Elucidating Key Characteristics of PFAS Binding to Human Peroxisome Proliferator-Activated Receptor Alpha: An Explainable Machine Learning Approach
ENVIRONMENTAL SCIENCE & TECHNOLOGY(2023)
摘要
Per- and polyfluoroalkyl substances (PFAS) are widely employed anthropogenic fluorinated chemicals known to disrupt hepatic lipid metabolism by binding to human peroxisome proliferator-activated receptor alpha (PPAR alpha). Therefore, screening for PFAS that bind to PPAR alpha is of critical importance. Machine learning approaches are promising techniques for rapid screening of PFAS. However, traditional machine learning approaches lack interpretability, posing challenges in investigating the relationship between molecular descriptors and PPAR alpha binding. In this study, we aimed to develop a novel, explainable machine learning approach to rapidly screen for PFAS that bind to PPAR alpha. We calculated the PPAR alpha-PFAS binding score and 206 molecular descriptors for PFAS. Through systematic and objective selection of important molecular descriptors, we developed a machine learning model with good predictive performance using only three descriptors. The molecular size (b_single) and electrostatic properties (BCUT_PEOE_3 and PEOE_VSA_PPOS) are important for PPAR alpha-PFAS binding. Alternative PFAS are considered safer than their legacy predecessors. However, we found that alternative PFAS with many carbon atoms and ether groups exhibited a higher affinity for PPAR alpha. Therefore, confirming the toxicity of these alternative PFAS compounds with such characteristics through biological experiments is important.
更多查看译文
关键词
PFAS,PPAR alpha,machine learning,SHAP,QSAR,molecular descriptor
AI 理解论文
溯源树
样例
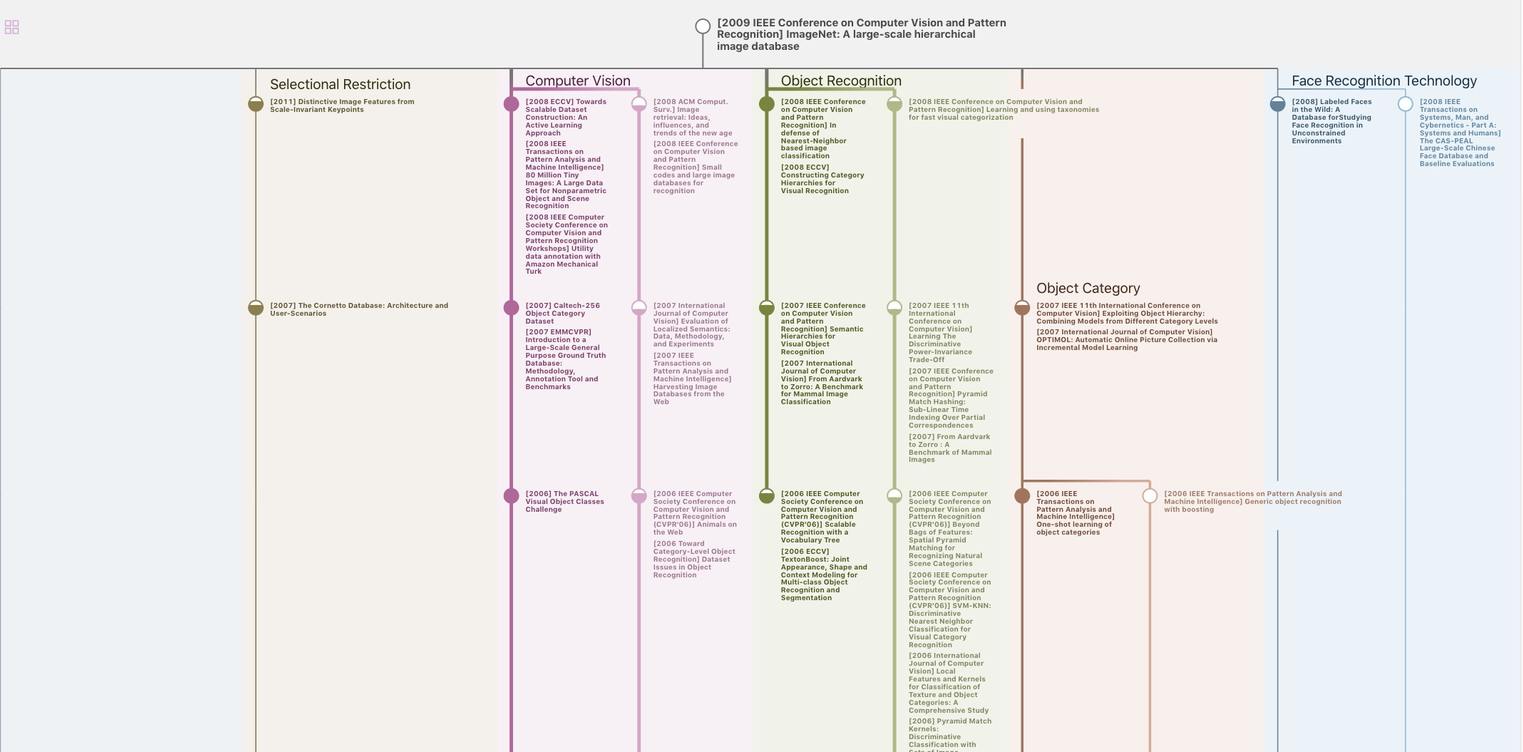
生成溯源树,研究论文发展脉络
Chat Paper
正在生成论文摘要