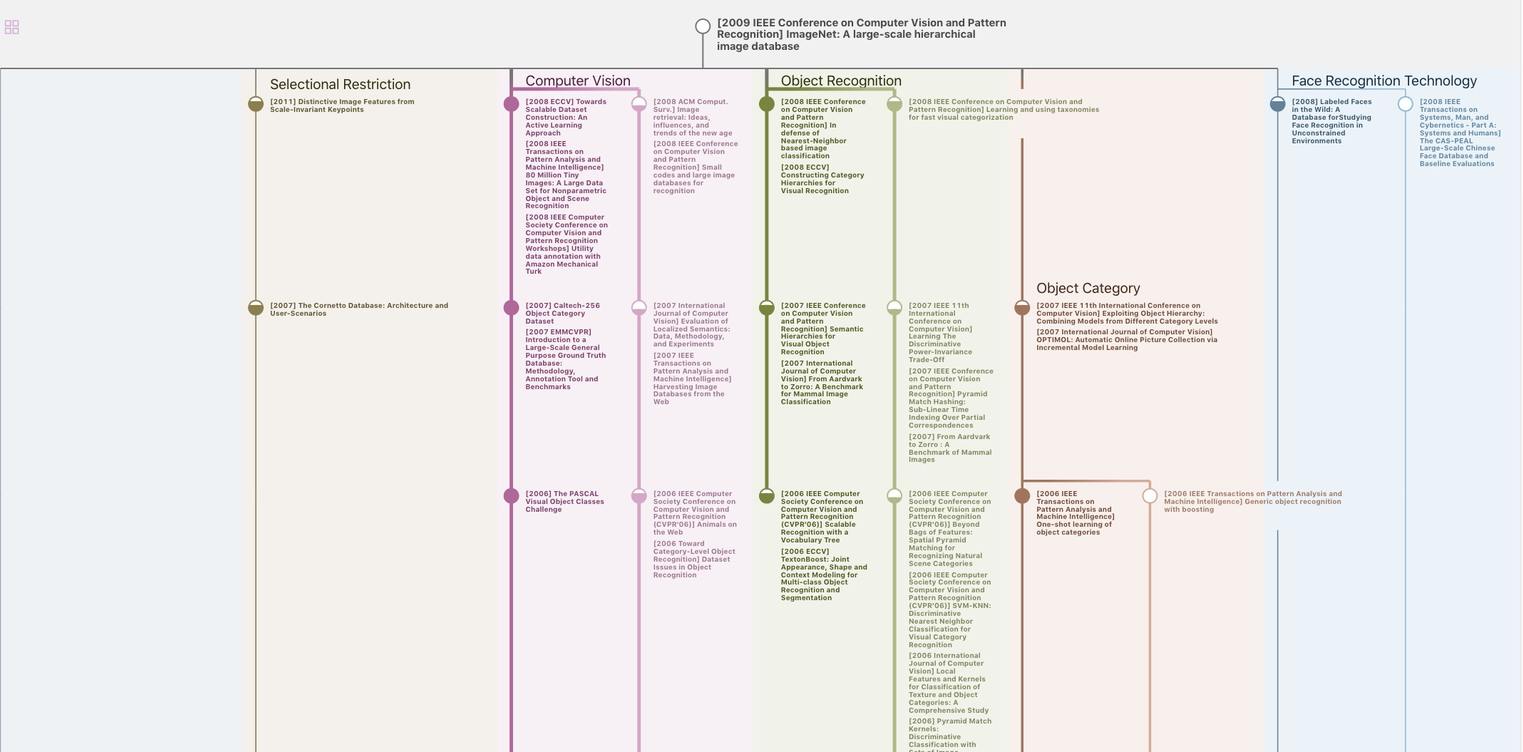
生成溯源树,研究论文发展脉络
Chat Paper
正在生成论文摘要