Application of the Improved K-Nearest Neighbor-Based Multi-Model Ensemble Method for Runoff Prediction
WATER(2024)
摘要
Hydrological forecasting plays a crucial role in mitigating flood risks and managing water resources. Data-driven hydrological models demonstrate exceptional fitting capabilities and adaptability. Recognizing the limitations of single-model forecasting, this study introduces an innovative approach known as the Improved K-Nearest Neighbor Multi-Model Ensemble (IKNN-MME) method to enhance the runoff prediction. IKNN-MME dynamically adjusts model weights based on the similarity of historical data, acknowledging the influence of different training data features on localized predictions. By combining an enhanced K-Nearest Neighbor (KNN) algorithm with adaptive weighting, it offers a more powerful and flexible ensemble. This study evaluates the performance of the IKNN-MME method across four basins in the United States and compares it to other multi-model ensemble methods and benchmark models. The results underscore its outstanding performance and adaptability, offering a promising avenue for improving runoff forecasting.
更多查看译文
关键词
runoff prediction,data-driven hydrological models,multi-model ensemble,IKNN-MME
AI 理解论文
溯源树
样例
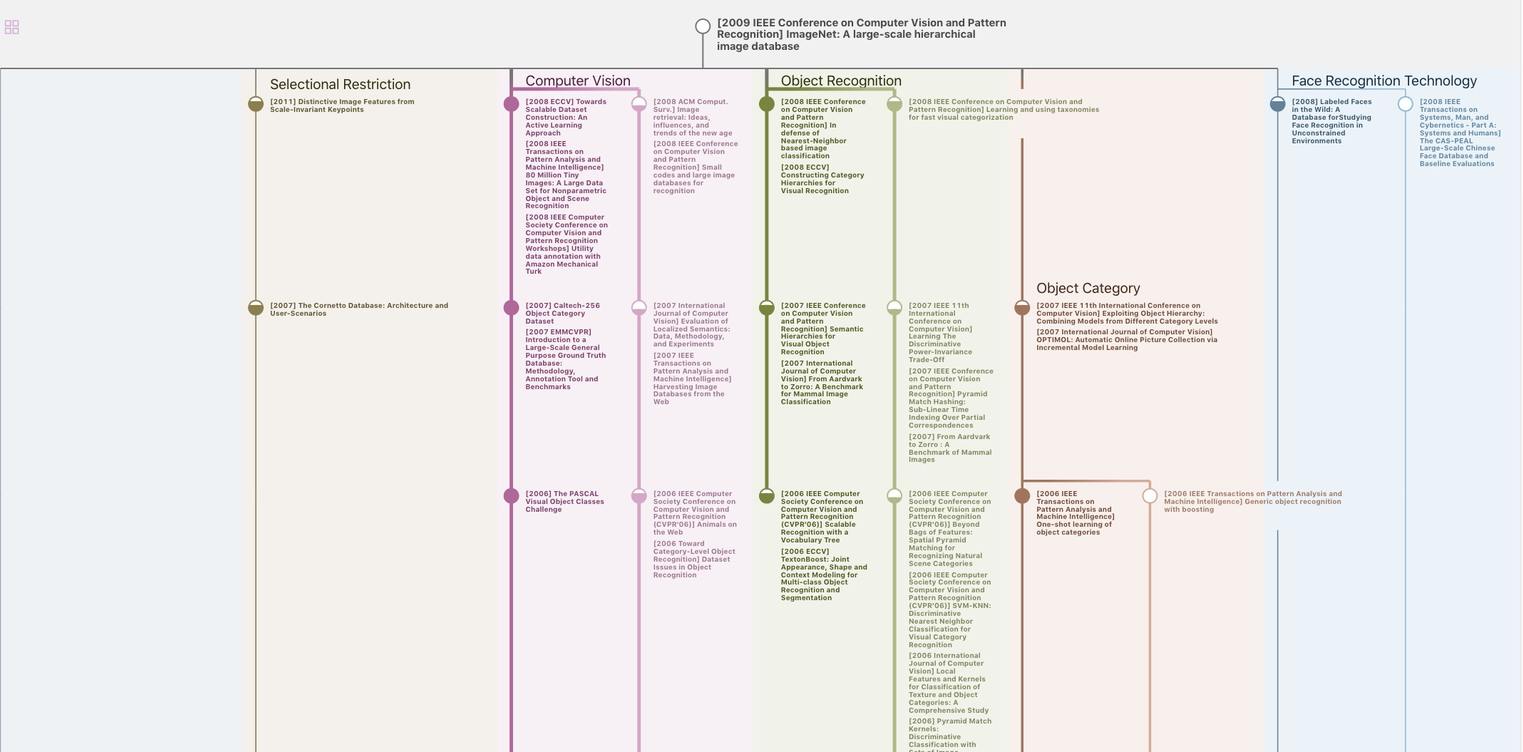
生成溯源树,研究论文发展脉络
Chat Paper
正在生成论文摘要