Multimodal Attention Merging for Improved Speech Recognition and Audio Event Classification
CoRR(2023)
摘要
Training large foundation models using self-supervised objectives on
unlabeled data, followed by fine-tuning on downstream tasks, has emerged as a
standard procedure. Unfortunately, the efficacy of this approach is often
constrained by both limited fine-tuning compute and scarcity in labeled
downstream data. We introduce Multimodal Attention Merging (MAM), an attempt
that facilitates direct knowledge transfer from attention matrices of models
rooted in high resource modalities, text and images, to those in
resource-constrained domains, speech and audio, employing a zero-shot paradigm.
MAM reduces the relative Word Error Rate (WER) of an Automatic Speech
Recognition (ASR) model by up to 6.70
Audio Event Classification (AEC) model by 10.63
data/compute is available, we present Learnable-MAM, a data-driven approach to
merging attention matrices, resulting in a further 2.90
WER for ASR and 18.42
更多查看译文
关键词
Knowledge transfer,cross-modal adaptation,speech recognition,and acoustic modeling
AI 理解论文
溯源树
样例
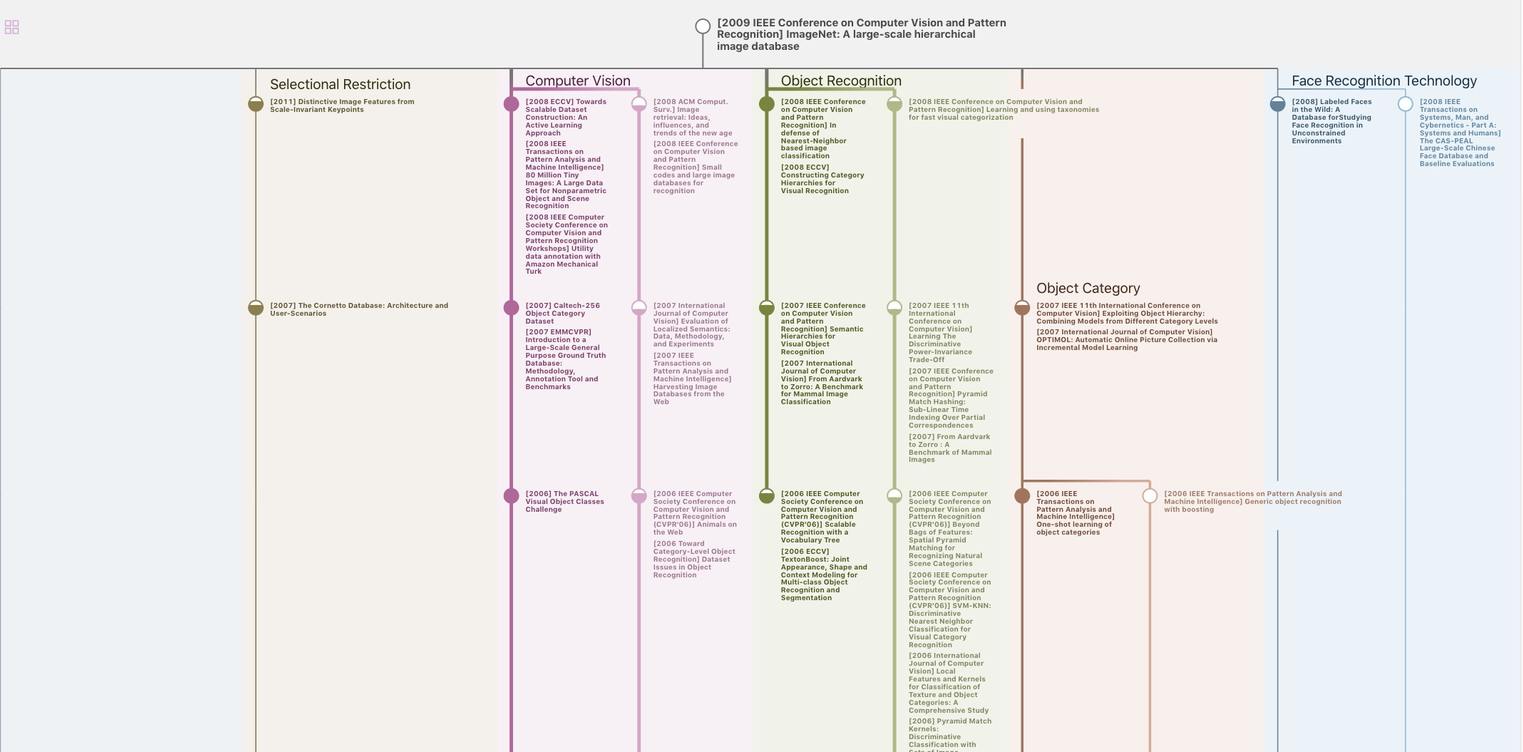
生成溯源树,研究论文发展脉络
Chat Paper
正在生成论文摘要