Harnessing the Final Control Error for Optimal Data-Driven Predictive Control
CoRR(2023)
摘要
Model Predictive Control (MPC) is a powerful method for complex system
regulation, but its reliance on accurate models poses many limitations in
real-world applications. Data-driven predictive control (DDPC) offers a valid
alternative, eliminating the need for model identification. However, it may
falter in the presence of noisy data. In response, in this work, we present a
unified stochastic framework for direct DDPC where control actions are obtained
by optimizing the Final Control Error, directly computed from available data
only, that automatically weighs the impact of uncertainty on the control
objective. Our approach generalizes existing DDPC methods, like regularized
Data-enabled Predictive Control (DeePC) and $\gamma$-DDPC, and thus provides a
path toward noise-tolerant data-based control, with rigorous optimality
guarantees. The theoretical investigation is complemented by a series of
numerical case studies, revealing that the proposed method consistently
outperforms or, at worst, matches existing techniques without requiring tuning
regularization parameters as methods do.
更多查看译文
AI 理解论文
溯源树
样例
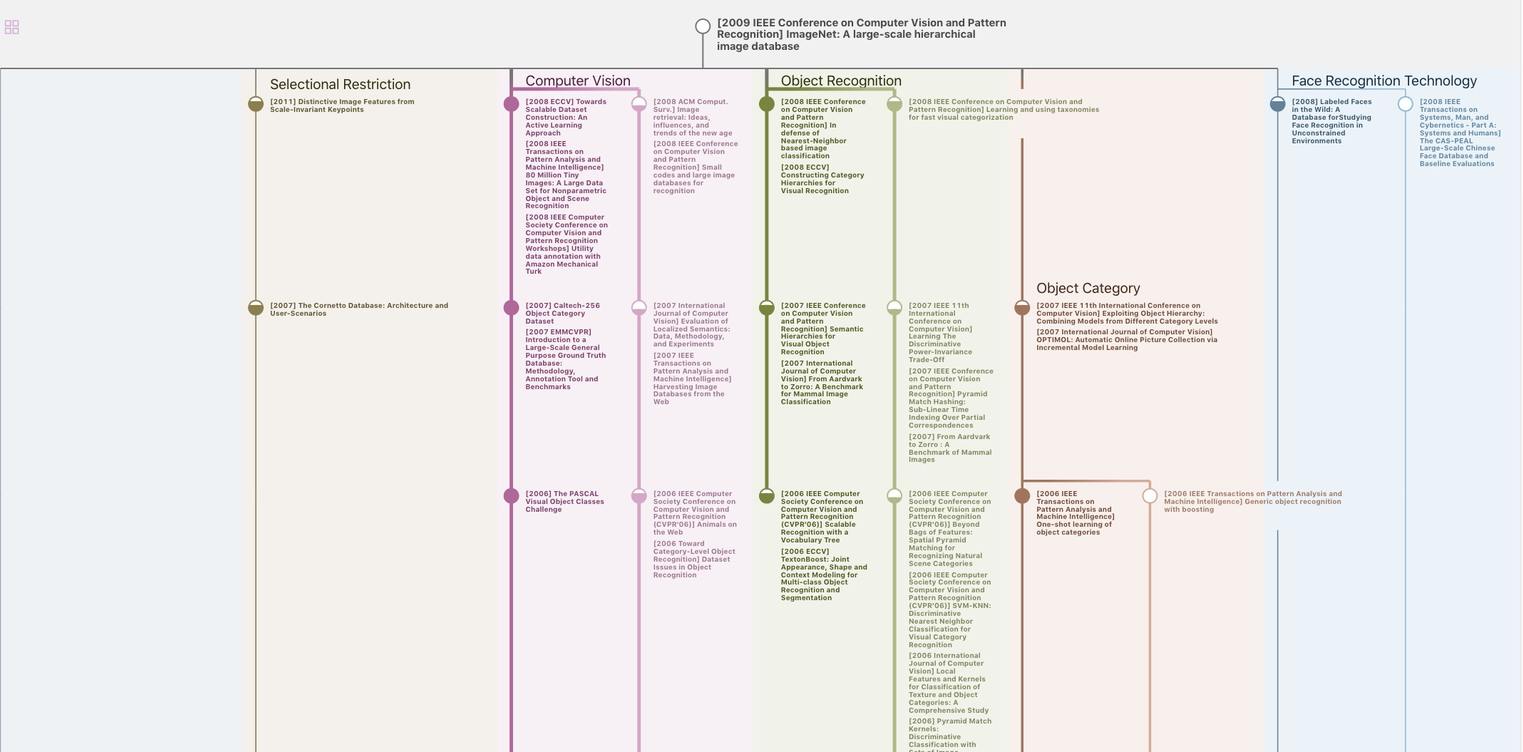
生成溯源树,研究论文发展脉络
Chat Paper
正在生成论文摘要