Deep Learning Approaches for Quantifying Ventilation Defects in Hyperpolarized Gas Magnetic Resonance Imaging of the Lung: A Review
BIOENGINEERING-BASEL(2023)
Abstract
This paper provides an in-depth overview of Deep Neural Networks and their application in the segmentation and analysis of lung Magnetic Resonance Imaging (MRI) scans, specifically focusing on hyperpolarized gas MRI and the quantification of lung ventilation defects. An in-depth understanding of Deep Neural Networks is presented, laying the groundwork for the exploration of their use in hyperpolarized gas MRI and the quantification of lung ventilation defects. Five distinct studies are examined, each leveraging unique deep learning architectures and data augmentation techniques to optimize model performance. These studies encompass a range of approaches, including the use of 3D Convolutional Neural Networks, cascaded U-Net models, Generative Adversarial Networks, and nnU-net for hyperpolarized gas MRI segmentation. The findings highlight the potential of deep learning methods in the segmentation and analysis of lung MRI scans, emphasizing the need for consensus on lung ventilation segmentation methods.
MoreTranslated text
Key words
deep learning,Magnetic Resonance Imaging (MRI),hyperpolarized gas MRI,segmentation,ventilation defect,chronic obstructive pulmonary disease (COPD),lung imaging
AI Read Science
Must-Reading Tree
Example
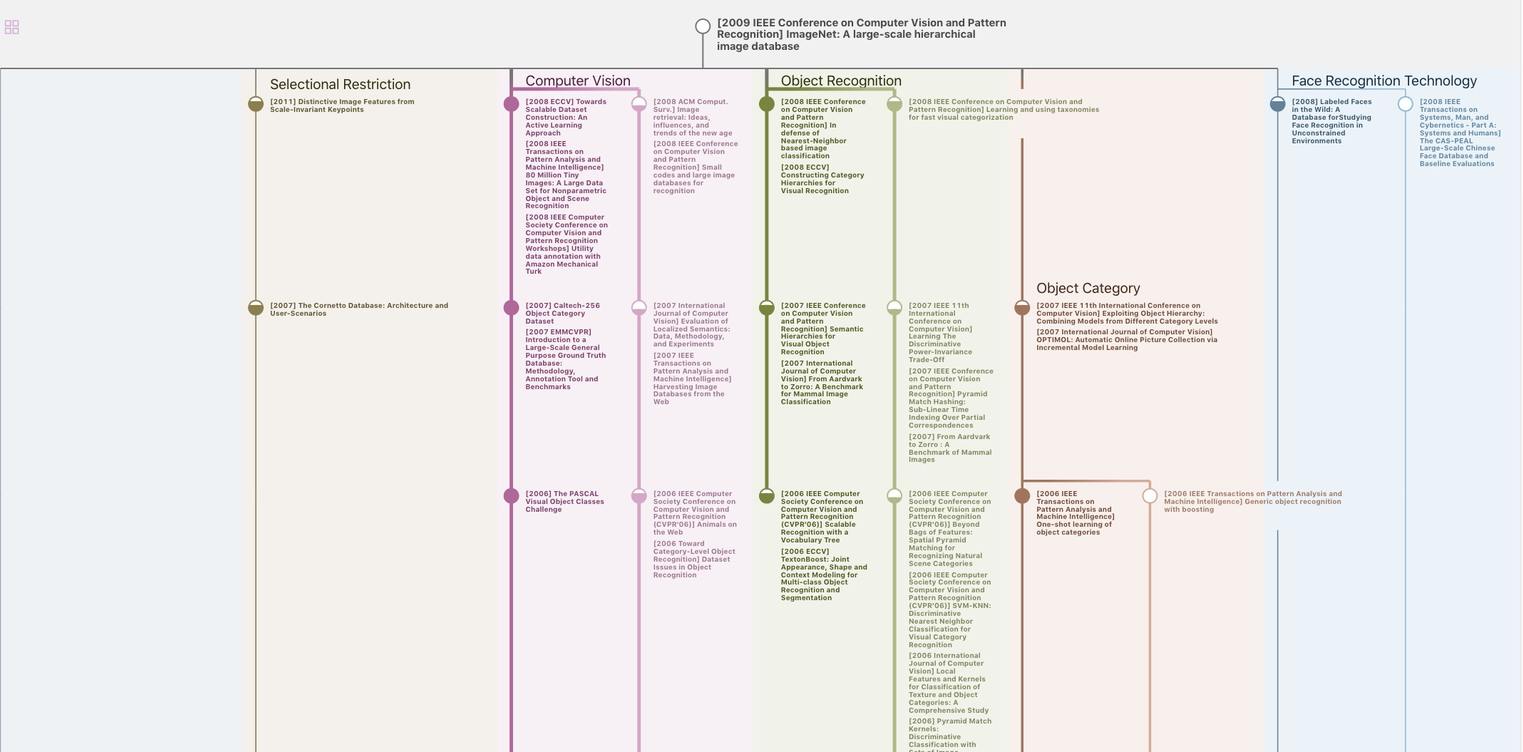
Generate MRT to find the research sequence of this paper
Chat Paper
Summary is being generated by the instructions you defined