Automated Optical Accelerator Search Toward Superior Acceleration Efficiency, Inference Robustness, and Development Speed
IEEE TRANSACTIONS ON COMPUTER-AIDED DESIGN OF INTEGRATED CIRCUITS AND SYSTEMS(2024)
摘要
Remarkable breakthroughs but daunting complexities of deep learning have aroused widespread interest in dedicated deep neural network (DNN) acceleration hardware, among which optical accelerators (OAs) are particularly promising thanks to their unprecedentedly high-performance-per-watt. However, the development of OAs is much slower than that of electrical accelerators due to threefold challenges. First, the OA design space is ample and discrete, making it tough for OA optimization; Second, the ecosystem that facilitates OA development is still in its infancy. Techniques to support OA design remain less explored, limiting both the achievable performance and the innovative development of OAs; and Third, OAs are highly sensitive to fabrication-induced process variations and thermal fluctuations (i.e., PTVs), which degrades OAs' inference robustness and even renders them unusable in practice. In this article, we develop AutOAS, the first-of-its-kind framework for Automated Optical Accelerator Search, in order to jointly boost acceleration efficiency, inference robustness, and development speed. Our AutOAS comprises four enabling components: 1) a holistic OA search space, which takes full consideration of OAs' micro-architectures (e.g., the type, shape and size of core functional units for data computation and data access), dataflow choices, DNN-to-accelerator mapping methods, memory hierarchy and PTV mitigation techniques; 2) a PTV Regulator, which can emulate the impact of PTVs on OAs' inference accuracy based on given PTV profiles, and enables energy-efficient PTV mitigation on OAs; 3) an O-Performance Predictor, which enables accurate yet efficient predictions of an OA's energy, throughput (latency) and chip area according to the DNN model and OA architecture parameters; and 4) two O-Search Engines (i.e., a differentiable search engine and an evolutionary search engine), which can automatically explore the large design space of OAs and identify the optimal accelerators to maximize the acceleration targets. Based on 10 DNN models widely applied in both computer vision and sequence modeling tasks, extensive experiments and ablation studies validate the effectiveness of our PTV Regulator, O-Performance Predictor, and O-Search Engines, as well as the superior performance of AutOAS-generated OAs.
更多查看译文
关键词
Optical waveguides,Engines,Robustness,Computer architecture,Computational modeling,Artificial neural networks,Regulators,Acceleration efficiency,automated search,development tools,inference robustness,optical deep neural network (DNN) accelerator
AI 理解论文
溯源树
样例
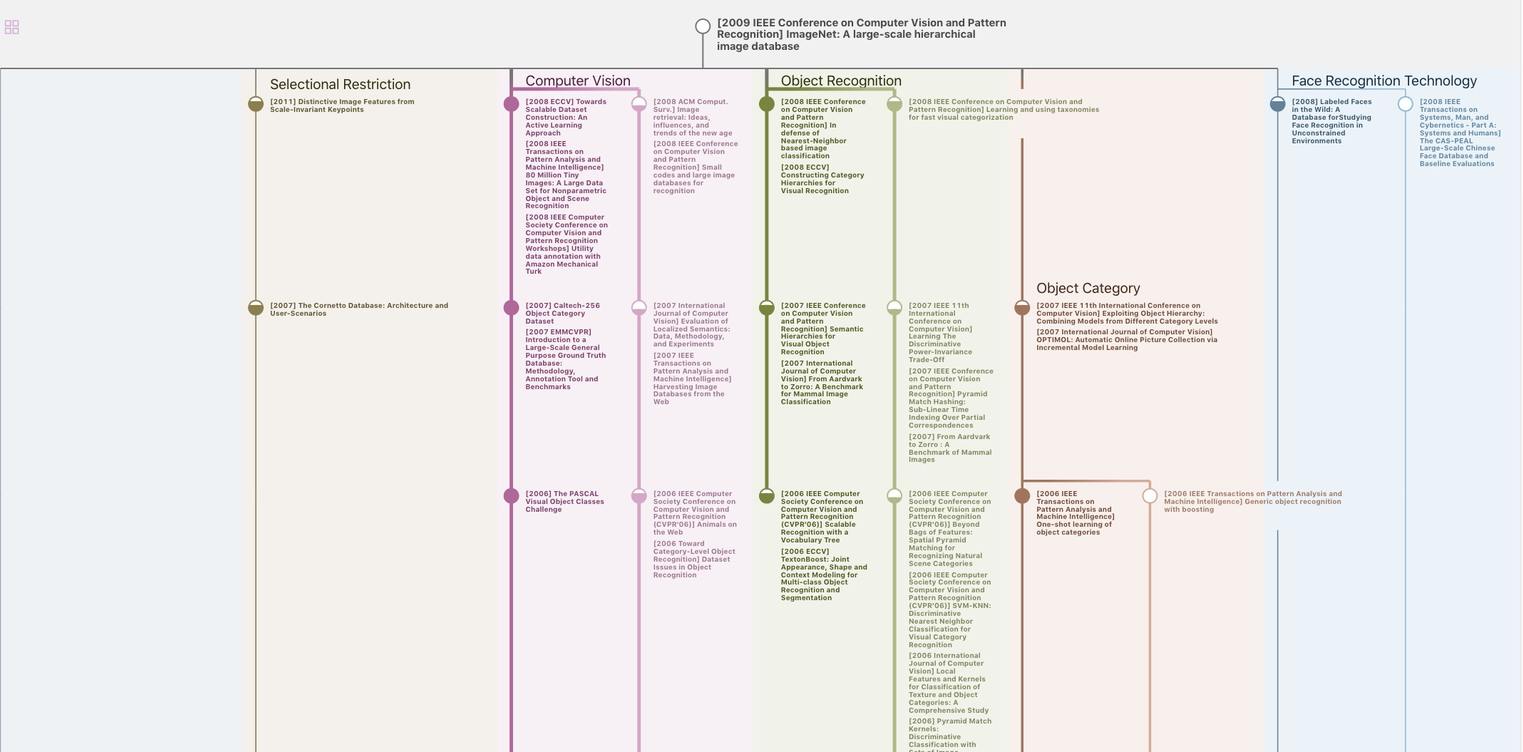
生成溯源树,研究论文发展脉络
Chat Paper
正在生成论文摘要