Deep Reinforcement Learning for Power Control in Secure Broadcast Channels.
2023 21st International Symposium on Modeling and Optimization in Mobile, Ad Hoc, and Wireless Networks (WiOpt)(2023)
摘要
In this work, we investigate the dynamic power management problem in a two-user secure broadcast channel scenario where the information at the source is kept in two separate queues, one for confidential and one for non-confidential information. The intended receiver of the confidential information transmits a jamming signal to prevent the other receiver from successfully decoding the confidential information. The decoding strategies used at the receivers include successive decoding (SD) and treating interference as noise (TIN). An autonomous agent, located at the source, decides on the power that will be used for the transmission of confidential information. We formulate the transmission power decision problem as a Markov Decision Process (MDP) and utilize the Deep Deterministic Policy Gradient (DDPG) algorithm for the agent to learn approximately optimal policies. We demonstrate that SD yields better rewards than TIN at the legitimate receiver. Additionally, we contrast the DDPG algorithm with three baseline power control policies and show that the former performs better than the latter.
更多查看译文
AI 理解论文
溯源树
样例
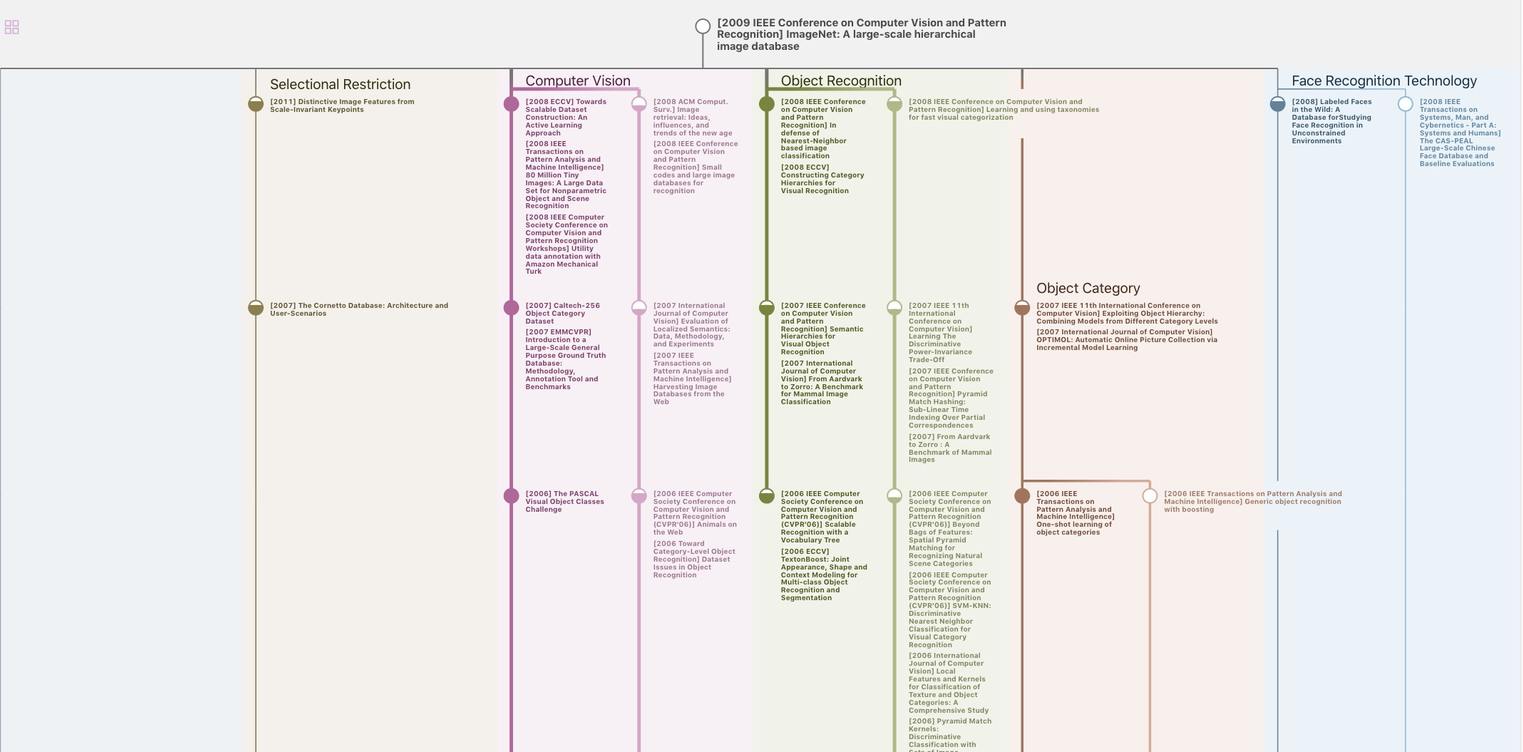
生成溯源树,研究论文发展脉络
Chat Paper
正在生成论文摘要