Adversarial data splitting for domain generalization
Science China Information Sciences(2024)
摘要
Domain generalization aims to learn a model that is generalizable to an unseen target domain, which is a fundamental and challenging task in machine learning for out-of-distribution generalization. This paper proposes a novel domain generalization approach that enforces the learned model to be able to generalize well over the train/val subset splitting of the training dataset. This idea is modeled herein as an adversarial data splitting framework, formulated as a min-max optimization problem inspired by the meta-learning approach. The min-max optimization problem is solved by iteratively splitting the training dataset into the training and val subsets to maximize the domain shift measured by the objective function and updating the model parameters to enable the model to generalize well from the training subset to the val subset by minimizing the objective function. This adversarial training approach does not assume the known domain labels of the training data; instead, it automatically investigates the “hard” splitting of the train/val subsets to learn the generalizable model. Extensive experimental results using three benchmark datasets demonstrate the superiority of this approach. In addition, we derive a generalization error bound for the theoretical understanding of our proposed approach.
更多查看译文
关键词
domain generalization,adversarial learning,data splitting,meta-learning,out of distribution
AI 理解论文
溯源树
样例
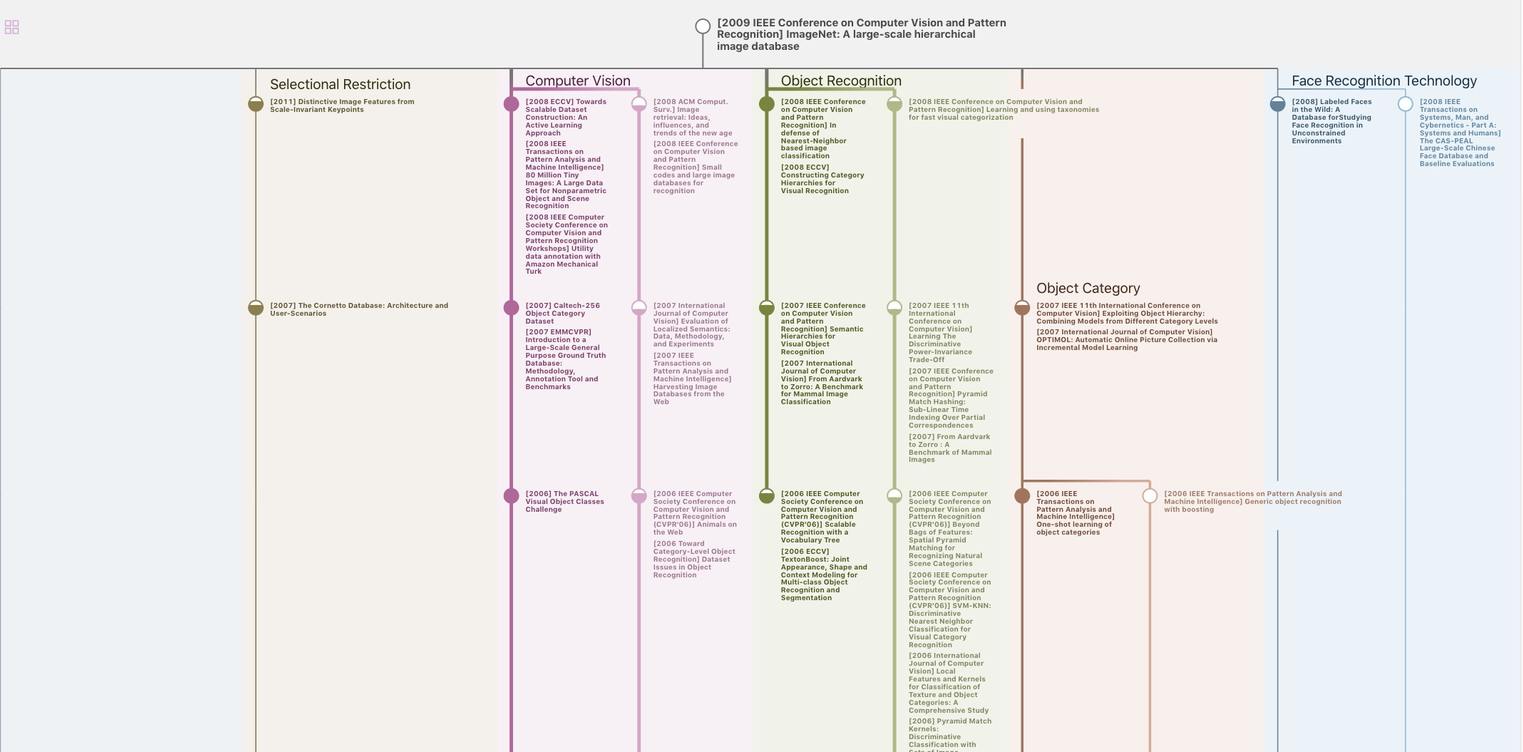
生成溯源树,研究论文发展脉络
Chat Paper
正在生成论文摘要